Sample size re-estimation in clinical trials
STATISTICS IN MEDICINE(2021)
摘要
In clinical trials, sample size re-estimation is often conducted at interim. The purpose is to determine whether the study will achieve study objectives if the observed treatment effect at interim preserves till end of the study. A traditional approach is to conduct a conditional power analysis for sample size only based on observed treatment effect. This approach, however, does not take into consideration the variabilities of (i) the observed (estimate) treatment effect and (ii) the observed (estimate) variability associated with the treatment effect. Thus, the resultant re-estimated sample sizes may not be robust and hence may not be reliable. In this article, a couple of methods are proposed, namely, adjusted effect size (AES) approach and iterated expectation/variance (IEV) approach, which can account for the variability associated with the observed responses at interim. The proposed methods provide interval estimates of sample size required for the intended trial, which is useful for making critical go/no go decision. Statistical properties of the proposed methods are evaluated in terms of controlling of type I error rate and statistical power. The results show that traditional approach performs poorly in controlling type I error inflation, whereas IEV approach has the best performance in most cases. Additionally, all re-estimation approaches can keep the statistical power over 80%; especially, IEV approach's statistical power, using adjusted significance level, is over 95%. However, IEV approach may lead to a greater increment in sample size when detecting a smaller effect size. In general, IEV approach is effective when effect size is large; otherwise, AES approach is more suitable for controlling type I error rate and keep power over 80% with a more reasonable re-estimated sample size.
更多查看译文
关键词
adjusted effect size approach, adjusted statistical power, control of type I error rate, iterated expectation, variance approach
AI 理解论文
溯源树
样例
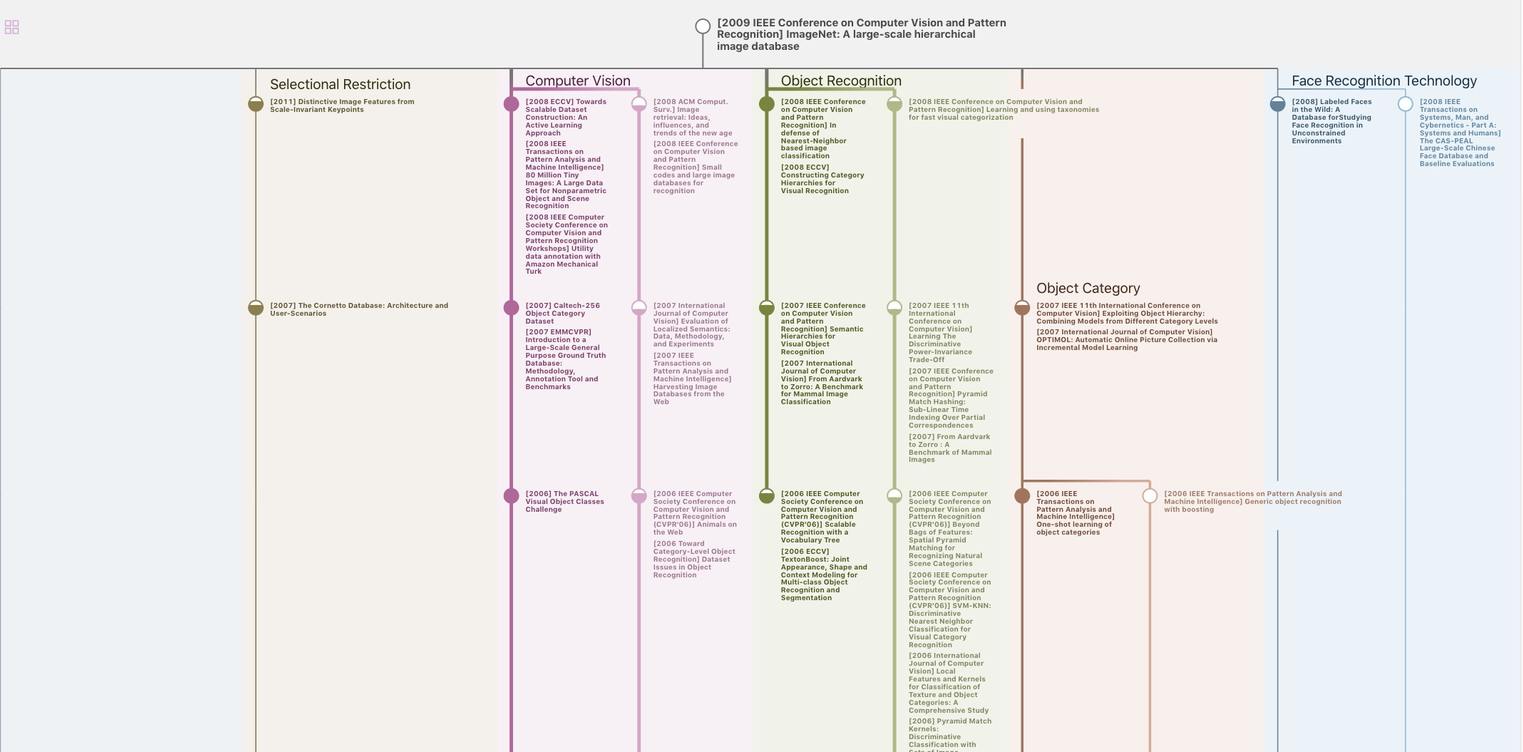
生成溯源树,研究论文发展脉络
Chat Paper
正在生成论文摘要