Learning 3D medical image keypoint descriptors with the triplet loss
INTERNATIONAL JOURNAL OF COMPUTER ASSISTED RADIOLOGY AND SURGERY(2021)
摘要
Purpose We propose to learn a 3D keypoint descriptor which we use to match keypoints extracted from full-body CT scans. Our methods are inspired by 2D keypoint descriptor learning, which was shown to outperform hand-crafted descriptors. Adapting these to 3D images is challenging because of the lack of labelled training data and high memory requirements. Method We generate semi-synthetic training data. For that, we first estimate the distribution of local affine inter-subject transformations using labelled anatomical landmarks on a small subset of the database. We then sample a large number of transformations and warp unlabelled CT scans, for which we can subsequently establish reliable keypoint correspondences using guided matching. These correspondences serve as training data for our descriptor, which we represent by a CNN and train using the triplet loss with online triplet mining. Results We carry out experiments on a synthetic data reliability benchmark and a registration task involving 20 CT volumes with anatomical landmarks used for evaluation purposes. Our learned descriptor outperforms the 3D-SURF descriptor on both benchmarks while having a similar runtime. Conclusion We propose a new method to generate semi-synthetic data and a new learned 3D keypoint descriptor. Experiments show improvement compared to a hand-crafted descriptor. This is promising as literature has shown that jointly learning a detector and a descriptor gives further performance boost.
更多查看译文
关键词
Medical imaging, Keypoints, Descriptors, Convolution neural network, Triplet loss
AI 理解论文
溯源树
样例
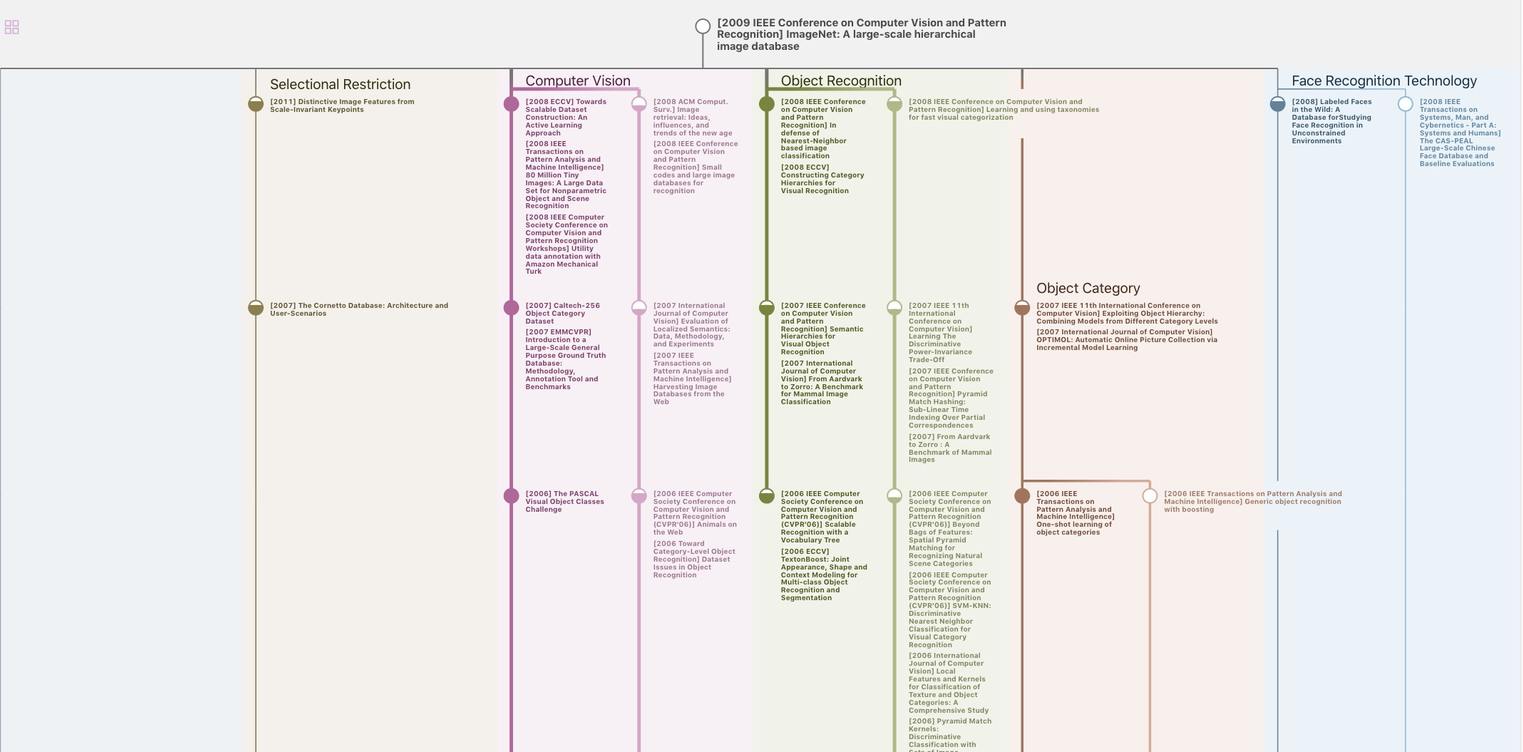
生成溯源树,研究论文发展脉络
Chat Paper
正在生成论文摘要