Automatic modeling and fault diagnosis of car production lines based on first-principle qualitative mechanics and semantic web technology
Advanced Engineering Informatics(2021)
摘要
Fault diagnosis is critical for intelligent manufacturing by monitoring the status of a production line and preventing financial loss. Model-based fault diagnosis has the advantage of being able to explain the cause and propagation of faults over model-free diagnosis, but would need knowledge about the configuration model and context-specific information of the production line. Ontology modelling can provide context-specific information on top of a configuration model to benefit fault diagnosis. Typically ontologies are manually constructed and then used by a reasoner based on a set of predefined rules. From the perspective of fault diagnosis, this approach works as an expert system where both the ontology models and predefined rules are specific to a given system. Once the system has changed which happens from time to time as repairs and updates in a production line, or in the case of a different system, the ontology models and predefined rules would need to be manually modified or reconstructed. Here a model-based method is proposed to automate generation of configuration models with context-specific information using semantic web technology when a production line is healthy, and to use the generated configuration model and information for diagnosis when the production line has a fault. The method does not rely on predefined rules and reasoners, but rather uses dynamics models that are based on first-principle qualitative mechanics. It uses numerical optimization to minimize the discrepancy between sensor data from the production line and from simulation running the dynamics model to achieve automatic configuration modelling and fault diagnosis. With three use cases commonly found for a production line, i.e. automatic sensor placement modeling or misplacement diagnosis, motor fault diagnosis with single sensor modality, and motor fault diagnosis with sensory substitution, the feasibility of the proposed method is demonstrated. The method’s faster computational speed and comparable accuracy to a quantitative model-based approach suggests it may complement and accelerate the latter with early-stage selection of candidate models for both modelling and fault diagnosis.
更多查看译文
关键词
Fault diagnosis,Intelligent manufacturing systems,Numerical optimization,Semantic web,Ontology,Time series analysis
AI 理解论文
溯源树
样例
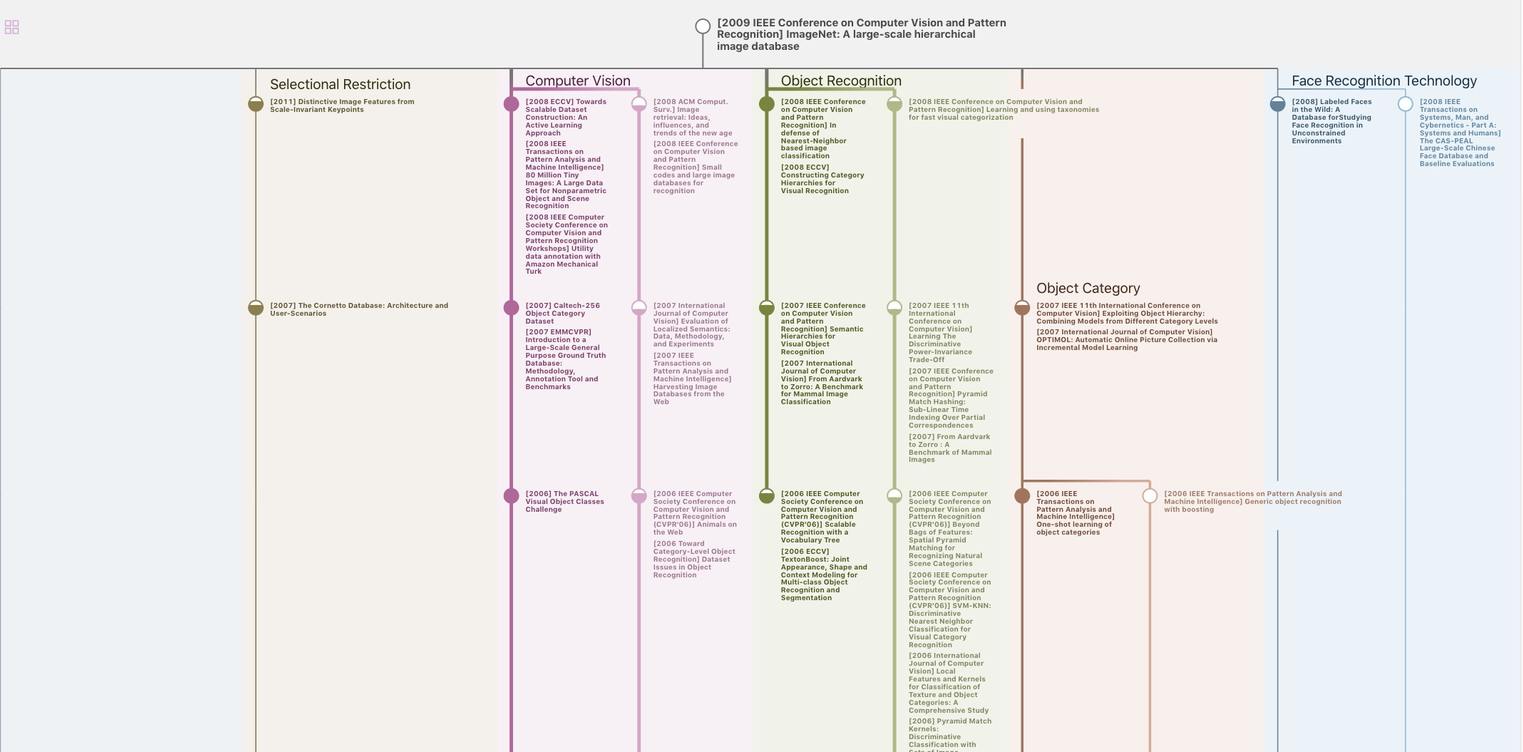
生成溯源树,研究论文发展脉络
Chat Paper
正在生成论文摘要