An Adaptive and Near Parameter-Free BRKGA Using Q-Learning Method
2021 IEEE CONGRESS ON EVOLUTIONARY COMPUTATION (CEC 2021)(2021)
摘要
The Biased Random-Key Genetic Algorithm (BRKGA) is an efficient metaheuristic to solve combinatorial optimization problems but requires parameter tuning so the intensification and diversification of the algorithm work in a balanced way. There is, however, not only one optimal parameter configuration, and the best configuration may differ according to the stages of the evolutionary process. Hence, in this research paper, a BRKGA with Q-Learning algorithm (BRKGA-QL) is proposed. The aim is to control the algorithm parameters during the evolutionary process using Reinforcement Learning, indicating the best configuration at each stage. In the experiments, BRKGA-QL was applied to the symmetric Traveling Salesman Problem, and the results show the efficiency and competitiveness of the proposed algorithm.
更多查看译文
关键词
Genetic Algorithm, Q-Learning, Reinforcement Learning, Parameter control
AI 理解论文
溯源树
样例
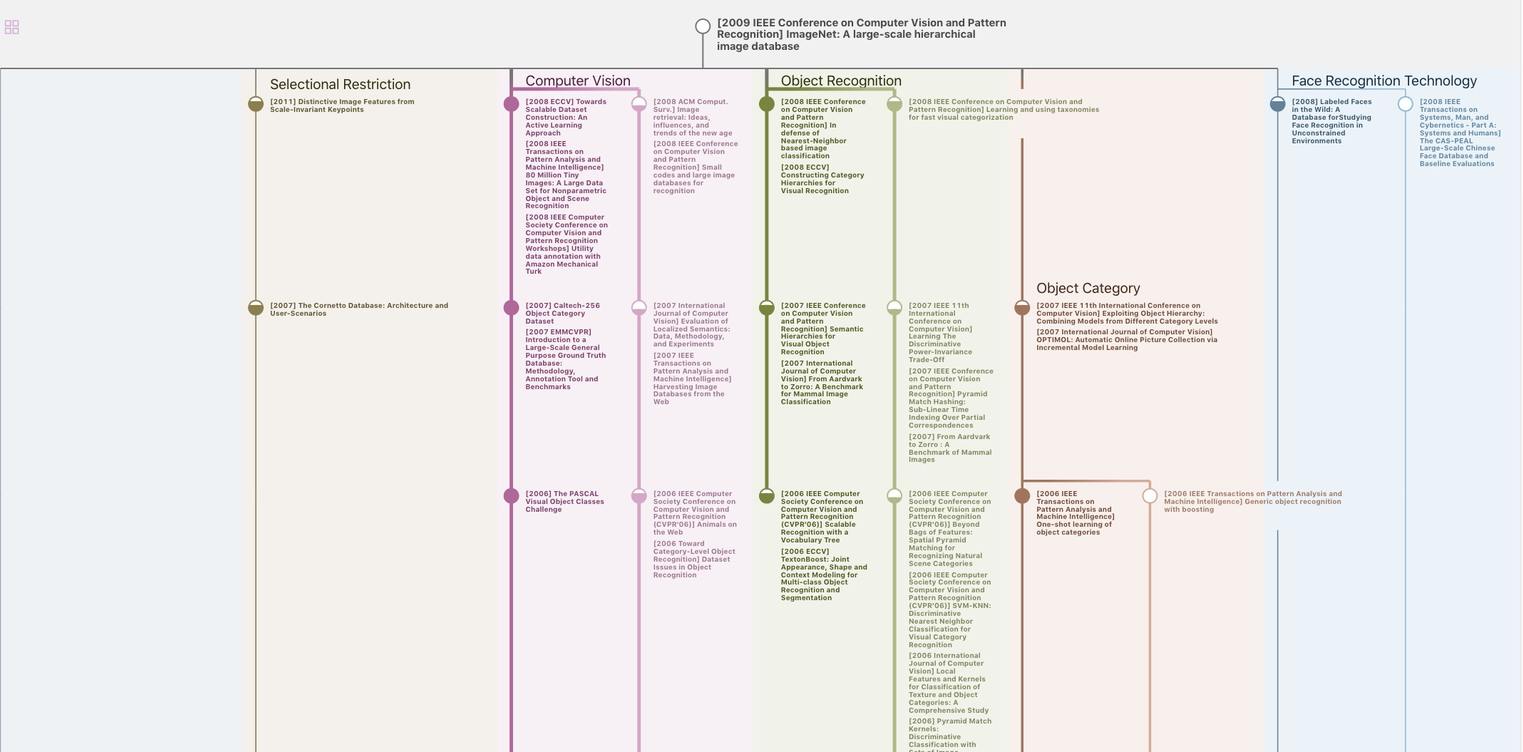
生成溯源树,研究论文发展脉络
Chat Paper
正在生成论文摘要