Automatic extraction of associated fact elements from civil cases based on a deep contextualized embeddings approach: KGCEE
SOFT COMPUTING(2021)
摘要
Automatic factor extraction is to extract the relevant facts from the case to assist the judge in the intelligent decision-making of civil disputes. Previously, the existing methods mainly focus on context-free word embeddings to deal with extraction tasks in the field of law, which cannot get a better semantic understanding of the text and in turn leads to an adverse extraction performance. Therefore, in this paper, a deep contextualized embeddings-based method called the knowledge-guided civil case fact elements extraction (KGCEE) model to automatically extract civil fact elements in the civil case domain is proposed. This approach is mainly based on the RoBERTa, but a few techniques make a more powerful model. Firstly, the model is retrained with civil domain data to provide more sensitive weight to initialize the model parameters in the downstream task. Secondly, the extraction is transformed into a sentence pairs task and we have incorporated data by leveraging label information to improve the generalization ability of the model. Thirdly, at the beginning of the KGCEE, we propose to inject part-of-speech information to the word embeddings to enhance the ability to capture the semantic and syntactic information, which aims to obtain better text representations. Finally, the KGCEE method is evaluated under civil domain data such as marriage and family, labor disputes and loan contracts originally from Chinese AI and Law (CAIL). The experimental results demonstrate that our KGCEE method outperforms other context-free word embeddings methods and other traditional transformer-based methods.
更多查看译文
关键词
Civil domain,Fact elements,Fine-tuned RoBERTa model,Knowledge-guided civil case elements extraction,Deep contextualized embeddings,Sentence pairs
AI 理解论文
溯源树
样例
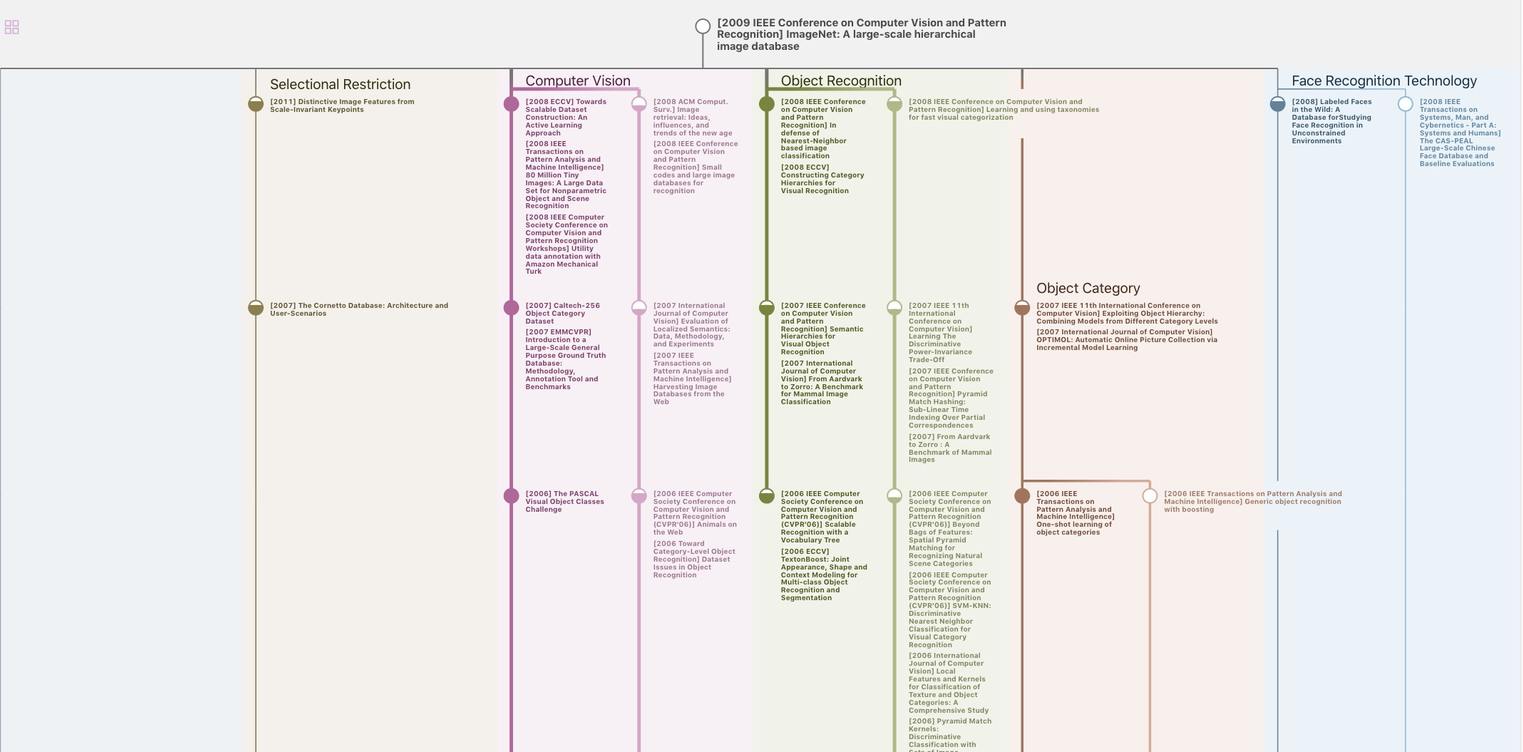
生成溯源树,研究论文发展脉络
Chat Paper
正在生成论文摘要