Task-Coupling Elastic Learning for Physical Sign-Based Medical Image Classification
IEEE Journal of Biomedical and Health Informatics(2022)
摘要
Physical signs of patients indicate crucial evidence for diagnosing both location and nature of the disease, where there is a sequential relationship between the two tasks. Thus their joint learning can utilize intrinsic association by transferring related knowledge across relevant tasks. Choosing the right time to transfer is a critical problem for joint learning. However, how to dynamically adjust when tasks interact to capture the right time for transferring related knowledge is still an open issue. To this end, we propose a Task-Coupling Elastic Learning (TCEL) framework to model the task relatedness for classifying disease-location and disease-nature based on physical sign images. The main idea is to dynamically transfer relevant knowledge by progressively shifting task-coupling from loose to tight during the multi-stage training. In the early stage of training, we relax the constraints of modeling relations to focus more in learning the generic task-common features. In the later stage, the semantic guidance will be strengthened to learn the task-specific features. Specifically, a dynamic sequential module (DSM) is proposed to explicitly model the sequential relationship and enable multi-stage training. Moreover, to address the side effect of DSM, a new loss regularization is proposed. The extensive experiments on these two clinical datasets show the superiority of the proposed method over the baselines, and demonstrate the effectiveness of the proposed task-coupling elastic mechanism.
更多查看译文
关键词
Multi-task learning,task-coupling,multi-stage learning,physical sign,medical image
AI 理解论文
溯源树
样例
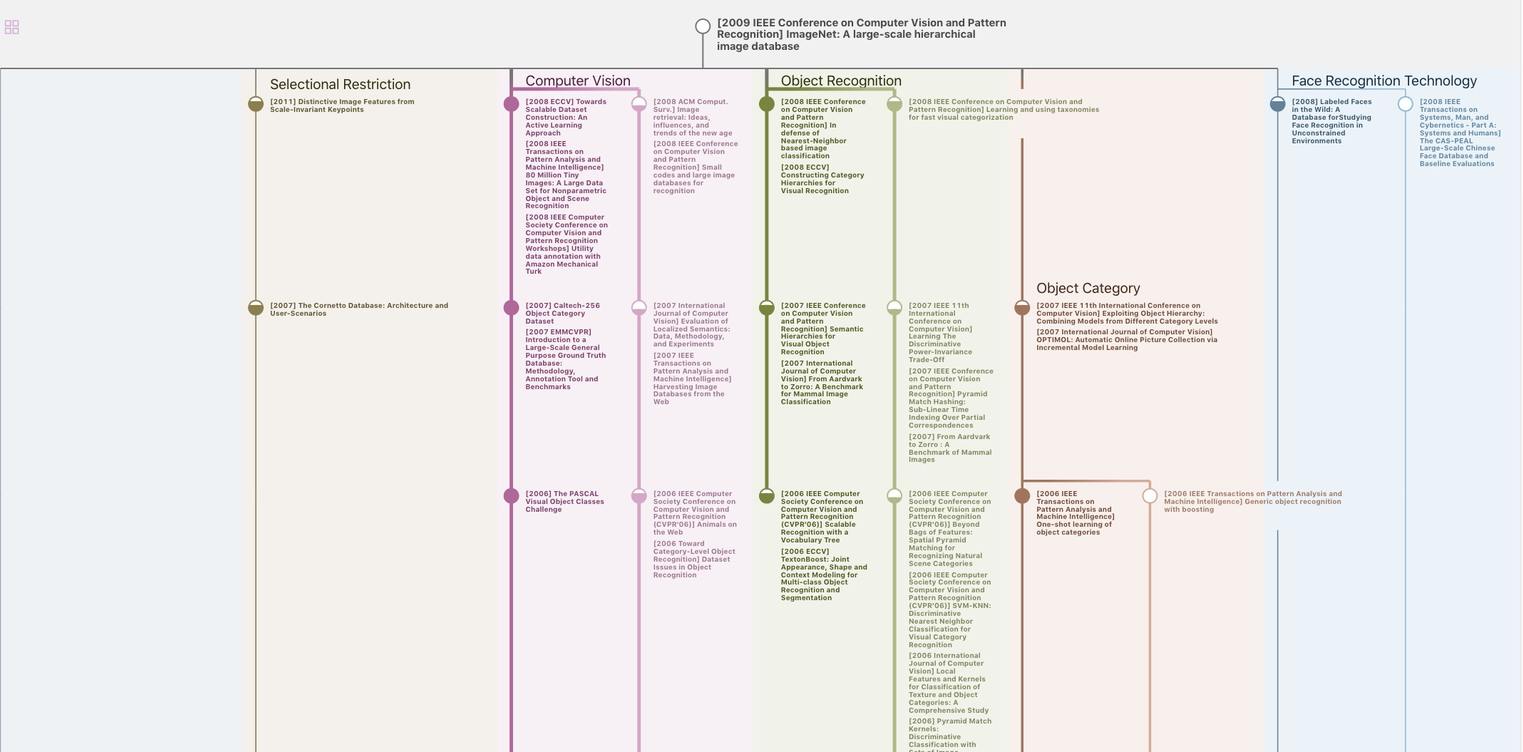
生成溯源树,研究论文发展脉络
Chat Paper
正在生成论文摘要