Towards Rule-ranking Based Fuzzy Rule Interpolation
2021 IEEE International Conference on Fuzzy Systems (FUZZ-IEEE)(2021)
摘要
Recently proposed methods of TSK inference extension (TSK+) and K closest rules based TSK (KCR) are able to potentially perform fuzzy rule-based inference with a sparse rule base, for regression problems. However, in certain real-world applications, observations may be rather far away from any rules that may be fired in order to implement the required regression and hence, these state-of-the-art techniques may not work satisfactorily. To investigate an alternative to address such practical problems, this paper presents a novel fuzzy rule interpolation (FRI) approach. It works based on integrating feature selection and feature aggregation to attain a rule-ranking list within the given sparse rule base. The process of acquiring an ordered rule list is accomplished offline, with an ordered rule base ready for use prior to the arrival of any observation online. It boosts the computational efficiency of closest rule selection during the FRI process. Initial experimental results demonstrate that the proposed approach is able to address the problems that TSK+ and KCR fail to do while having a similar time efficiency to them. Further, only two nearest rules to the observation are required to derive interpolated results, thereby significantly improving the automation level of the interpolative reasoning system.
更多查看译文
关键词
feature aggregation,rule-ranking list,sparse rule base,ordered rule list,ordered rule base,closest rule selection,nearest rules,TSK+,fuzzy rule-based inference,regression problems,fuzzy rule interpolation,feature selection,rule-ranking based fuzzy rule interpolation,TSK inference extension,K closest rules based TSK,computational efficiency,interpolative reasoning system
AI 理解论文
溯源树
样例
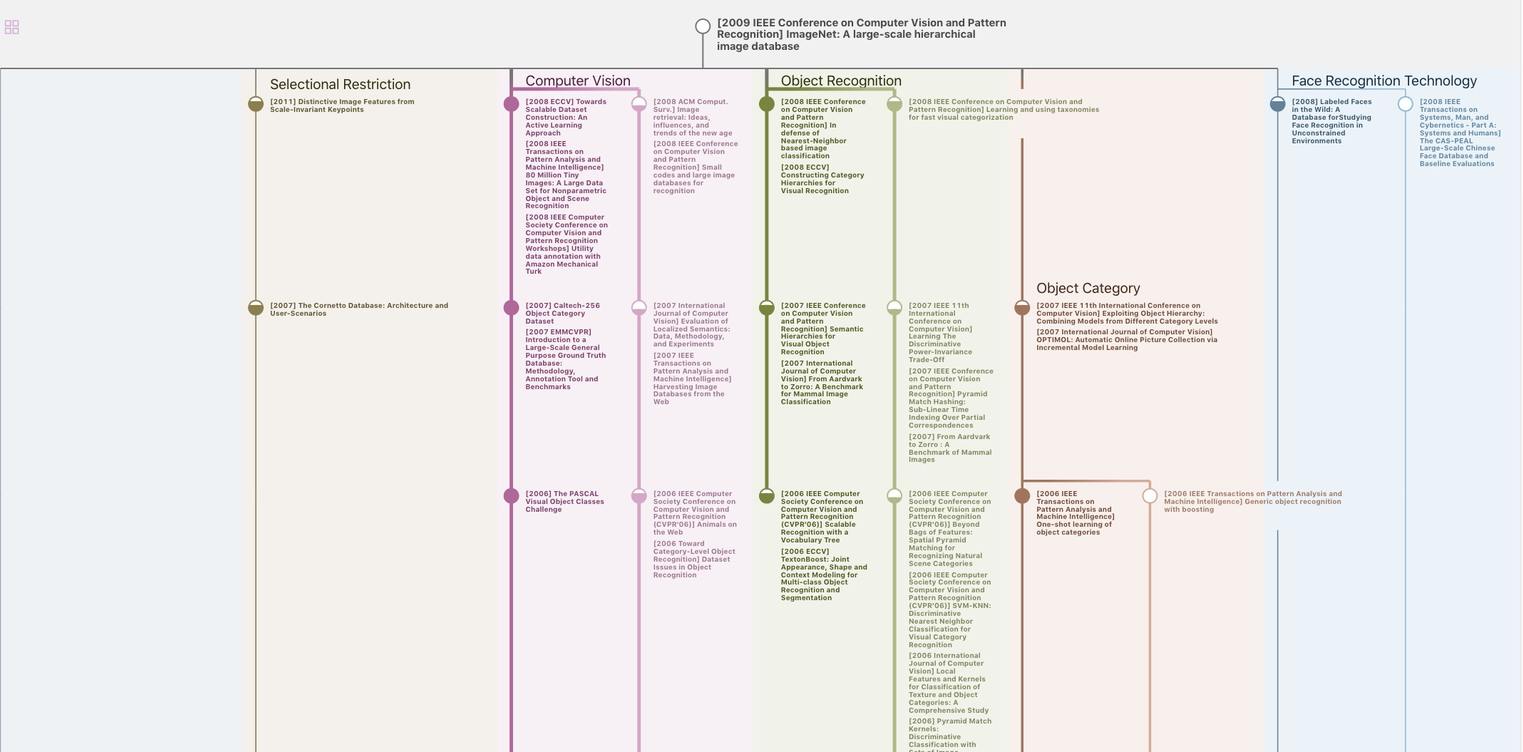
生成溯源树,研究论文发展脉络
Chat Paper
正在生成论文摘要