Gaussian Process Regression For Materials And Molecules
CHEMICAL REVIEWS(2021)
摘要
We provide an introduction to Gaussian process regression (GPR) machinelearning methods in computational materials science and chemistry. The focus of the present review is on the regression of atomistic properties: in particular, on the construction of interatomic potentials, or force fields, in the Gaussian Approximation Potential (GAP) framework; beyond this, we also discuss the fitting of arbitrary scalar, vectorial, and tensorial quantities. Methodological aspects of reference data generation, representation, and regression, as well as the question of how a data-driven model may be validated, are reviewed and critically discussed. A survey of applications to a variety of research questions in chemistry and materials science illustrates the rapid growth in the field. A vision is outlined for the development of the methodology in the years to come.
更多查看译文
关键词
gaussian process,regression,molecules,materials
AI 理解论文
溯源树
样例
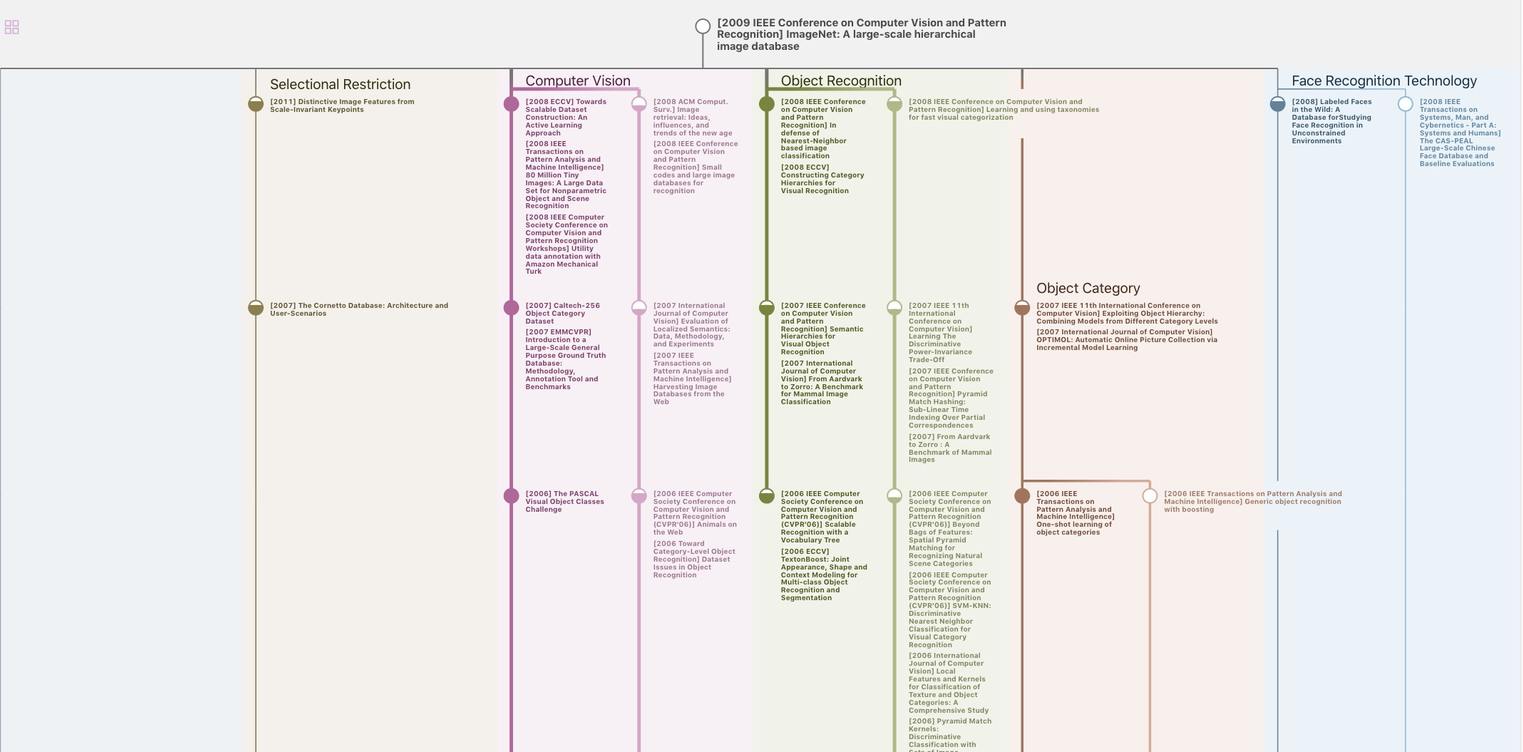
生成溯源树,研究论文发展脉络
Chat Paper
正在生成论文摘要