Adaptive Synapse Arrangement In Cortical Learning Algorithm
JOURNAL OF ADVANCED COMPUTATIONAL INTELLIGENCE AND INTELLIGENT INFORMATICS(2021)
摘要
The cortical learning algorithm (CLA) is a time-series data prediction method that is designed based on the human neocortex. The CLA has multiple columns that are associated with the input data bits by synapses. The input data is then converted into an internal column representation based on the synapse relation. Because the synapse relation between the columns and input data bits is fixed during the entire prediction process in the conventional CLA, it cannot adapt to input data biases. Consequently, columns not used for internal representations arise, resulting in a low prediction accuracy in the conventional CLA. To improve the prediction accuracy of the CLA, we propose a CLA that self-adaptively arranges the column synapses according to the input data tendencies and verify its effectiveness with several artificial time-series data and real-world electricity load prediction data from New York City. Experimental results show that the proposed CLA achieves higher prediction accuracy than the conventional CLA and LSTMs with different network optimization algorithms by arranging column synapses according to the input data tendency.
更多查看译文
关键词
cortical learning algorithm, time-series data prediction, predictor adaptation
AI 理解论文
溯源树
样例
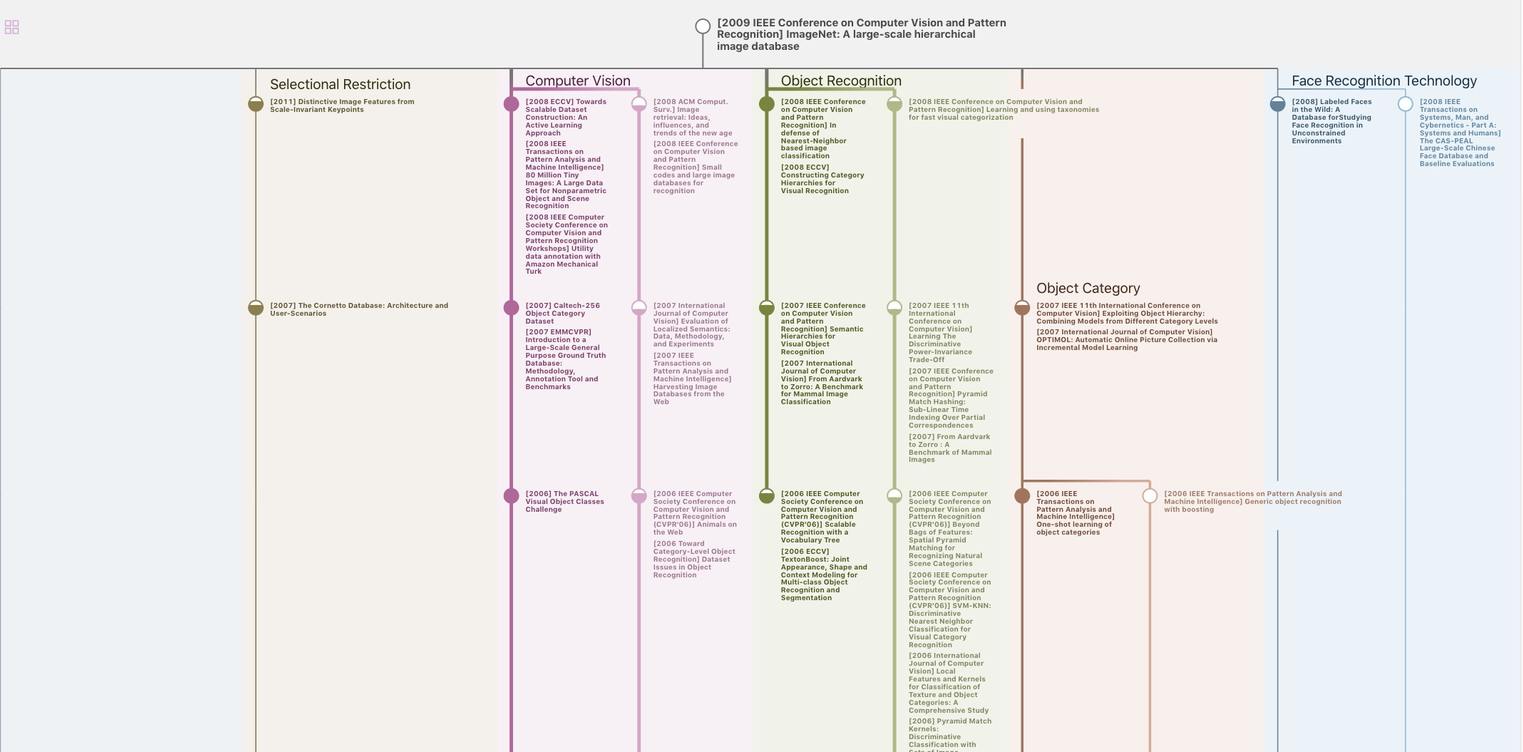
生成溯源树,研究论文发展脉络
Chat Paper
正在生成论文摘要