Flexible And Efficient Inference With Particles For The Variational Gaussian Approximation
ENTROPY(2021)
摘要
Variational inference is a powerful framework, used to approximate intractable posteriors through variational distributions. The de facto standard is to rely on Gaussian variational families, which come with numerous advantages: they are easy to sample from, simple to parametrize, and many expectations are known in closed-form or readily computed by quadrature. In this paper, we view the Gaussian variational approximation problem through the lens of gradient flows. We introduce a flexible and efficient algorithm based on a linear flow leading to a particle-based approximation. We prove that, with a sufficient number of particles, our algorithm converges linearly to the exact solution for Gaussian targets, and a low-rank approximation otherwise. In addition to the theoretical analysis, we show, on a set of synthetic and real-world high-dimensional problems, that our algorithm outperforms existing methods with Gaussian targets while performing on a par with non-Gaussian targets.
更多查看译文
关键词
variational inference, Gaussian, particle flow, variable flow
AI 理解论文
溯源树
样例
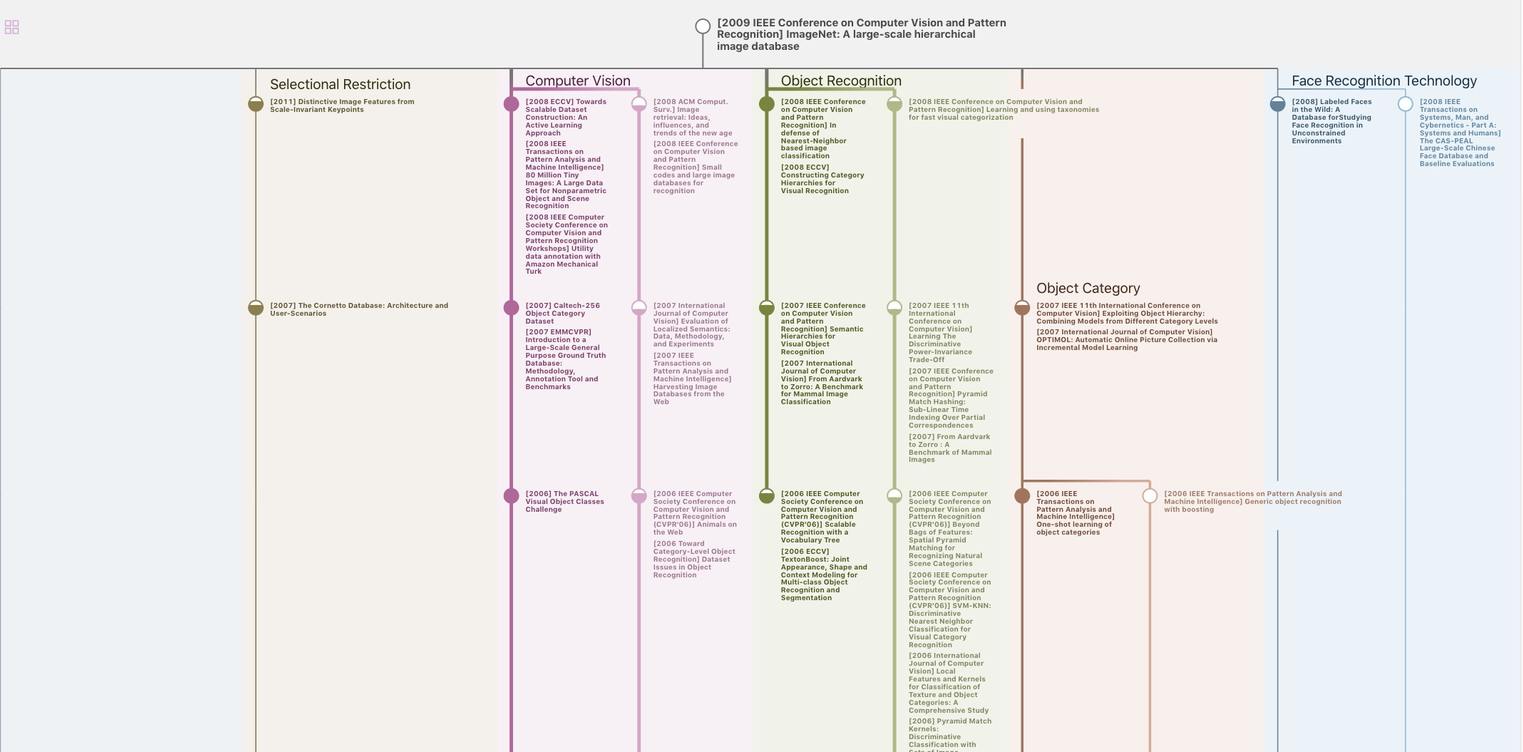
生成溯源树,研究论文发展脉络
Chat Paper
正在生成论文摘要