Boosting the Generalization Capability in Cross-Domain Few-shot Learning via Noise-enhanced Supervised Autoencoder.
ICCV(2021)
摘要
State of the art (SOTA) few-shot learning (FSL) methods suffer significant performance drop in the presence of domain differences between source and target datasets. The strong discrimination ability on the source dataset does not necessarily translate to high classification accuracy on the target dataset. In this work, we address this cross-domain few-shot learning (CDFSL) problem by boosting the generalization capability of the model. Specifically, we teach the model to capture broader variations of the feature distributions with a novel noise-enhanced supervised autoencoder (NSAE). NSAE trains the model by jointly reconstructing inputs and predicting the labels of inputs as well as their reconstructed pairs. Theoretical analysis based on intra-class correlation (ICC) shows that the feature embeddings learned from NSAE have stronger discrimination and generalization abilities in the target domain. We also take advantage of NSAE structure and propose a two-step fine-tuning procedure that achieves better adaption and improves classification performance in the target domain. Extensive experiments and ablation studies are conducted to demonstrate the effectiveness of the proposed method. Experimental results show that our proposed method consistently outperforms SOTA methods under various conditions.
更多查看译文
关键词
Transfer/Low-shot/Semi/Unsupervised Learning,Optimization and learning methods,Recognition and classification,Representation learning
AI 理解论文
溯源树
样例
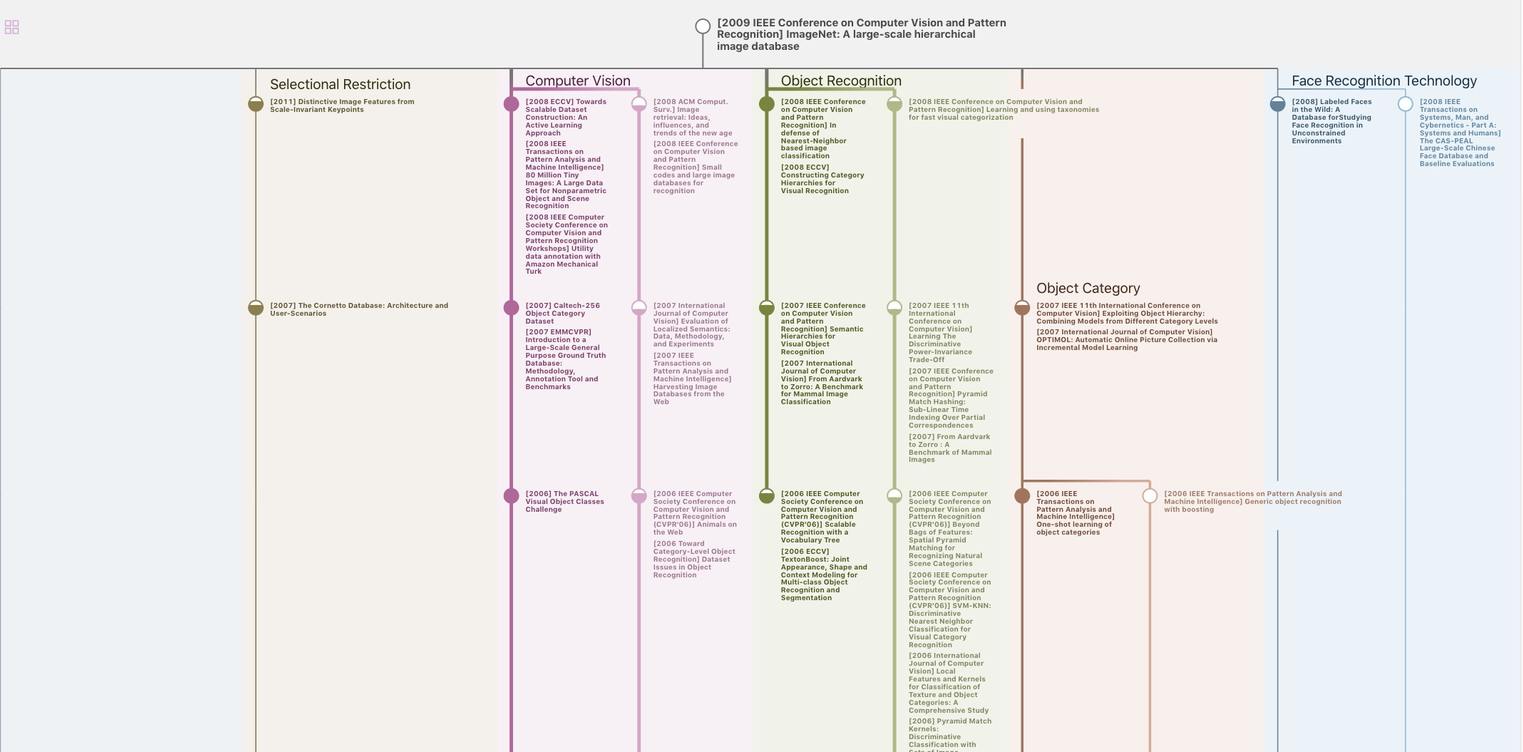
生成溯源树,研究论文发展脉络
Chat Paper
正在生成论文摘要