Training Sparse Fuzzy Classifiers Using Metaheuristic Optimization
IEEE CIS INTERNATIONAL CONFERENCE ON FUZZY SYSTEMS 2021 (FUZZ-IEEE)(2021)
摘要
This paper proposes a novel classification model called sparse fuzzy classifier (SFC), which uses a sparse Takagi-Sugeno fuzzy system to classify data. This system is considered with Gaussian fuzzy sets in the antecedents and first-order polynomials in the consequents of fuzzy rules. The input fuzzy sets are determined using a metaheuristic optimization method (particle swarm optimization, genetic algorithm, simulated annealing, or pattern search). The output polynomials are obtained by a sparse regression represented by the elastic net. A quality criterion based on the confusion value is used to select the best model. The experiments showed that the proposed method could reduce the confusion and simplify fuzzy models by zeroing some of the polynomial coefficients.
更多查看译文
关键词
classification, fuzzy system, sparse regression, metaheuristic optimization, particle swarm optimization, genetic algorithm
AI 理解论文
溯源树
样例
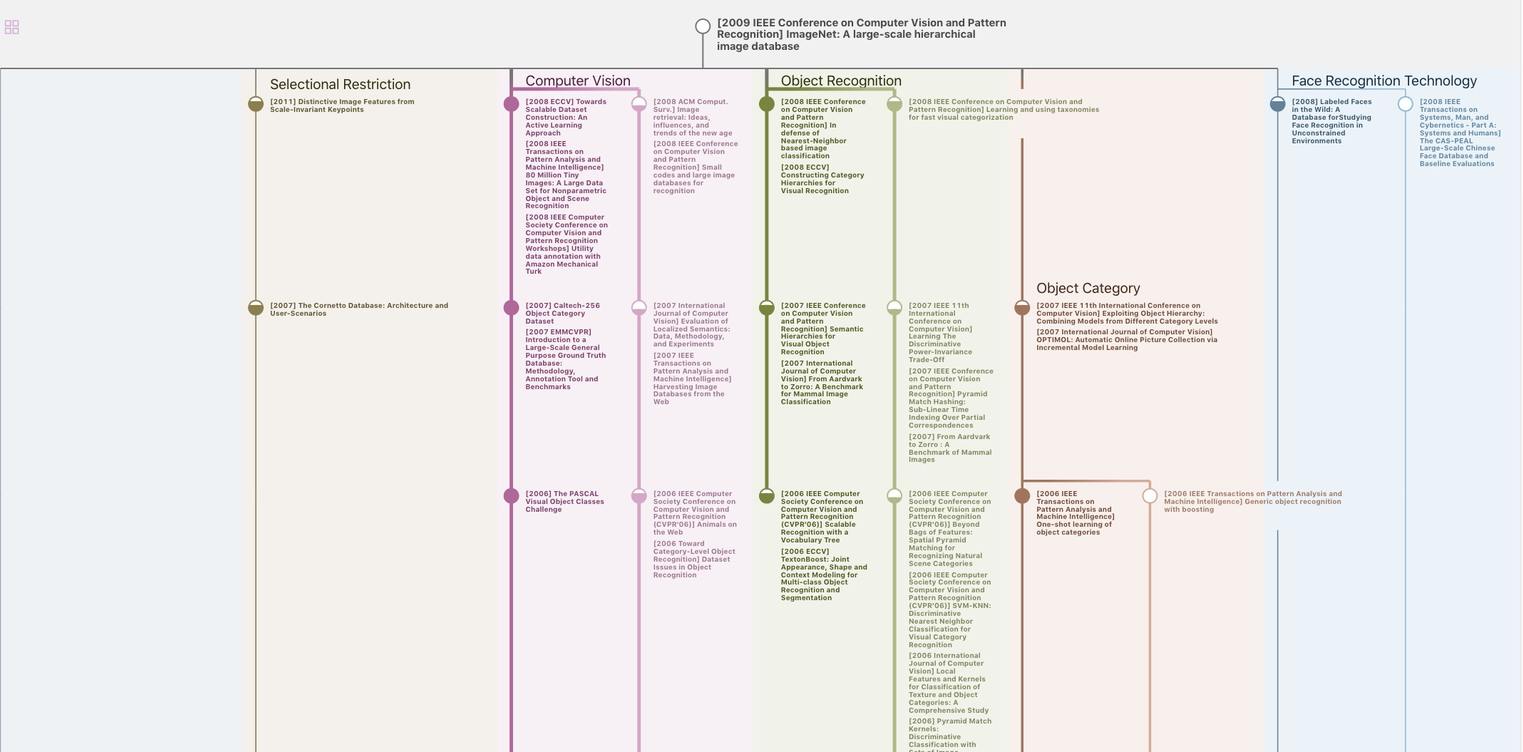
生成溯源树,研究论文发展脉络
Chat Paper
正在生成论文摘要