Understanding Longitudinal Dynamics of Recommender Systems with Agent-Based Modeling and Simulation
arxiv(2021)
摘要
Today's research in recommender systems is largely based on experimental designs that are static in a sense that they do not consider potential longitudinal effects of providing recommendations to users. In reality, however, various important and interesting phenomena only emerge or become visible over time, e.g., when a recommender system continuously reinforces the popularity of already successful artists on a music streaming site or when recommendations that aim at profit maximization lead to a loss of consumer trust in the long run. In this paper, we discuss how Agent-Based Modeling and Simulation (ABM) techniques can be used to study such important longitudinal dynamics of recommender systems. To that purpose, we provide an overview of the ABM principles, outline a simulation framework for recommender systems based on the literature, and discuss various practical research questions that can be addressed with such an ABM-based simulation framework.
更多查看译文
关键词
recommender systems,longitudinal dynamics,modeling,agent-based
AI 理解论文
溯源树
样例
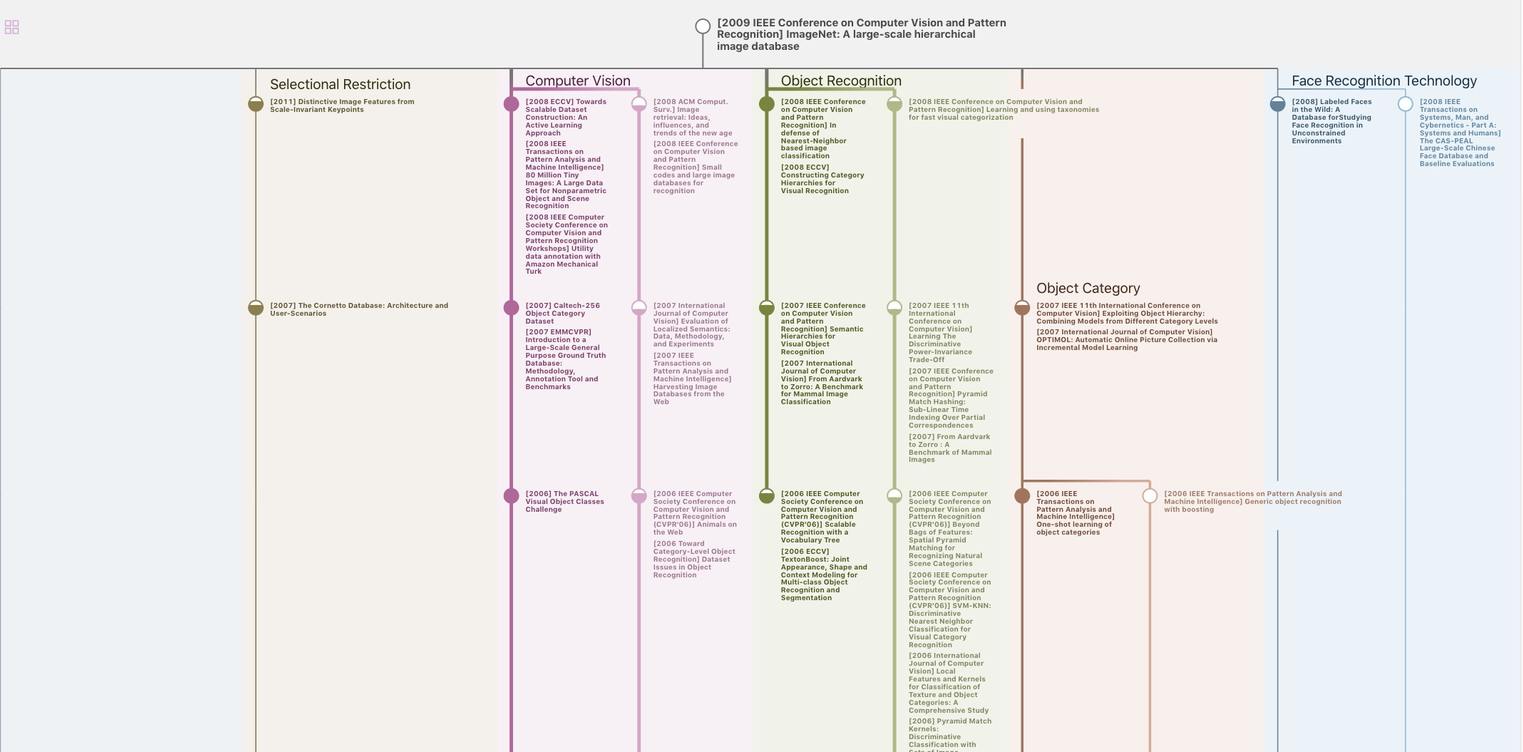
生成溯源树,研究论文发展脉络
Chat Paper
正在生成论文摘要