Survey and Evaluation of Neural 3D Shape Classification Approaches
IEEE Transactions on Pattern Analysis and Machine Intelligence(2022)
摘要
Classification of 3D objects – the selection of a category in which each object belongs – is of great interest in the field of machine learning. Numerous researchers use deep neural networks to address this problem, altering the network architecture and representation of the 3D shape used as an input. To investigate the effectiveness of their approaches, we conduct an extensive survey of existing methods and identify common ideas by which we categorize them into a taxonomy. Second, we evaluate 11 selected classification networks on two 3D object datasets, extending the evaluation to a larger dataset on which most of the selected approaches have not been tested yet. For this, we provide a framework for converting shapes from common 3D mesh formats into formats native to each network, and for training and evaluating different classification approaches on this data. Despite being partially unable to reach the accuracies reported in the original papers, we compare the relative performance of the approaches as well as their performance when changing datasets as the only variable to provide valuable insights into performance on different kinds of data. We make our code available to simplify running training experiments with multiple neural networks with different prerequisites.
更多查看译文
关键词
3D shape analysis,classification algorithms,computer graphics,convolutional neural network,deep learning,image processing,machine learning,multi-layer neural network,neural networks,object recognition
AI 理解论文
溯源树
样例
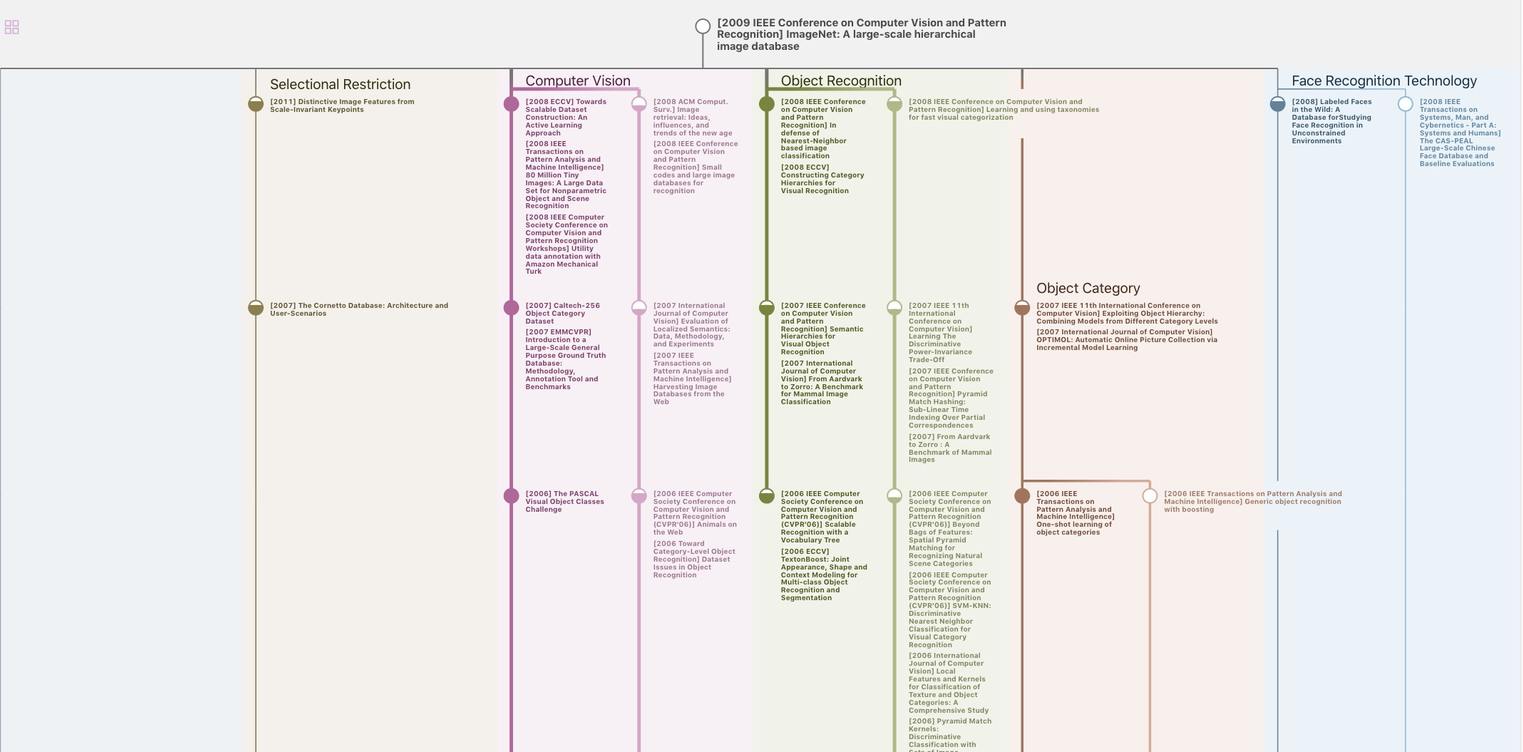
生成溯源树,研究论文发展脉络
Chat Paper
正在生成论文摘要