Deep learning radiomic nomogram to predict recurrence in soft tissue sarcoma: a multi-institutional study
EUROPEAN RADIOLOGY(2021)
摘要
Objectives To evaluate the performance of a deep learning radiomic nomogram (DLRN) model at predicting tumor relapse in patients with soft tissue sarcomas (STS) who underwent surgical resection. Methods In total, 282 patients who underwent MRI and resection for STS at three independent centers were retrospectively enrolled. In addition, 113 of the 282 patients received additional contrast-enhanced MRI scans. We separated the participants into a development cohort and an external test cohort. The development cohort consisted of patients from one center and the external test cohort consisted of patients from two other centers. Two MRI-based DLRNs for prediction of tumor relapse after resection of STS were established. We universally tested the DLRNs and compared them with other prediction models constructed by using widespread adopted predictors (i.e., staging systems and Ki67) instead of radiomics features. Results The DLRN1 model incorporated plain MRI-based radiomics signature into the clinical data, and the DLRN2 model integrated radiomics signature extracted from plain and contrast-enhanced MRI with the clinical predictors. Across both study sets, the two MRI-based DLRNs had relatively better prognostic capability ( C index ≥ 0.721 and median AUC ≥ 0.746; p < 0.05 compared with most other models and predictors) and less opportunity for prediction error (integrated Brier score ≤ 0.159). The decision curve analysis indicates that the DLRNs have greater benefits than staging systems, Ki67, and other models. We selected appropriate cutoff values for the DLRNs to divide STS recurrence into three risk strata (low, medium, and high) and calculated those groups’ cumulative risk rates. Conclusion The DLRNs were shown to be a reliable and externally validated tool for predicting STS recurrence by comparing with other prediction models. Key Points • The prediction of a high recurrence rate of STS before emergence of local recurrence can help to determine whether more active treatment should be implemented. • Two MRI-based DLRNs for prediction of tumor relapse were shown to be a reliable and externally validated tool for predicting STS recurrence. • We used the DLRNs to divide STS recurrence into three risk strata (low, medium, and high) to facilitate more targeted postoperative management in the clinic.
更多查看译文
关键词
Magnetic resonance imaging, Deep learning, Radiomic nomogram, Soft tissue sarcomas, Recurrence
AI 理解论文
溯源树
样例
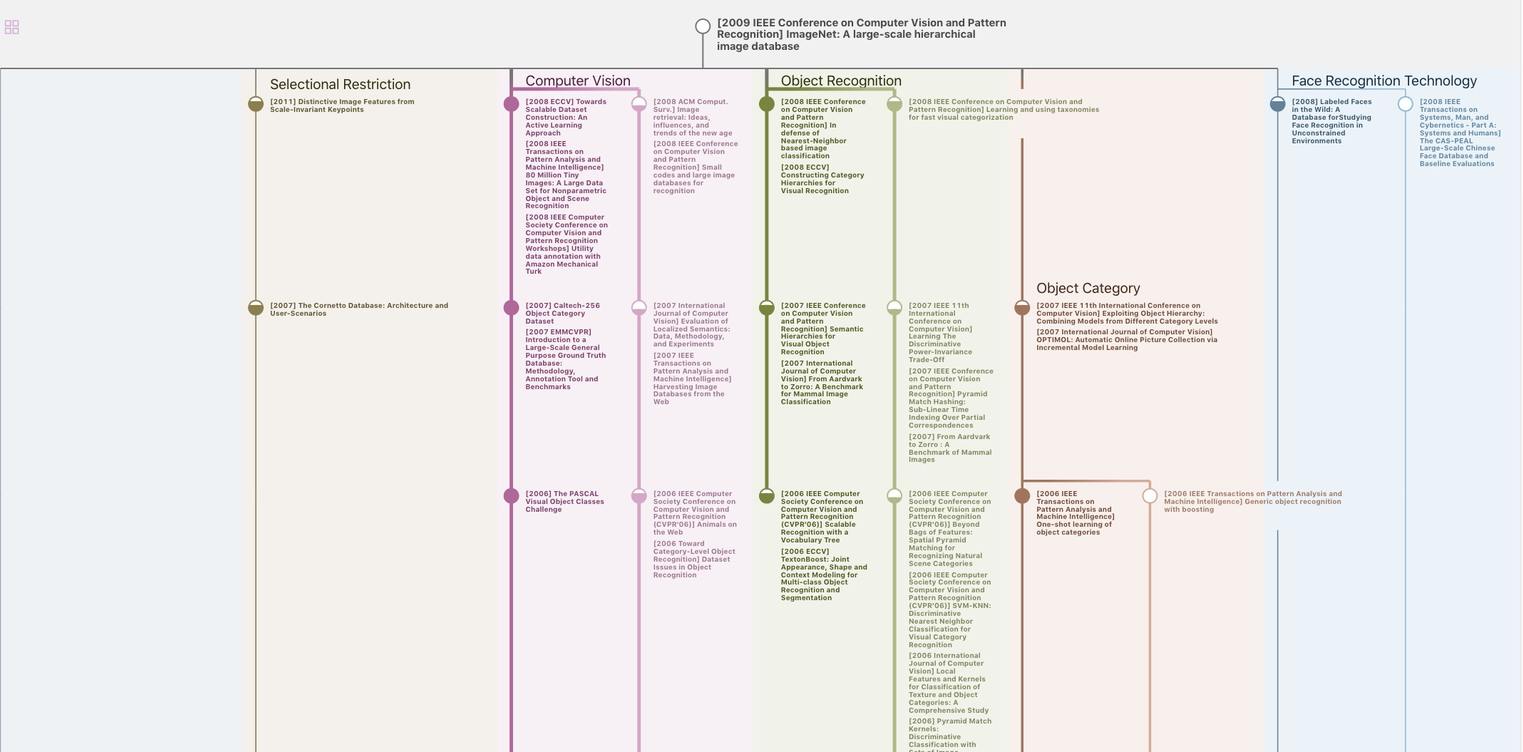
生成溯源树,研究论文发展脉络
Chat Paper
正在生成论文摘要