EviDR - Evidence-Emphasized Discrete Reasoning for Reasoning Machine Reading Comprehension.
NLPCC(2021)
摘要
Reasoning machine reading comprehension (R-MRC) aims to answer complex questions that require discrete reasoning based on text. To support discrete reasoning, evidence, typically the concise textual fragments that describe question-related facts, including topic entities and attribute values, are crucial clues from question to answer. However, previous end-to-end methods that achieve state-of-the-art performance rarely solve the problem by paying enough emphasis on the modeling of evidence, missing the opportunity to further improve the model's reasoning ability for R-MRC. To alleviate the above issue, in this paper, we propose an evidence-emphasized discrete reasoning approach (EviDR), in which sentence and clause level evidence is first detected based on distant supervision, and then used to drive a reasoning module implemented with a relational heterogeneous graph convolutional network to derive answers. Extensive experiments are conducted on DROP (discrete reasoning over paragraphs) dataset, and the results demonstrate the effectiveness of our proposed approach. In addition, qualitative analysis verifies the capability of the proposed evidence-emphasized discrete reasoning for R-MRC.
更多查看译文
关键词
reading comprehension,discrete reasoning,evidence-emphasized
AI 理解论文
溯源树
样例
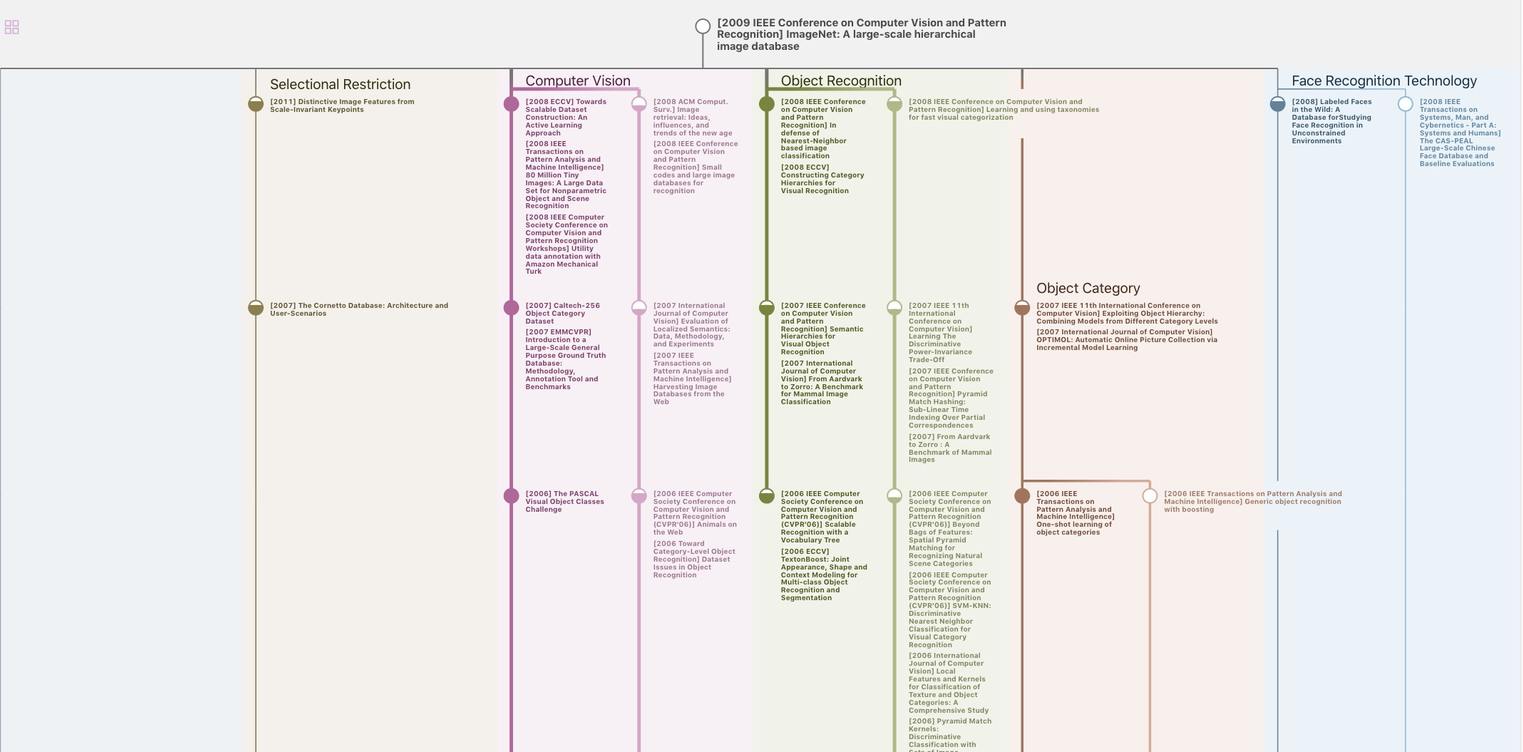
生成溯源树,研究论文发展脉络
Chat Paper
正在生成论文摘要