A Random Forest Model For The Classification Of Wheat And Rye Leaf Rust Symptoms Based On Pure Spectra At Leaf Scale
JOURNAL OF PHOTOCHEMISTRY AND PHOTOBIOLOGY B-BIOLOGY(2021)
摘要
The pure spectra acquisition of plant disease symptoms is essential to improving the reliability of remote sensing methods in crop protection. The reflectance values read from the pure spectra can be used as valuable training data for development of algorithms designed for plant disease detection at leaf and canopy scale. The aim of this paper is to identify and distinguish spectrally the leaf rust symptoms caused by two closely related special forms (f. sp.) of Puccinia recondita f. sp. tritici on wheat and Puccinia recondita f. sp. recondita on rye at leaf scale. Spectral measurements were made with FieldSpec 3 spectrometer in the wavelength range of 350-2500 nm. The spectrometer was connected to a microscope by optical fiber. Raw spectra of uredinia, chlorotic discoloration, green leaves, senescent inoculated leaves and senescent uninoculated leaves of wheat and rye, all of which obtained for this study, were investigated with a view towards making an automized classification of plant species and their phases. The created Random Forest models were tested separately using pure spectra, and from these vegetation indices were derived as predictors. Three vegetation indices, namely CRI, PRI and GNDVI, appeared to be the most robust in terms of distinguishing uredinia from other symptoms on rye and wheat leaves. PRI, EVI, NDVI705, and GNDVI were the most suitable for distinguishing uredinia, chlorotic discoloration, and green leaf stages on rye. That tusk on wheat leaves can be recognized if seven indices (PRI, MSAWI, SAVI, NDVI, NDVI705, GNDVI and RVI) are used together. For the classification of all disease symptoms for both plant species, the most useful were wavelengths in the VIS range: 431-436, 696-703 and 646-686 nm. However, the ranges of SWIR wavelengths (1938, 1955) and NIR wavelengths (1099-1104) also have a high contribution to the discrimination accuracy of the model. In the classification of all disease symptoms, the most important vegetation indices were CRI, OSAVI, and GNDVI. Analysis of the results revealed the advantage of the model based on the selected spectral wavelengths (Hit Rate of 96.6%) in comparison with predictions based on vegetation indices alone (Hit Rate of 91.7%). Both approaches show the highly applicable character of utilizing high quality spectral products such as satellite images in reducing operational costs of crop protection.
更多查看译文
关键词
Leaf rust, Symptom discrimination, Pure spectra, Random forest, Wavebands, Vegetation indices
AI 理解论文
溯源树
样例
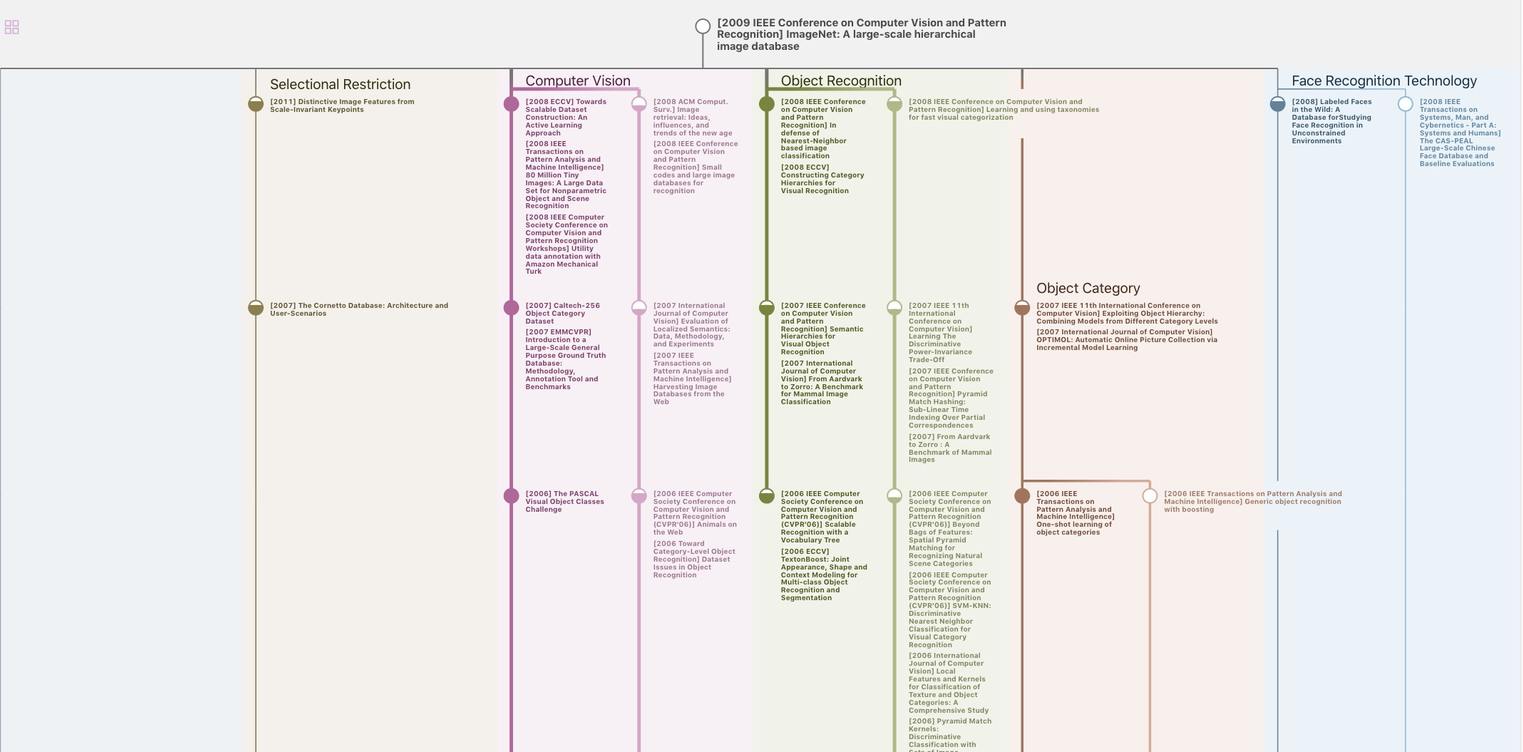
生成溯源树,研究论文发展脉络
Chat Paper
正在生成论文摘要