HandFoldingNet - A 3D Hand Pose Estimation Network Using Multiscale-Feature Guided Folding of a 2D Hand Skeleton.
ICCV(2021)
摘要
With increasing applications of 3D hand pose estimation in various human-computer interaction applications, convolution neural networks (CNNs) based estimation models have been actively explored. However, the existing models require complex architectures or redundant computational resources to trade with the acceptable accuracy. To tackle this limitation, this paper proposes HandFoldingNet, an accurate and efficient hand pose estimator that regresses the hand joint locations from the normalized 3D hand point cloud input. The proposed model utilizes a folding-based decoder that folds a given 2D hand skeleton into the corresponding joint coordinates. For higher estimation accuracy, folding is guided by multi-scale features, which include both global and joint-wise local features. Experimental results show that the proposed model outperforms the existing methods on three hand pose benchmark datasets with the lowest model parameter requirement. Code is available at https://github.com/cwc1260/HandFold.
更多查看译文
关键词
handfoldingnet,handfoldingnet,3d,multiscale-feature
AI 理解论文
溯源树
样例
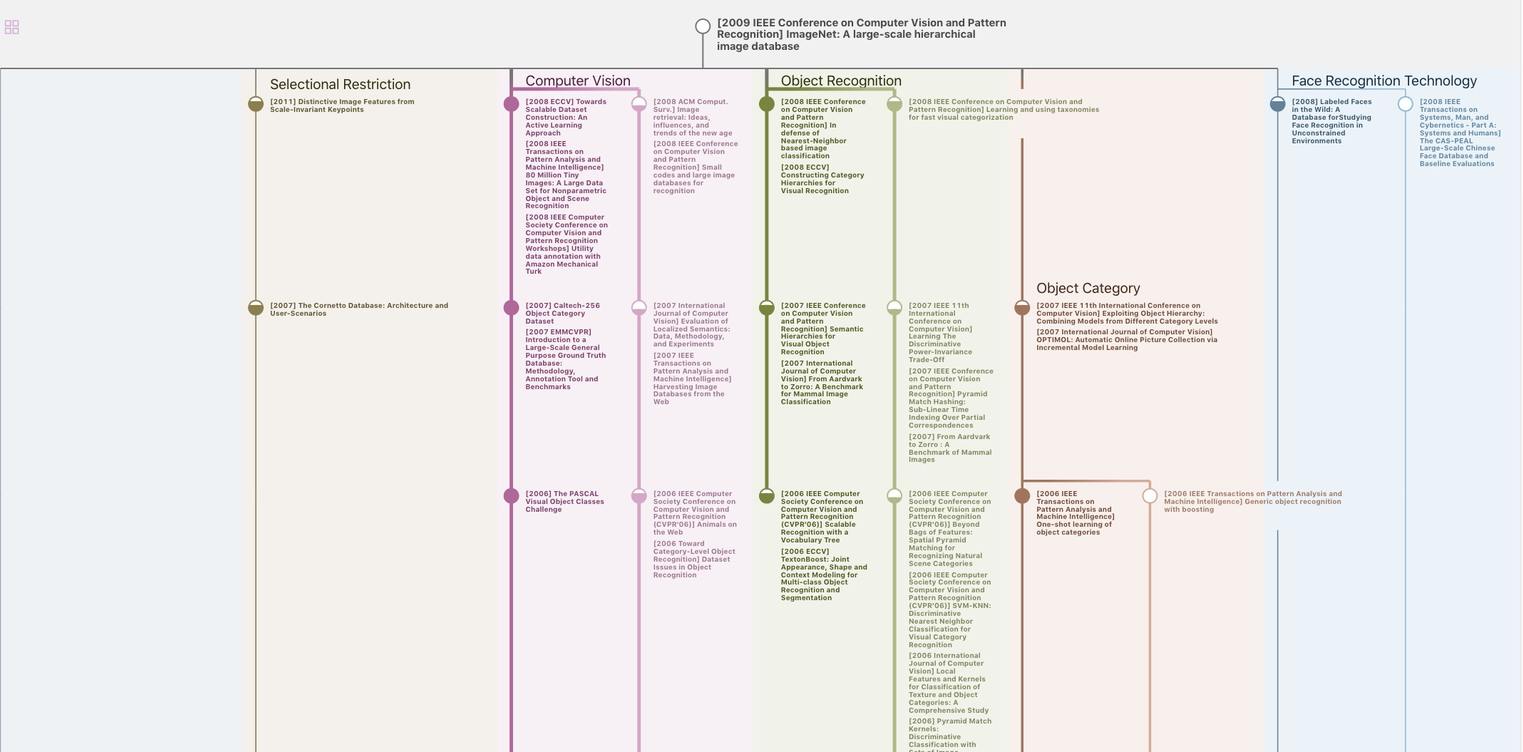
生成溯源树,研究论文发展脉络
Chat Paper
正在生成论文摘要