DnD - Dense Depth Estimation in Crowded Dynamic Indoor Scenes.
ICCV(2021)
摘要
We present a novel approach for estimating depth from a monocular camera as it moves through complex and crowded indoor environments, e.g., a department store or a metro station. Our approach predicts absolute scale depth maps over the entire scene consisting of a static background and multiple moving people, by training on dynamic scenes. Since it is difficult to collect dense depth maps from crowded indoor environments, we design our training framework without requiring depths produced from depth sensing devices. Our network leverages RGB images and sparse depth maps generated from traditional 3D reconstruction methods to estimate dense depth maps. We use two constraints to handle depth for non-rigidly moving people without tracking their motion explicitly. We demonstrate that our approach offers consistent improvements over recent depth estimation methods on the NAVERLABS dataset, which includes complex and crowded scenes.
更多查看译文
关键词
dense depth estimation
AI 理解论文
溯源树
样例
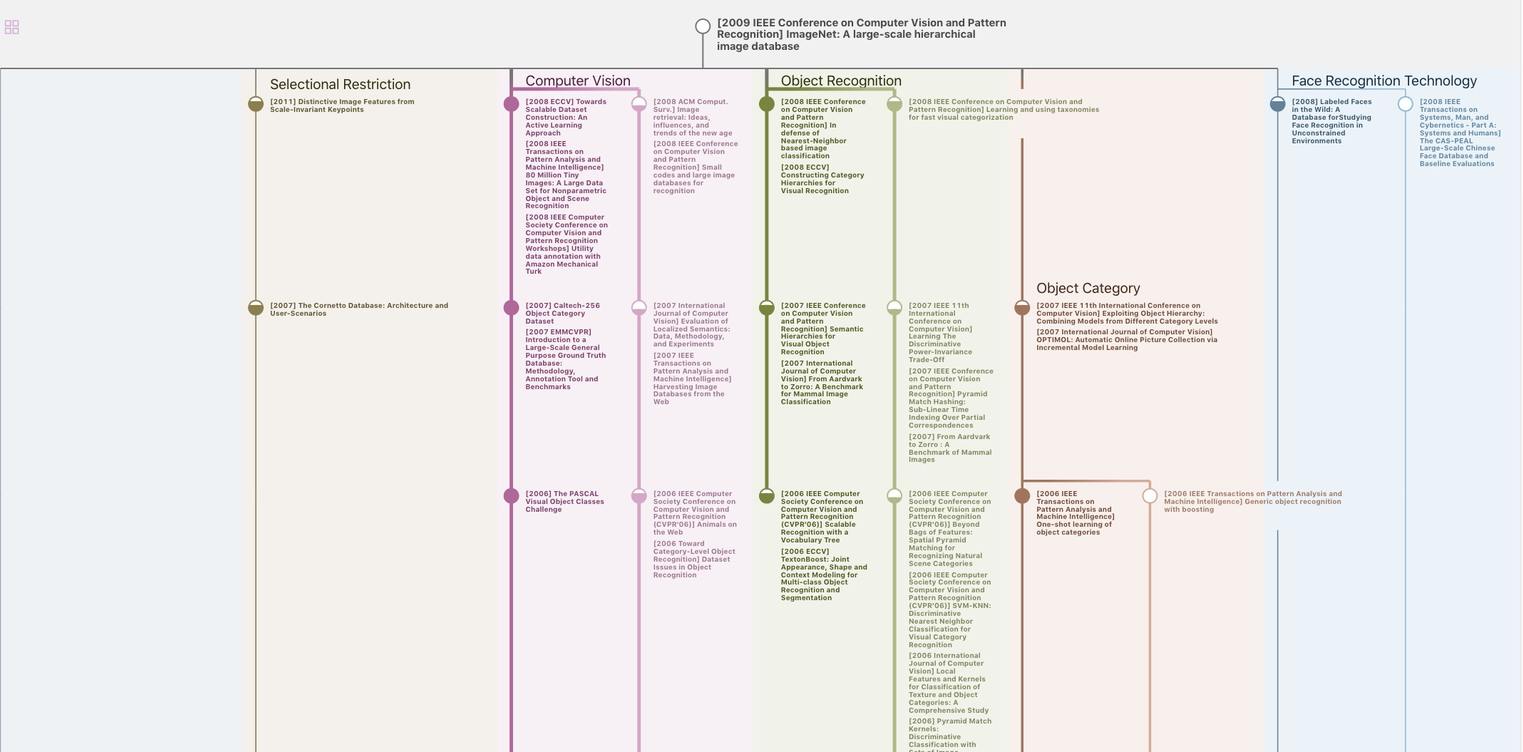
生成溯源树,研究论文发展脉络
Chat Paper
正在生成论文摘要