Re-calibrating pure risk integrating individual data from two-phase studies with external summary statistics.
Biometrics(2022)
摘要
Accurate risk assessment is critical in clinical decision-making. It entails the projected risk based on a risk prediction model agreeing with the observed risk in the target cohort. However, the model often over- or under-estimates the risk. Building a new model for the target cohort would be ideal but costly. It is therefore of great interest to recalibrate an existing model for the target cohort. Existing methods have been proposed to recalibrate the model by leveraging the disease incidence rates from the target cohort. However, they assume the same covariate distribution across cohorts and when the assumption is violated, the recalibrated model can be substantially biased. Further, recalibration is also complicated by the two-phase sampling design that is commonly used for developing risk prediction models. In this paper, we develop a weighted estimating-equation approach accounting for the two-phase design and combine it with a weighted empirical likelihood that leverages the summary information on both disease incidence rates and covariates from the target cohort. We provide a resampling-based inference procedure. Our extensive simulation results show that using the summary information from the target population, the proposed recalibration method yields nearly unbiased risk estimates under a wide range of scenarios. An application to a colorectal cancer study also illustrates that the proposed method yields a well-calibrated model in the target cohort.
更多查看译文
关键词
Cox model,baseline hazard function,empirical likelihood,external risk projection,resampling inference procedure
AI 理解论文
溯源树
样例
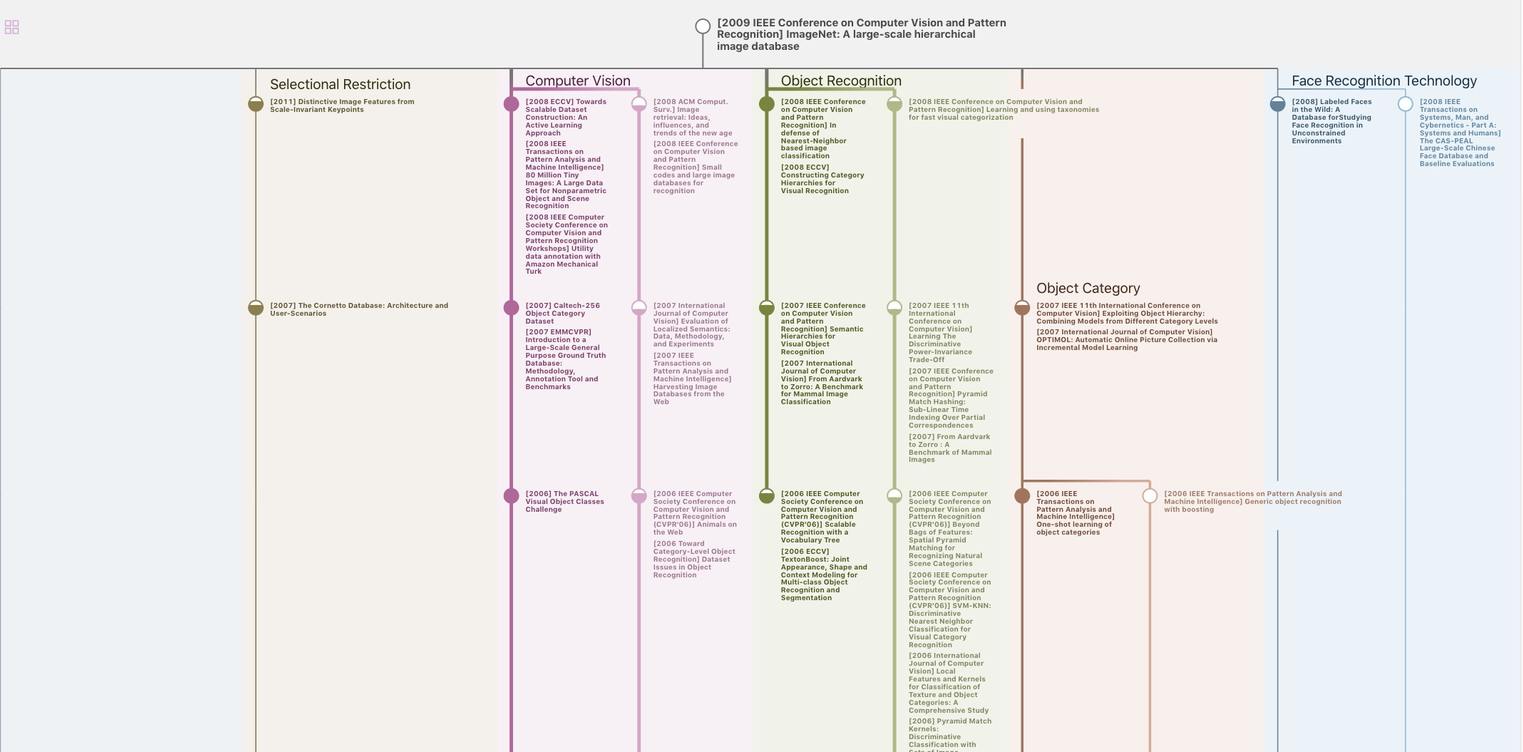
生成溯源树,研究论文发展脉络
Chat Paper
正在生成论文摘要