Discriminative deep learning based benignity/malignancy diagnosis of dermatologic ultrasound skin lesions with pretrained artificial intelligence architecture
SKIN RESEARCH AND TECHNOLOGY(2022)
摘要
Background Deep-learning algorithms (DLAs) have been used in artificial intelligence aided ultrasonography diagnosis of thyroid and breast lesions. However, its use has not been described in the case of dermatologic ultrasound lesions. Our purpose was to train a DLA to discriminate benign form malignant lesions in dermatologic ultrasound images. Materials and methods We trained a prebuilt neural network architecture (EfficientNet B4) in a commercial artificial intelligence platform (Peltarion, Stockholm, Sweden) with 235 color Doppler images of both benign and malignant ultrasound images of 235 excised and histologically confirmed skin lesions (84.3% training, 15.7% validation). An additional 35 test images were used for testing the algorithm discrimination for correct benign/malignant diagnosis. One dermatologist with more than 5 years of experience in dermatologic ultrasound blindly evaluated the same 35 test images for malignancy or benignity. Results EfficientNet B4 trained dermatologic ultrasound algorithm sensitivity; specificity; predictive positive values, and predicted negative values for validation algorithm were 0.8, 0.86, 0.86, and 0.8, respectively for malignancy diagnosis. When tested with 35 previously unevaluated images sets, the algorithm ' s accuracy for correct benign/malignant diagnosis was 77.1%, not statistically significantly different from the dermatologist's evaluation (74.1%). Conclusion An adequately trained algorithm, even with a limited number of images, is at least as accurate as a dermatologic-ultrasound experienced dermatologist in the evaluation of benignity/malignancy of ultrasound skin tumor images devoid of clinical data.
更多查看译文
关键词
artificial intelligence, deep learning, dermatologic ultrasound, skin ultrasound
AI 理解论文
溯源树
样例
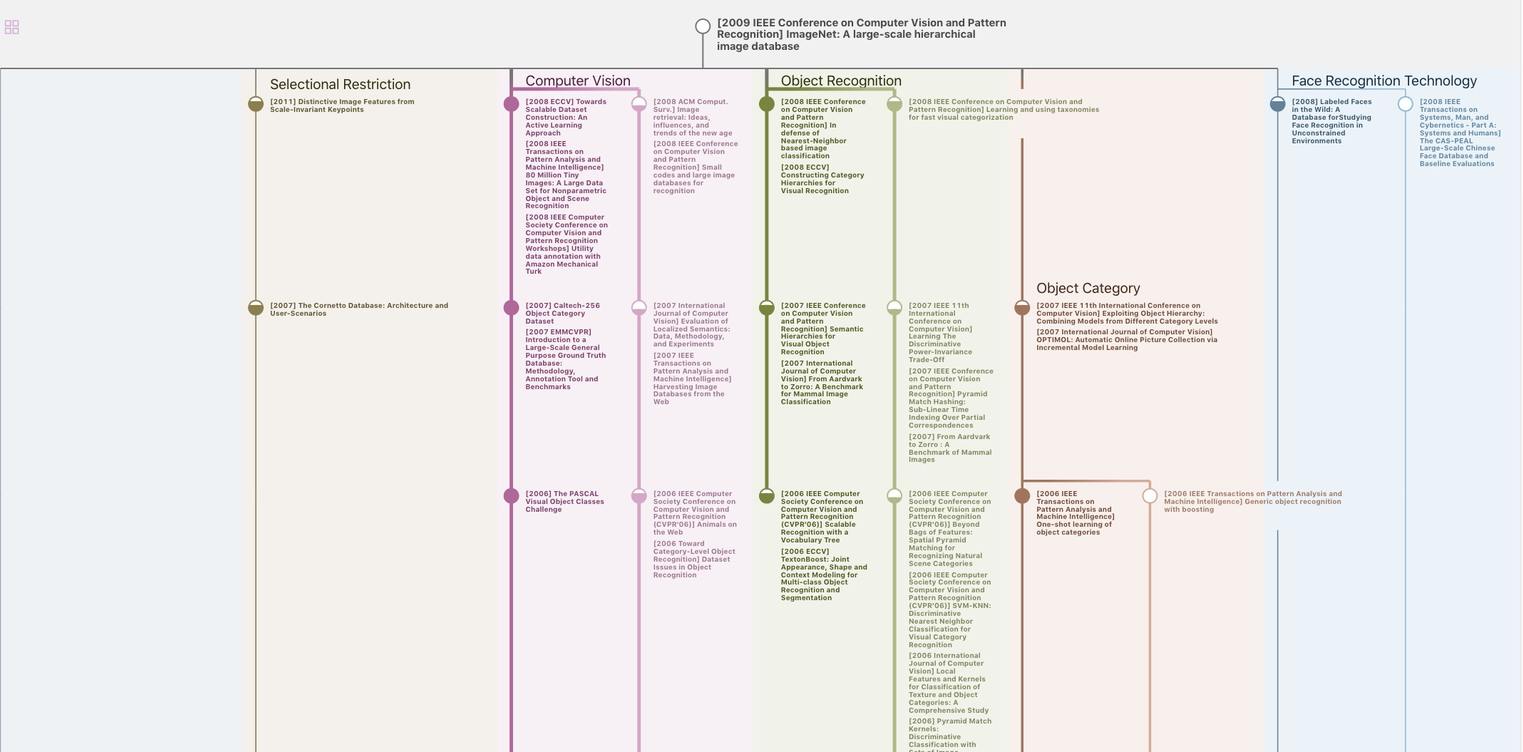
生成溯源树,研究论文发展脉络
Chat Paper
正在生成论文摘要