Convolutional Neural Networks For Challenges In Automated Nuclide Identification
SENSORS(2021)
摘要
Improvements in Radio-Isotope IDentification (RIID) algorithms have seen a resurgence in interest with the increased accessibility of machine learning models. Convolutional Neural Network (CNN)-based models have been developed to identify arbitrary mixtures of unstable nuclides from gamma spectra. In service of this, methods for the simulation and pre-processing of training data were also developed. The implementation of 1D multi-class, multi-label CNNs demonstrated good generalisation to real spectra with poor statistics and significant gain shifts. It is also shown that even basic CNN architectures prove reliable for RIID under the challenging conditions of heavy shielding and close source geometries, and may be extended to generalised solutions for pragmatic RIID.
更多查看译文
关键词
radio-isotope identification, convolutional neural network, gamma spectrometry, simulations, GEANT, nuclear applications
AI 理解论文
溯源树
样例
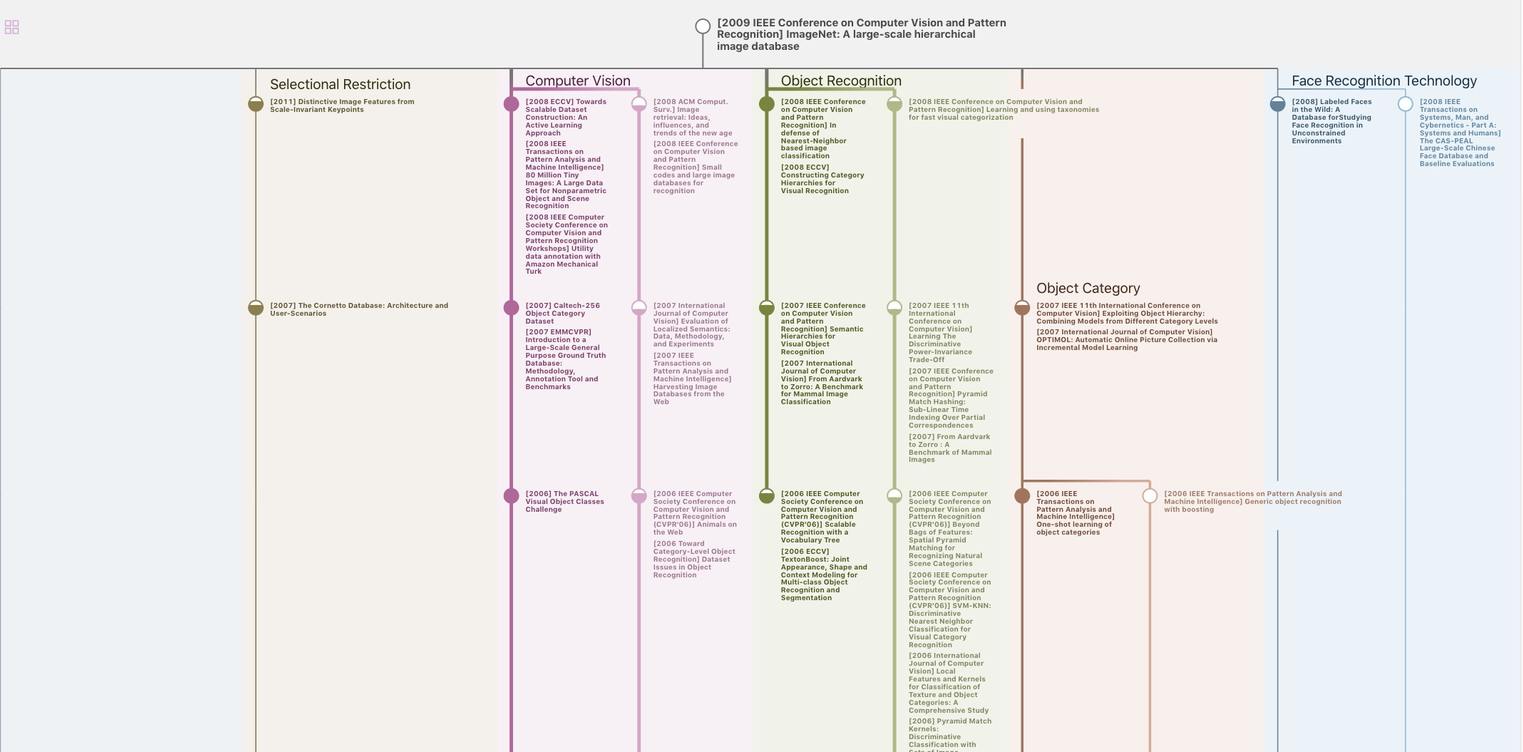
生成溯源树,研究论文发展脉络
Chat Paper
正在生成论文摘要