DP-LTOD: Differential Privacy Latent Trajectory Community Discovering Services over Location-Based Social Networks
IEEE Transactions on Services Computing(2021)
摘要
Community detection for Location-based Social Networks (LBSNs) has been received great attention mainly in the field of large-scale Wireless Communication Networks. In this paper, we present a Differential Privacy Latent Trajectory cOmmunity Discovering (DP-LTOD) scheme, which obfuscates original trajectory sequences into differential privacy-guaranteed trajectory sequences for trajectory privacy-preserving, and discovers latent trajectory communities through clustering the uploaded trajectory sequences. Different with traditional trajectory privacy-preserving methods, we first partition original trajectory sequence into different segments. Then, the suitable locations and segments are selected to constitute obfuscated trajectory sequence. Specifically, we formulate the trajectory obfuscation problem to select an optimal trajectory sequence which has the smallest difference with original trajectory sequence. In order to prevent privacy leakage, we add Laplace noise and exponential noise to the outputs during the stages of location obfuscation matrix generation and trajectory sequence function generation, respectively. Through formal privacy analysis, we prove that DP-LTOD scheme can guarantee ϵ-differential private. Moreover, we develop a trajectory clustering algorithm to classify the trajectories into different kinds of clusters according to semantic distance and geographical distance. Extensive experiments on two real-world datasets illustrate that our DP-LTOD scheme can not only discover latent trajectory communities, but also protect user privacy from leaking.
更多查看译文
关键词
Location-based social networks,communication detection,trajectory clustering,privacy preserving
AI 理解论文
溯源树
样例
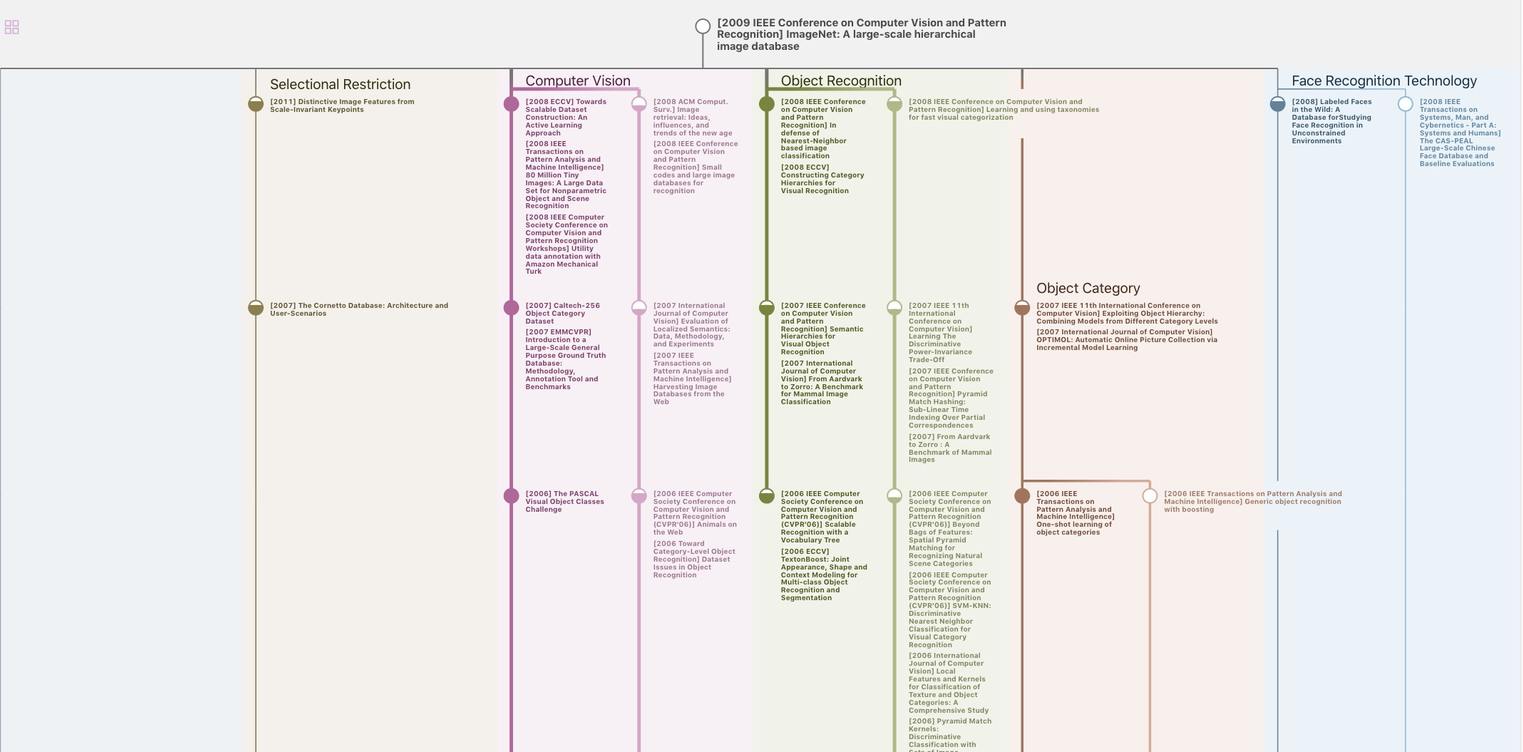
生成溯源树,研究论文发展脉络
Chat Paper
正在生成论文摘要