Machine Learning for Sensor Transducer Conversion Routines
IEEE Embedded Systems Letters(2022)
摘要
Sensors with digital outputs require software conversion routines to transform the unitless analog-to-digital converter samples to physical quantities with correct units. These conversion routines are computationally complex given the limited computational resources of low-power embedded systems. This letter presents a set of machine learning methods to learn new, less-complex conversion routines that do not sacrifice accuracy for the BME680 environmental sensor. We present a Pareto analysis of the tradeoff between accuracy and computational overhead for the models and models that reduce the computational overhead of the existing industry-standard conversion routines for temperature, pressure, and humidity by 62%, 71%, and 18%, respectively. The corresponding RMS errors are 0.0114 °C, 0.0280 KPa, and 0.0337%. These results show that machine learning methods for learning conversion routines can produce conversion routines with a reduced computational overhead which maintain good accuracy.
更多查看译文
关键词
Machine learning,regression,sensor
AI 理解论文
溯源树
样例
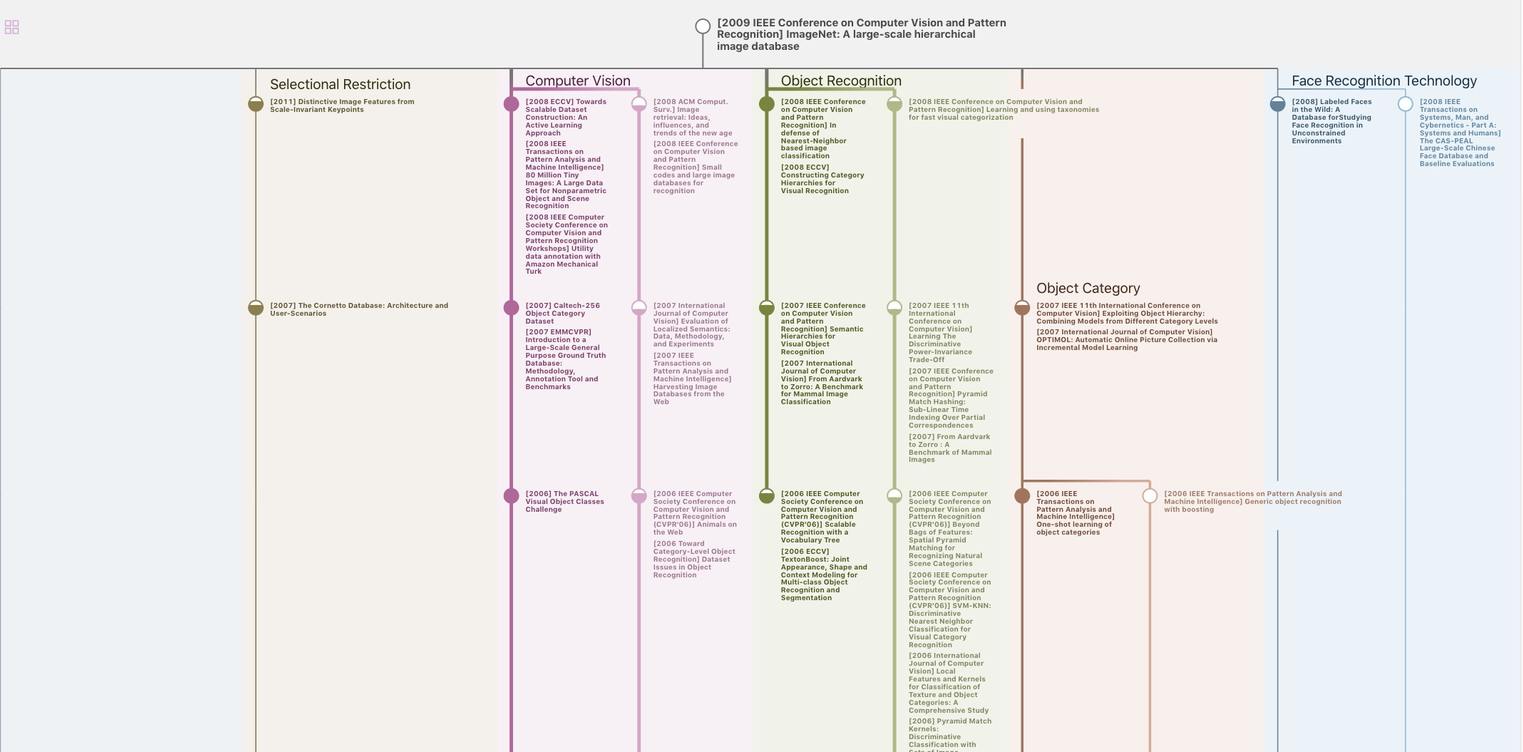
生成溯源树,研究论文发展脉络
Chat Paper
正在生成论文摘要