The Interplay Between Implicit Bias and Benign Overfitting in Two-Layer Linear Networks
arxiv(2022)
摘要
The recent success of neural network models has shone light on a rather surprising sta-tistical phenomenon: statistical models that perfectly fit noisy data can generalize well to unseen test data. Understanding this phenomenon of benign overfitting has attracted intense theoretical and empirical study. In this paper, we consider interpolating two-layer linear neural networks trained with gradient flow on the squared loss and derive bounds on the excess risk when the covariates satisfy sub-Gaussianity and anti-concentration prop-erties, and the noise is independent and sub-Gaussian. By leveraging recent results that characterize the implicit bias of this estimator, our bounds emphasize the role of both the quality of the initialization as well as the properties of the data covariance matrix in achieving low excess risk.
更多查看译文
关键词
implicit bias, generalization, benign overfitting, interpolation, neural net-works, regression
AI 理解论文
溯源树
样例
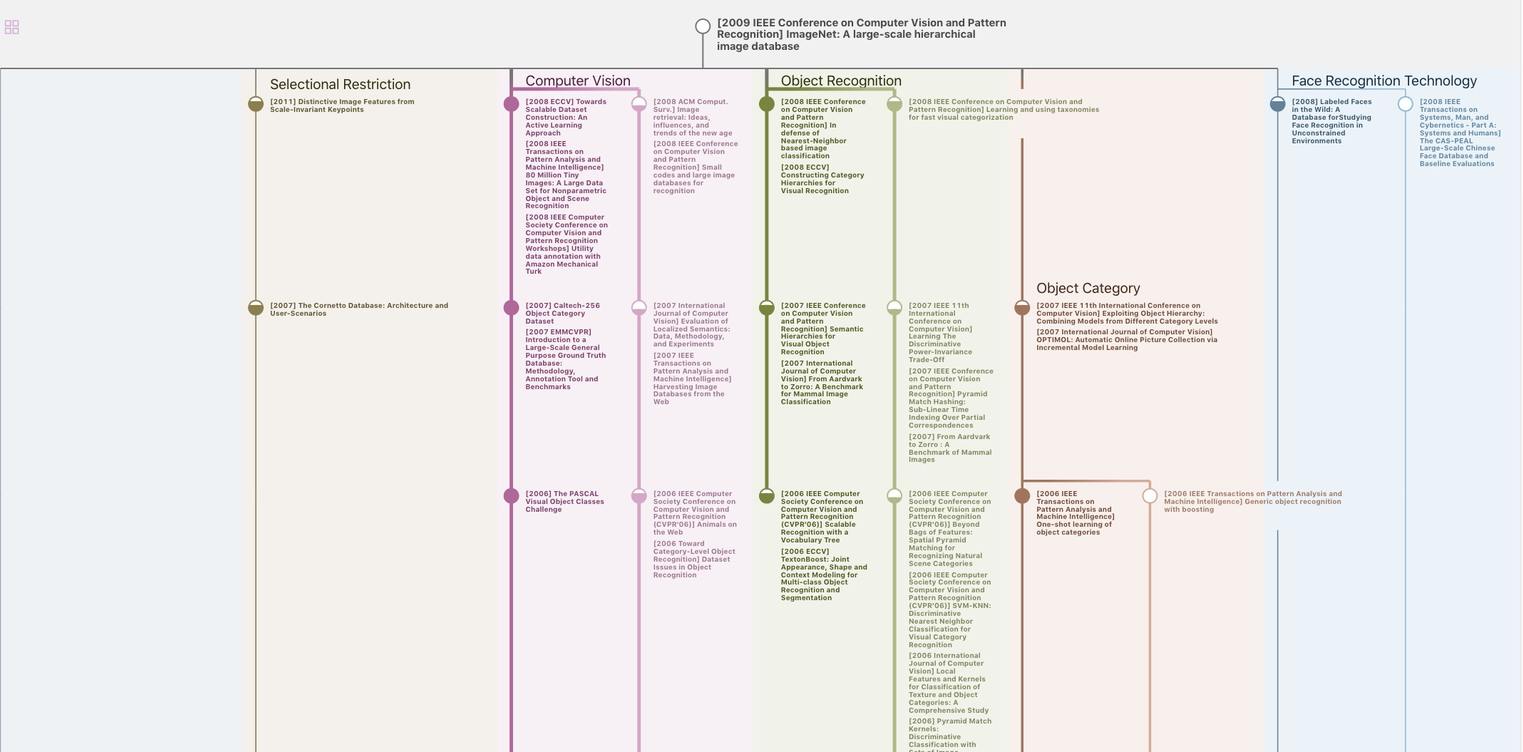
生成溯源树,研究论文发展脉络
Chat Paper
正在生成论文摘要