Model-Based Sparse Coding Beyond Gaussian Independent Model
COMPUTATIONAL STATISTICS & DATA ANALYSIS(2022)
摘要
Sparse coding aims to model data vectors as sparse linear combinations of basis elements, but a majority of related studies are restricted to continuous data without spatial or temporal structure. A new model-based sparse coding (MSC) method is proposed to provide an effective and flexible framework for learning features from different data types: continuous, discrete, or categorical, and modeling different types of correlations: spatial or temporal. The specification of the sparsity level and how to adapt the estimation method to large-scale studies are also addressed. A fast EM algorithm is proposed for estimation, and its superior performance is demonstrated in simulation and multiple real applications such as image denoising, brain connectivity study, and spatial transcriptomic imaging. (C) 2021 Elsevier B.V. All rights reserved.
更多查看译文
关键词
Fast EM algorithm, Probabilistic model, Sparse coding
AI 理解论文
溯源树
样例
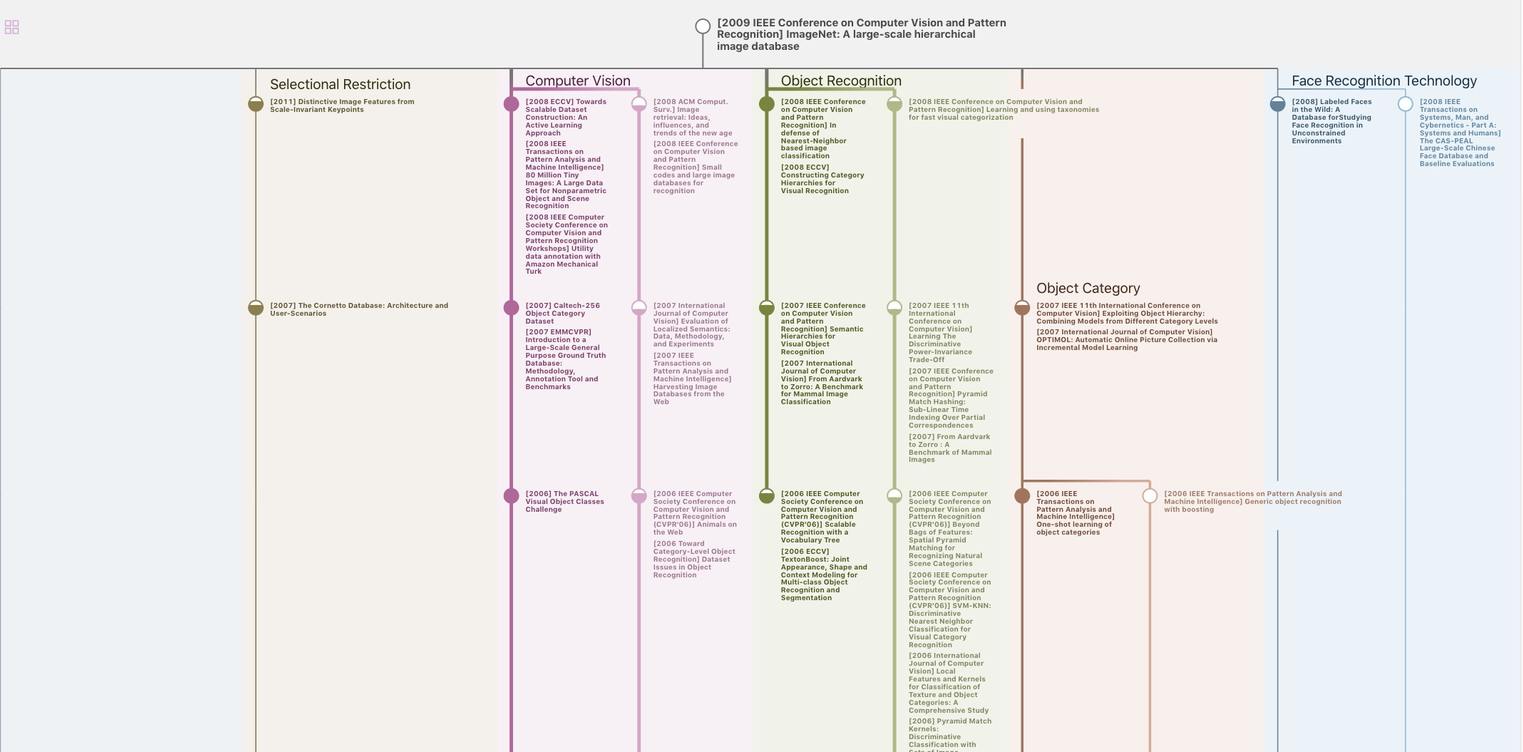
生成溯源树,研究论文发展脉络
Chat Paper
正在生成论文摘要