A Machine Learning Approach To Predict Extreme Inactivity In Copd Patients Using Non-Activity-Related Clinical Data
PLOS ONE(2021)
摘要
Facilitating the identification of extreme inactivity (EI) has the potential to improve morbidity and mortality in COPD patients. Apart from patients with obvious EI, the identification of a such behavior during a real-life consultation is unreliable. We therefore describe a machine learning algorithm to screen for EI, as actimetry measurements are difficult to implement. Complete datasets for 1409 COPD patients were obtained from COLIBRI-COPD, a database of clinicopathological data submitted by French pulmonologists. Patient- and pulmonologist-reported estimates of PA quantity (daily walking time) and intensity (domestic, recreational, or fitness-directed) were first used to assign patients to one of four PA groups (extremely inactive [EI], overtly active [OA], intermediate [INT], inconclusive [INC]). The algorithm was developed by (i) using data from 80% of patients in the EI and OA groups to identify 'phenotype signatures' of non-PA-related clinical variables most closely associated with EI or OA; (ii) testing its predictive validity using data from the remaining 20% of EI and OA patients; and (iii) applying the algorithm to identify EI patients in the INT and INC groups. The algorithm's overall error for predicting EI status among EI and OA patients was 13.7%, with an area under the receiver operating characteristic curve of 0.84 (95% confidence intervals: 0.75-0.92). Of the 577 patients in the INT/INC groups, 306 (53%) were reclassified as EI by the algorithm. Patient- and physician- reported estimation may underestimate EI in a large proportion of COPD patients. This algorithm may assist physicians in identifying patients in urgent need of interventions to promote PA.
更多查看译文
AI 理解论文
溯源树
样例
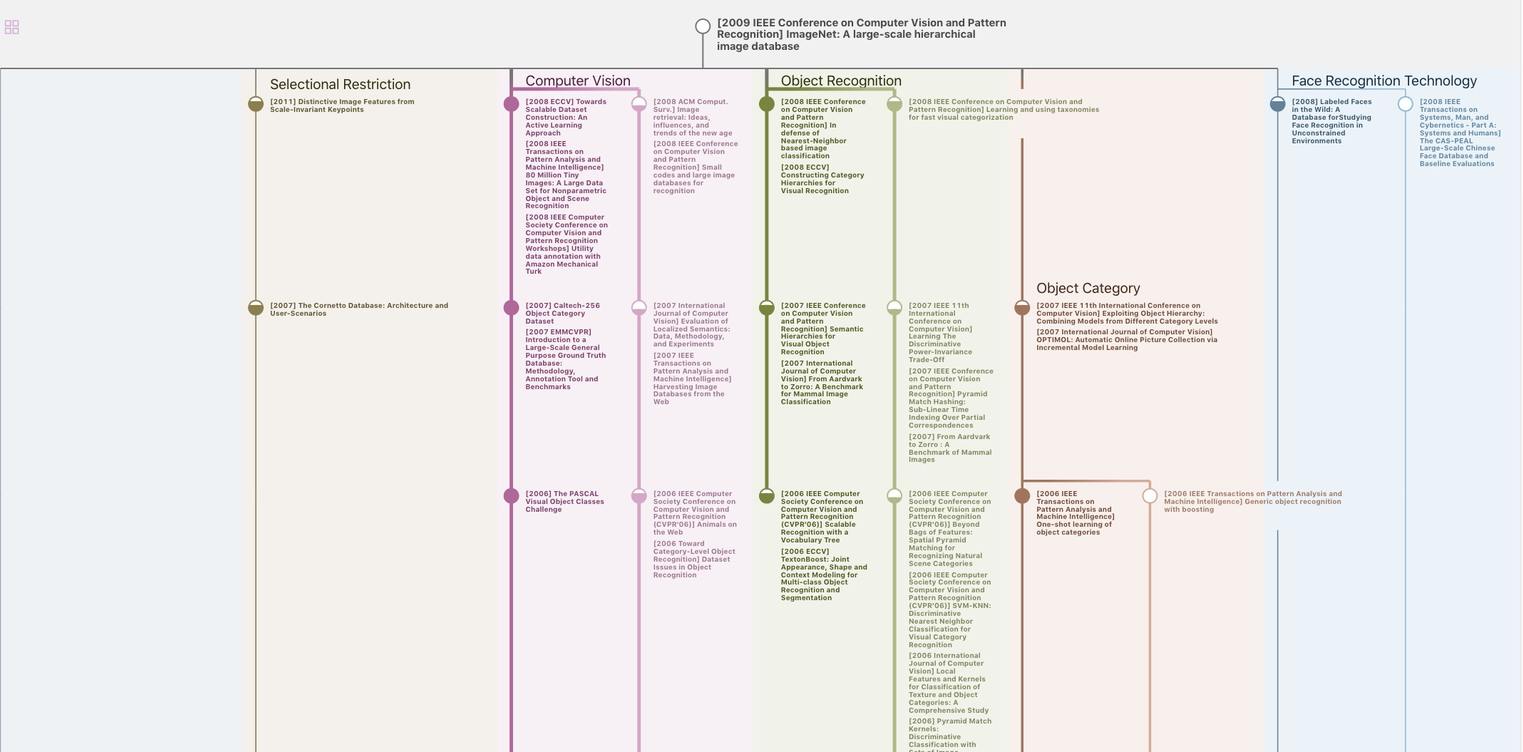
生成溯源树,研究论文发展脉络
Chat Paper
正在生成论文摘要