Event Extraction by Associating Event Types and Argument Roles
IEEE TRANSACTIONS ON BIG DATA(2023)
摘要
Event extraction (EE), which acquires structural event knowledge from texts, can be divided into two sub-tasks: event type classification and element extraction (namely identifying triggers and arguments under different role patterns). As different event types always own distinct extraction schemas (i.e., role patterns), previous work on EE usually follows an isolated learning paradigm, performing element extraction independently for different event types. It ignores meaningful associations among event types and argument roles, leading to relatively poor performance for less frequent types/roles. This paper proposes a novel neural association framework for the EE task. Given a document, it first performs type classification via constructing a document-level event graph to associate sentence nodes of different types and adopting a document-awared graph attention network to learn sentence embeddings. Then, element extraction is achieved by building a new schema of argument roles, with a type-awared parameter inheritance mechanism to enhance role preference for extracted elements. As such, our model takes into account type and role associations during EE, enabling implicit information sharing among them. Experimental results show that our approach consistently outperforms most state-of-the-art EE methods in both sub-tasks, especially at least 2.51% and 1.12% improvement of the event trigger identification and argument role classification sub-tasks. Particularly, for types/roles with less training data, the performance is superior to the existing methods.
更多查看译文
关键词
Task analysis,Data mining,Big Data,Social networking (online),Instruments,Feature extraction,Bit error rate,Event extraction,argument roles,graph attention network,new schema,parameter inheritance
AI 理解论文
溯源树
样例
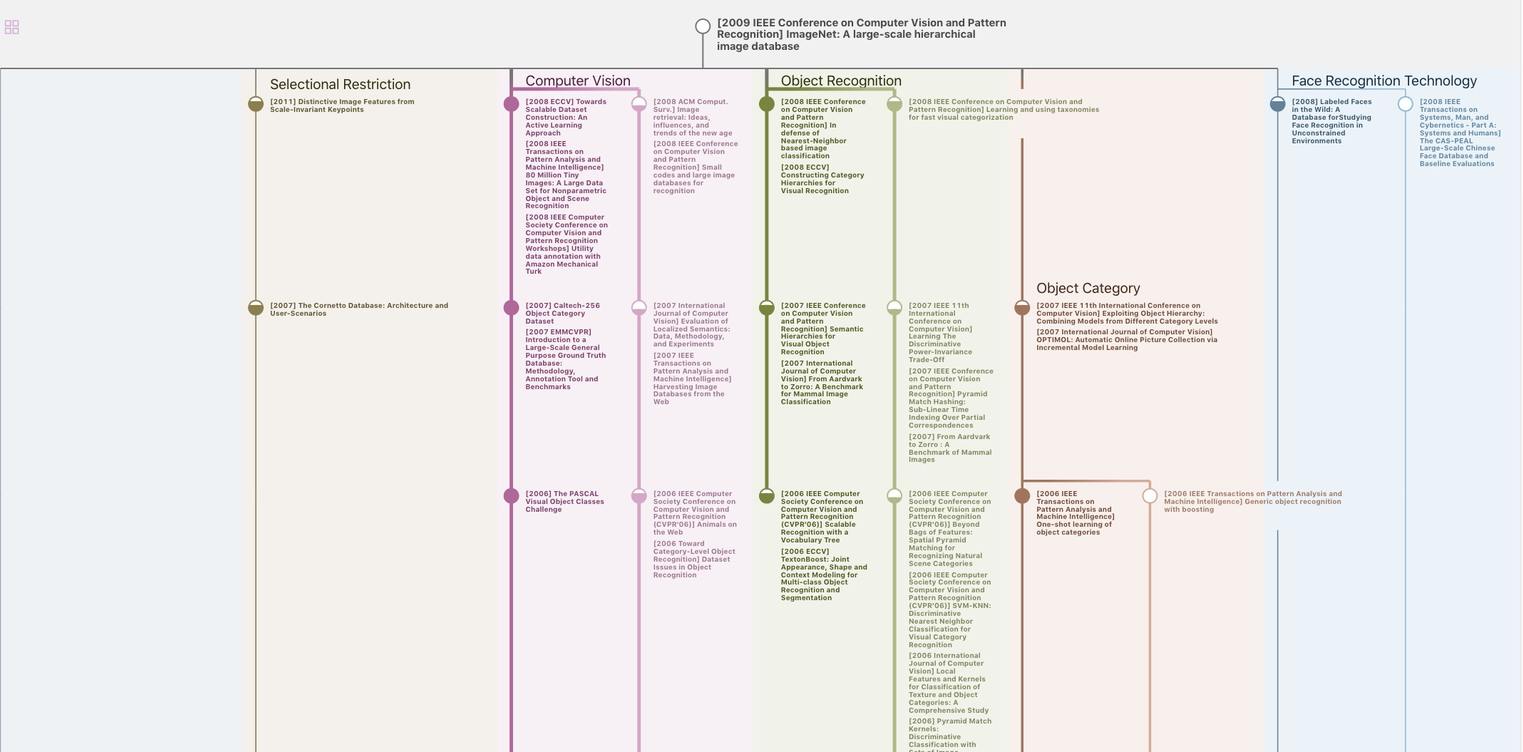
生成溯源树,研究论文发展脉络
Chat Paper
正在生成论文摘要