Efficient On-Chip Communication for Parallel Graph-Analytics on Spatial Architectures
arxiv(2022)
摘要
Large-scale graph processing has drawn great attention in recent years. Most of the modern-day datacenter workloads can be represented in the form of Graph Processing such as MapReduce etc. Consequently, a lot of designs for Domain-Specific Accelerators have been proposed for Graph Processing. Spatial Architectures have been promising in the execution of Graph Processing, where the graph is partitioned into several nodes and each node works in parallel. We conduct experiments to analyze the on-chip movement of data in graph processing on a Spatial Architecture. Based on the observations, we identify a data movement bottleneck, in the execution of such highly parallel processing accelerators. To mitigate the bottleneck we propose a novel power-law aware Graph Partitioning and Data Mapping scheme to reduce the communication latency by minimizing the hop counts on a scalable network-on-chip. The experimental results on popular graph algorithms show that our implementation makes the execution 2-5x faster and 2.7-4x energy-efficient by reducing the data movement time in comparison to a baseline implementation.
更多查看译文
关键词
parallel,on-chip,graph-analytics
AI 理解论文
溯源树
样例
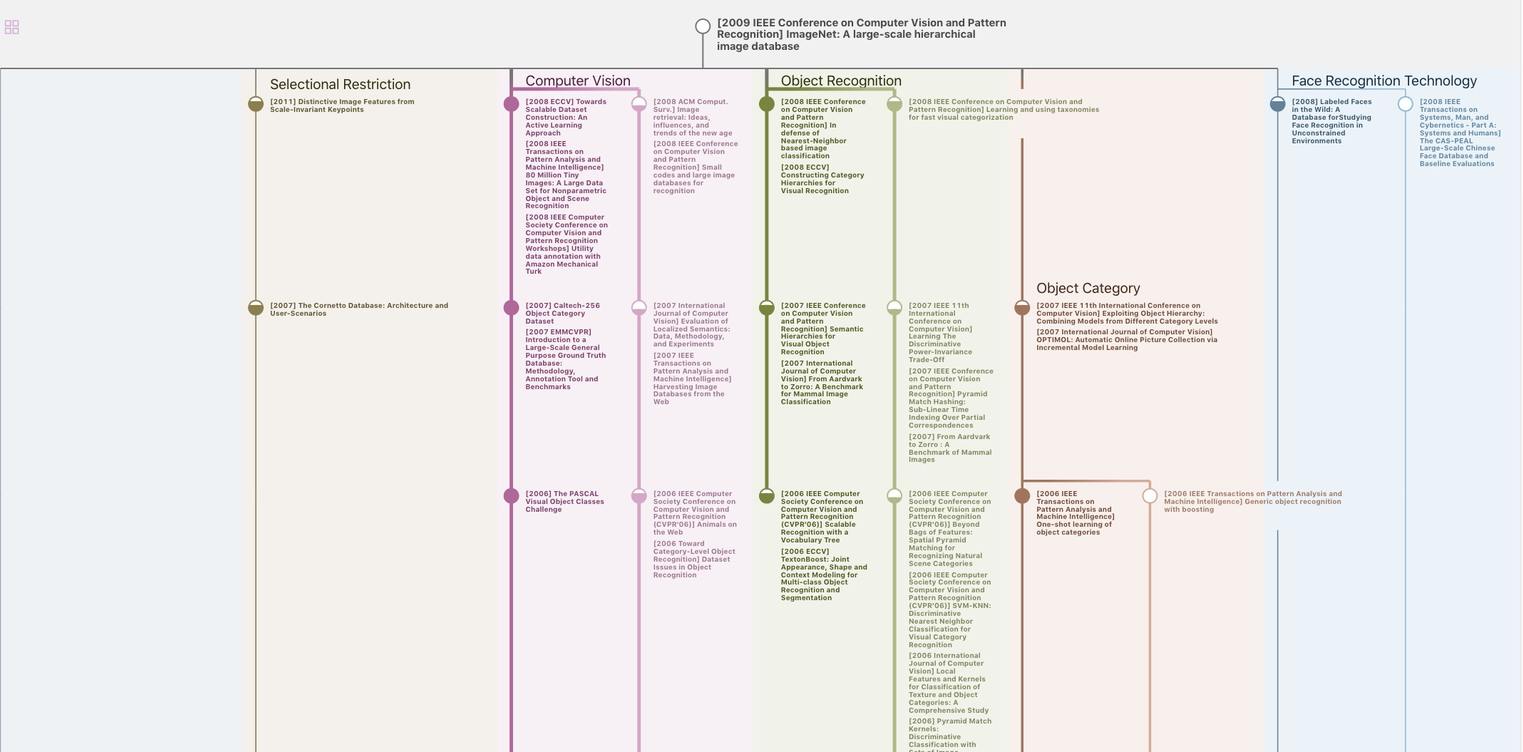
生成溯源树,研究论文发展脉络
Chat Paper
正在生成论文摘要