Weakly Supervised Extractive Summarization with Attention
SIGDIAL 2021: 22ND ANNUAL MEETING OF THE SPECIAL INTEREST GROUP ON DISCOURSE AND DIALOGUE (SIGDIAL 2021)(2021)
摘要
Automatic summarization aims to extract important information from large amounts of textual data in order to create a shorter version of the original texts while preserving its information. Training traditional extractive summarization models relies heavily on humanengineered labels such as sentence-level annotations of summary-worthiness. However, in many use cases, such human-engineered labels do not exist and manually annotating thousands of documents for the purpose of training models may not be feasible. On the other hand, indirect signals for summarization are often available, such as agent actions for customer service dialogues, headlines for news articles, diagnosis for Electronic Health Records, etc. In this paper, we develop a general framework that generates extractive summarization as a byproduct of supervised learning tasks for indirect signals via the help of attention mechanism. We test our models on customer service dialogues and experimental results demonstrated that our models can reliably select informative sentences and words for automatic summarization.
更多查看译文
关键词
weakly,attention
AI 理解论文
溯源树
样例
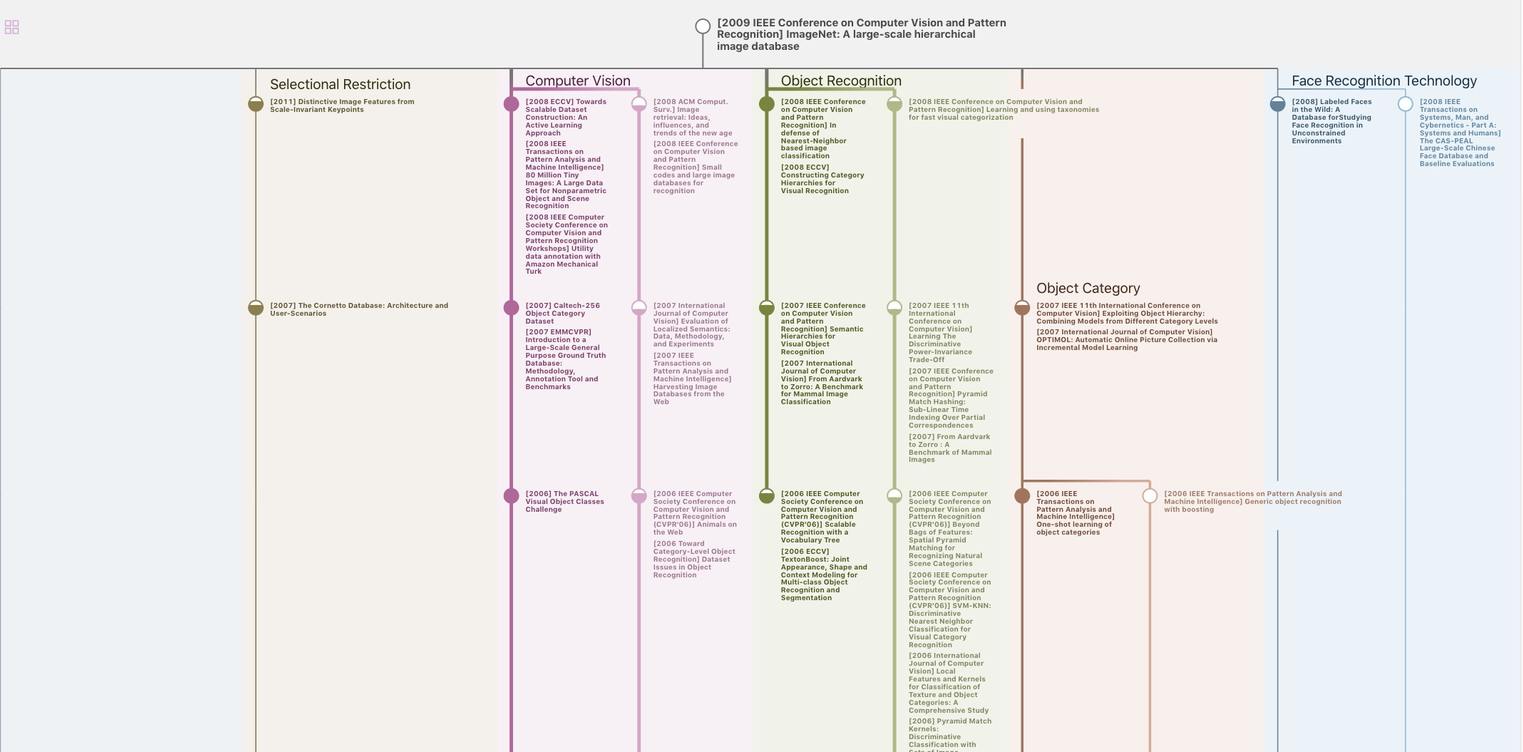
生成溯源树,研究论文发展脉络
Chat Paper
正在生成论文摘要