Interpreting and Improving Deep-Learning Models with Reality Checks.
International Conference on Machine Learning(2022)
摘要
Recent deep-learning models have achieved impressive predictive performance by learning complex functions of many variables, often at the cost of interpretability. This chapter covers recent work aiming to interpret models by attributing importance to features and feature groups for a single prediction. Importantly, the proposed attributions assign importance to interactions between features, in addition to features in isolation. These attributions are shown to yield insights across real-world domains, including bio-imaging, cosmology image and natural-language processing. We then show how these attributions can be used to directly improve the generalization of a neural network or to distill it into a simple model. Throughout the chapter, we emphasize the use of reality checks to scrutinize the proposed interpretation techniques. (Code for all methods in this chapter is available at github.com/csinva and github.com/Yu-Group, implemented in PyTorch [54]).
更多查看译文
关键词
Interpretability,Interactions,Feature importance,Neural network,Distillation
AI 理解论文
溯源树
样例
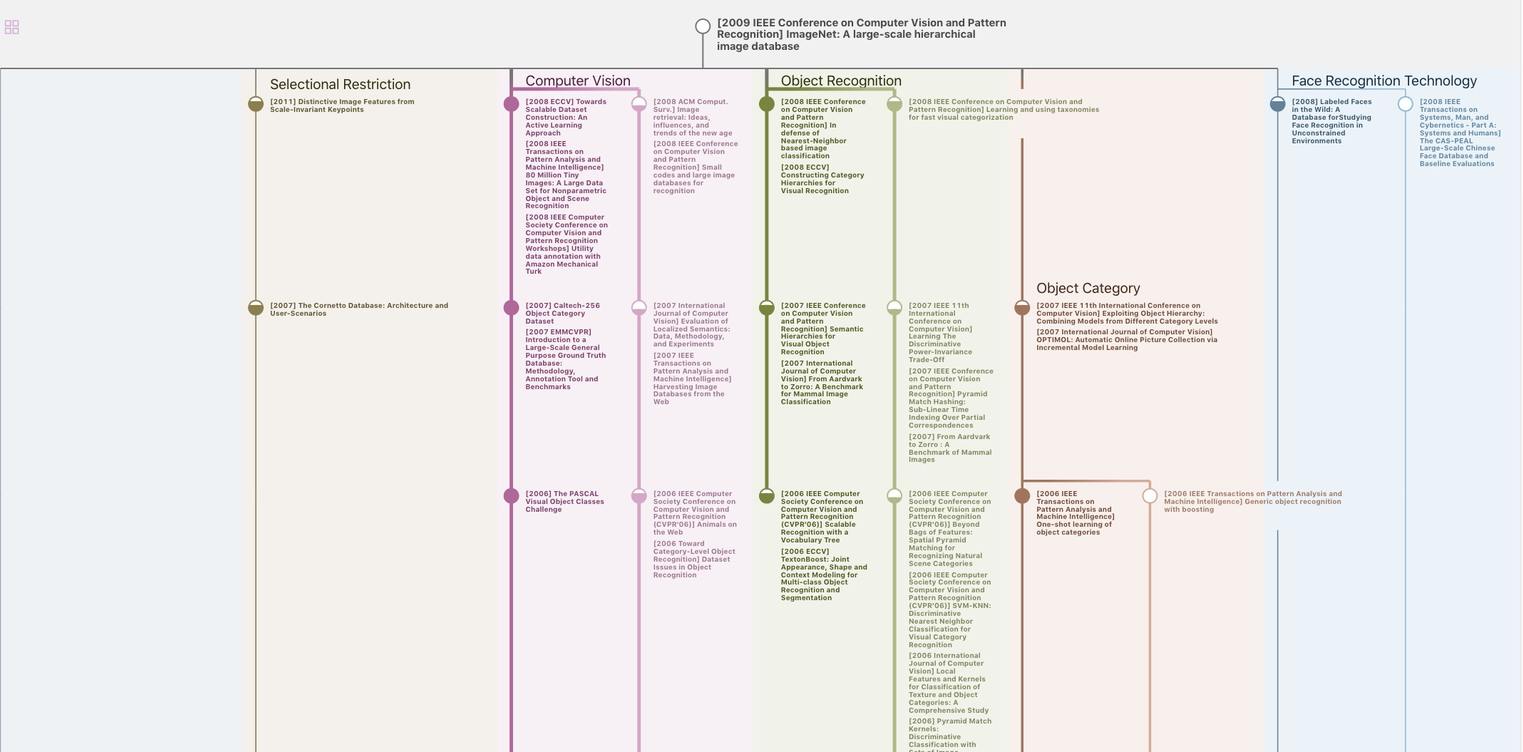
生成溯源树,研究论文发展脉络
Chat Paper
正在生成论文摘要