A novel Data-driven fuzzy aggregation method for Takagi-Sugeno-Kang fuzzy Neural network system using ensemble learning
2021 IEEE International Conference on Fuzzy Systems (FUZZ-IEEE)(2021)
摘要
Fuzzy aggregation operators commonly rely on expert information to solve multi-attribute decision-making problems. Such expert input may contain human bias or may sometimes be unavailable. This paper proposes a novel data-driven fuzzy aggregation method for Takagi-Sugeno-Kang fuzzy neural networks (TSKFNN) based upon the ensemble learning algorithm, AdaBoost. The objective of this research is to investigate whether ensemble learning is an effective tool for data-driven fuzzy aggregation. Our hypothesis is that ensemble learning would improve model performance and explainability. In this study, AdaBoost is applied to get a weighted combination of fuzzy rules in the TSKFNN and calculate the weighted average of these fuzzy rules to generate model predictions. Existing fuzzy aggregation operators are used as benchmarks to evaluate the proposed model. The results show that the proposed model is capable of yielding higher accuracy and greater interpretability than the existing methods through the identification of the most significant fuzzy rules used in the decision-making process.
更多查看译文
关键词
Fuzzy aggregation method,AdaBoost,TSK Fuzzy-Neural network,multi-attribute decision-making problems,Ensemble Learning
AI 理解论文
溯源树
样例
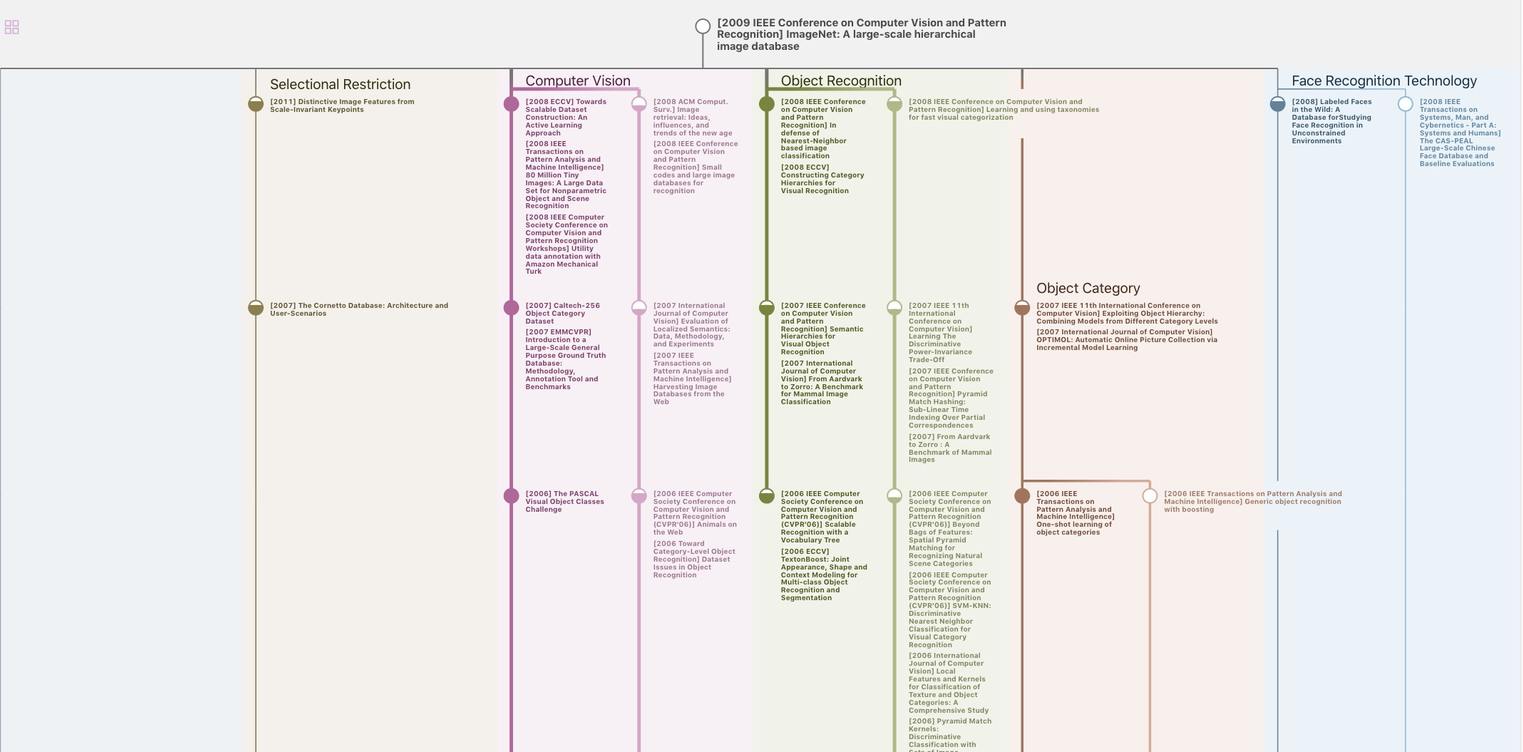
生成溯源树,研究论文发展脉络
Chat Paper
正在生成论文摘要