Sketches for Time-Dependent Machine Learning
arxiv(2021)
摘要
Time series data can be subject to changes in the underlying process that generates them and, because of these changes, models built on old samples can become obsolete or perform poorly. In this work, we present a way to incorporate information about the current data distribution and its evolution across time into machine learning algorithms. Our solution is based on efficiently maintaining statistics, particularly the mean and the variance, of data features at different time resolutions. These data summarisations can be performed over the input attributes, in which case they can then be fed into the model as additional input features, or over latent representations learned by models, such as those of Recurrent Neural Networks. In classification tasks, the proposed techniques can significantly outperform the prediction capabilities of equivalent architectures with no feature / latent summarisations. Furthermore, these modifications do not introduce notable computational and memory overhead when properly adjusted.
更多查看译文
关键词
machine learning,time-dependent
AI 理解论文
溯源树
样例
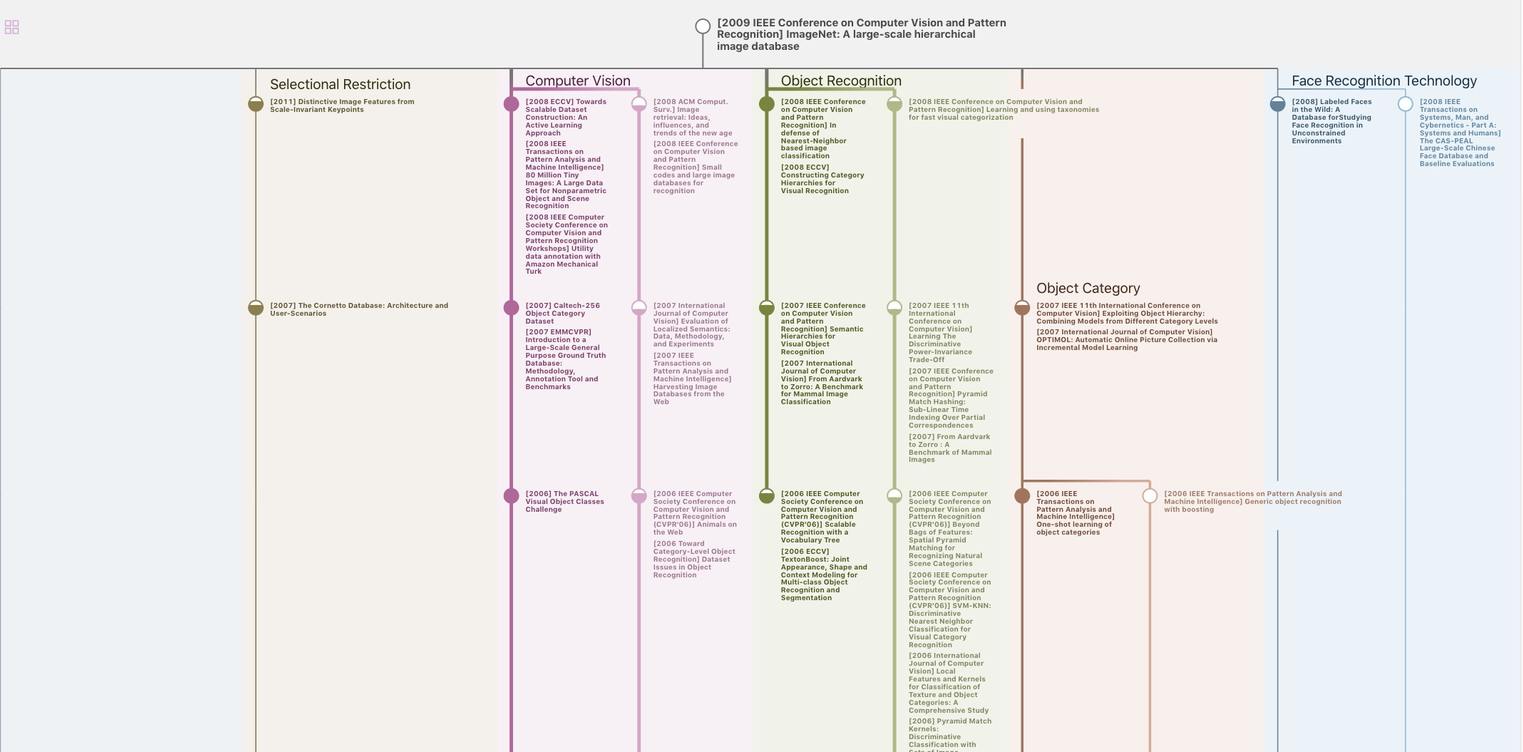
生成溯源树,研究论文发展脉络
Chat Paper
正在生成论文摘要