Prediction Of Egfr Mutation Status Based On F-18-Fdg Pet/Ct Imaging Using Deep Learning-Based Model In Lung Adenocarcinoma
FRONTIERS IN ONCOLOGY(2021)
摘要
Objective The purpose of this study was to develop a deep learning-based system to automatically predict epidermal growth factor receptor (EGFR) mutant lung adenocarcinoma in F-18-fluorodeoxyglucose (FDG) positron emission tomography/computed tomography (PET/CT).& nbsp;Methods Three hundred and one lung adenocarcinoma patients with EGFR mutation status were enrolled in this study. Two deep learning models (SECT and SEPET) were developed with Squeeze-and-Excitation Residual Network (SE-ResNet) module for the prediction of EGFR mutation with CT and PET images, respectively. The deep learning models were trained with a training data set of 198 patients and tested with a testing data set of 103 patients. Stacked generalization was used to integrate the results of SECT and SEPET.& nbsp;Results The AUCs of the SECT and SEPET were 0.72 (95% CI, 0.62-0.80) and 0.74 (95% CI, 0.65-0.82) in the testing data set, respectively. After integrating SECT and SEPET with stacked generalization, the AUC was further improved to 0.84 (95% CI, 0.75-0.90), significantly higher than SECT (p < 0.05).& nbsp;Conclusion The stacking model based on F-18-FDG PET/CT images is capable to predict EGFR mutation status of patients with lung adenocarcinoma automatically and non-invasively. The proposed model in this study showed the potential to help clinicians identify suitable advanced patients with lung adenocarcinoma for EGFR-targeted therapy.
更多查看译文
关键词
adenocarcinoma of lung, fluorodeoxyglucose F18, positron emission tomography computed tomography, deep learning, epidermal growth factor receptor
AI 理解论文
溯源树
样例
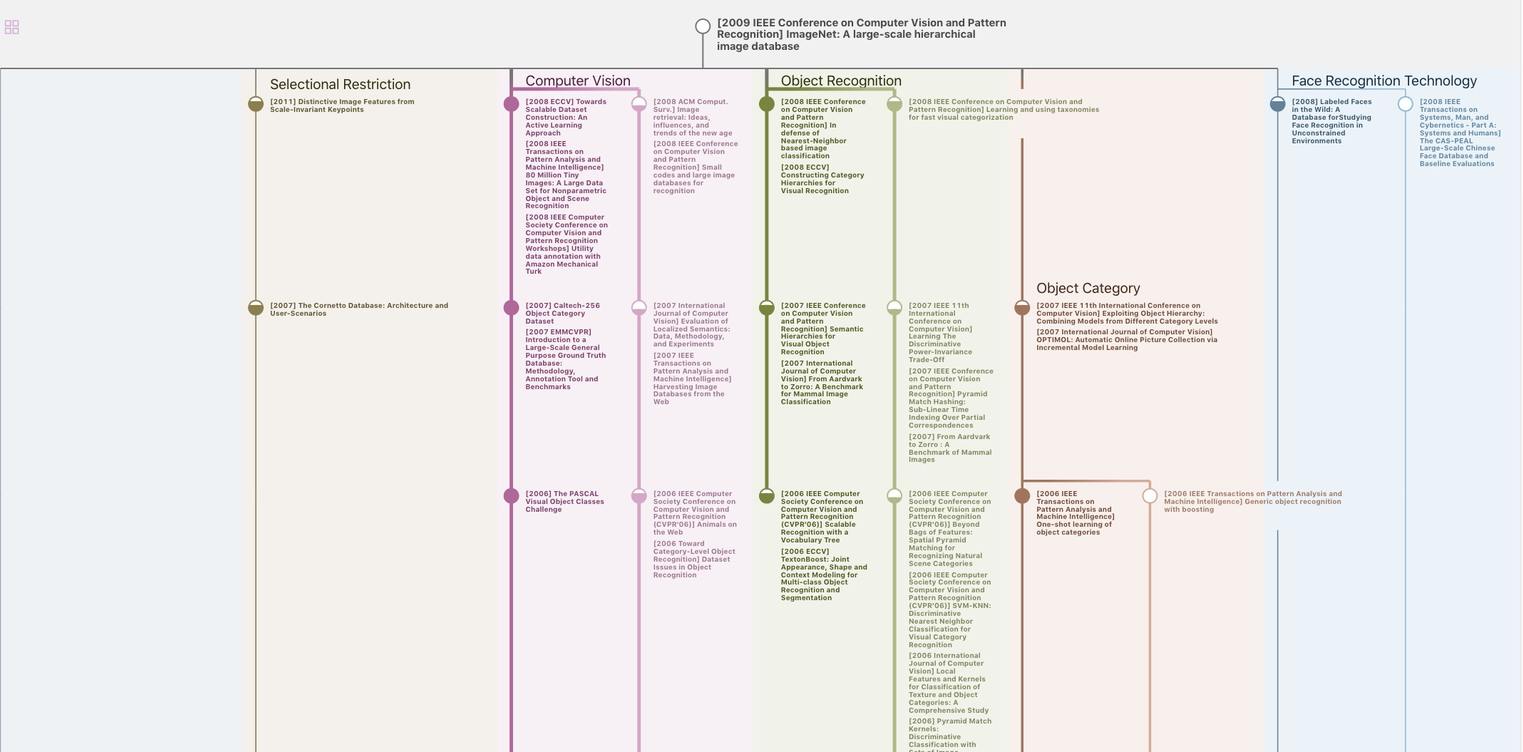
生成溯源树,研究论文发展脉络
Chat Paper
正在生成论文摘要