Near real-time streaming analysis of big fusion data
PLASMA PHYSICS AND CONTROLLED FUSION(2022)
摘要
Experiments on fusion plasmas produce high-dimensional data time series with ever-increasing magnitude and velocity, but turn-around times for analysis of this data have not kept up. For example, many data analysis tasks are often performed in a manual, ad-hoc manner some time after an experiment. In this article, we introduce the Delta framework that facilitates near real-time streaming analysis of big and fast fusion data. By streaming measurement data from fusion experiments to a high-performance compute center, Delta allows computationally expensive data analysis tasks to be performed in between plasma pulses. This article describes the modular and expandable software architecture of Delta and presents performance benchmarks of individual components as well as of an example workflow. Focusing on a streaming analysis workflow where electron cyclotron emission imaging (ECEi) data is measured at KSTAR on the National Energy Research Scientific Computing Center's (NERSC's) supercomputer we routinely observe data transfer rates of about 4 Gigabit per second. In NERSC, a demanding turbulence analysis workflow effectively utilizes multiple nodes and graphical processing units and executes them in under 5 min. We further discuss how Delta uses modern database systems and container orchestration services to provide web-based real-time data visualization. For the case of ECEi data we demonstrate how data visualizations can be augmented with outputs from machine learning models. By providing session leaders and physics operators, results of higher-order data analysis using live visualizations may make more informed decisions on how to configure the machine for the next shot.
更多查看译文
关键词
streaming analysis, machine learning, big data, fusion energy
AI 理解论文
溯源树
样例
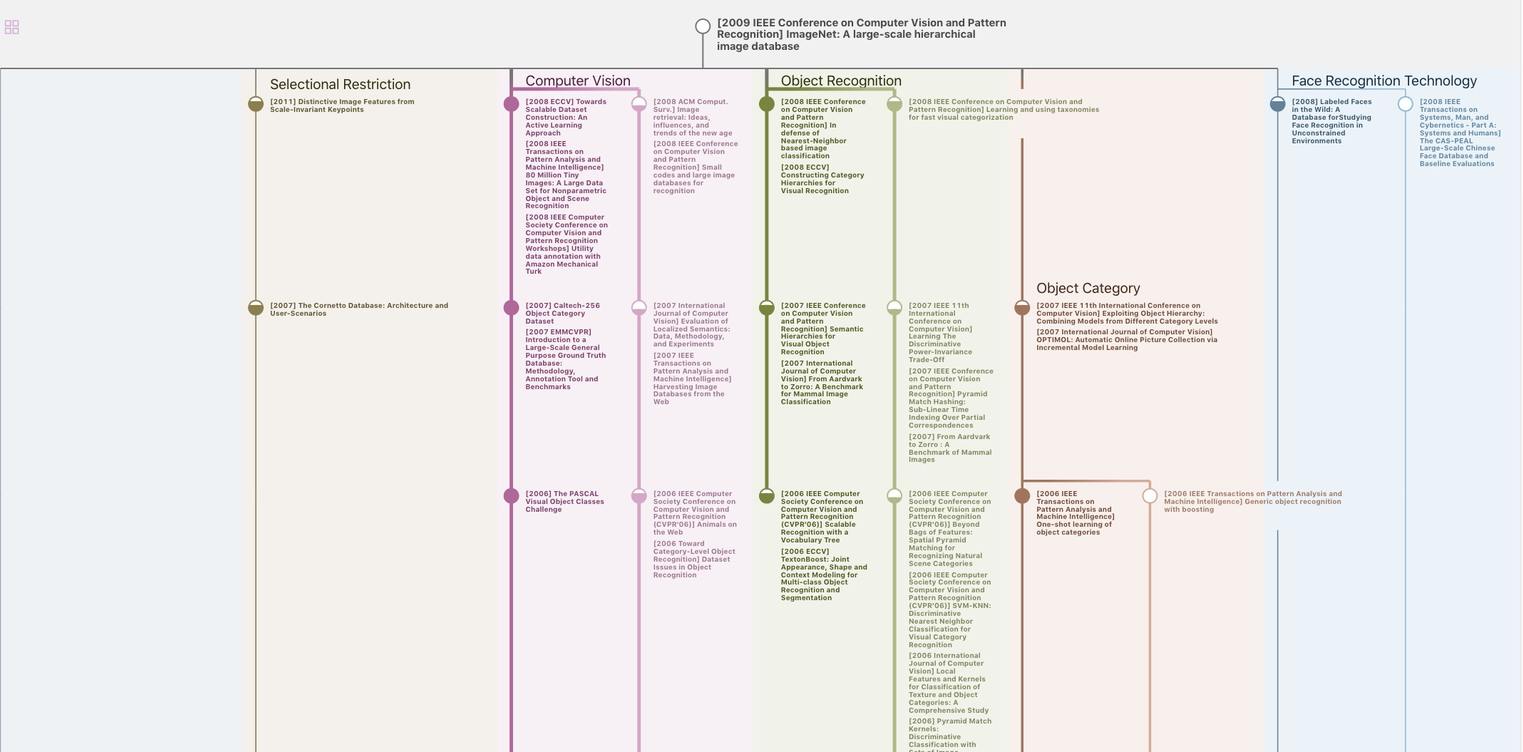
生成溯源树,研究论文发展脉络
Chat Paper
正在生成论文摘要