Deep Joint Source-Channel Coding for Transmission of Correlated Sources over AWGN Channels
IEEE INTERNATIONAL CONFERENCE ON COMMUNICATIONS (ICC 2021)(2021)
摘要
We revisit the joint source-channel coding (JSCC) problem of transmitting correlated sources over the additive white Gaussian noise channel using deep learning methods. Specifically, we consider the design of JSCC schemes for transmitting multivariate Gaussian sources, and Gauss-Markov processes over noisy channels with bandwidth (BW) compression and low delay. We show that encoding and decoding schemes represented by deep neural networks can be optimized jointly to obtain good JSCC schemes. Specifically, we adopt sinusoidal representation networks (SIRENs) for the transmission of multivariate Gaussian sources. The new architecture not only provides similar performance as the state-of-the-art (SOTA) with higher flexibility, but also results in interpretable encoder mappings. For the transmission of Gauss-Markov sources, recurrent neural networks (RNNs) are implemented to extract temporal information without resorting to explicit decorrelation prior to transmission. Experimental results show improved performance compared with traditional schemes.
更多查看译文
关键词
bandwidth compression,encoding schemes,decoding schemes,deep neural networks,good JSCC schemes,sinusoidal representation networks,multivariate Gaussian sources,Gauss-Markov sources,traditional schemes,deep joint source-channel coding,correlated sources,AWGN channels,joint source-channel coding problem,additive white Gaussian noise channel,deep learning methods,noisy channels
AI 理解论文
溯源树
样例
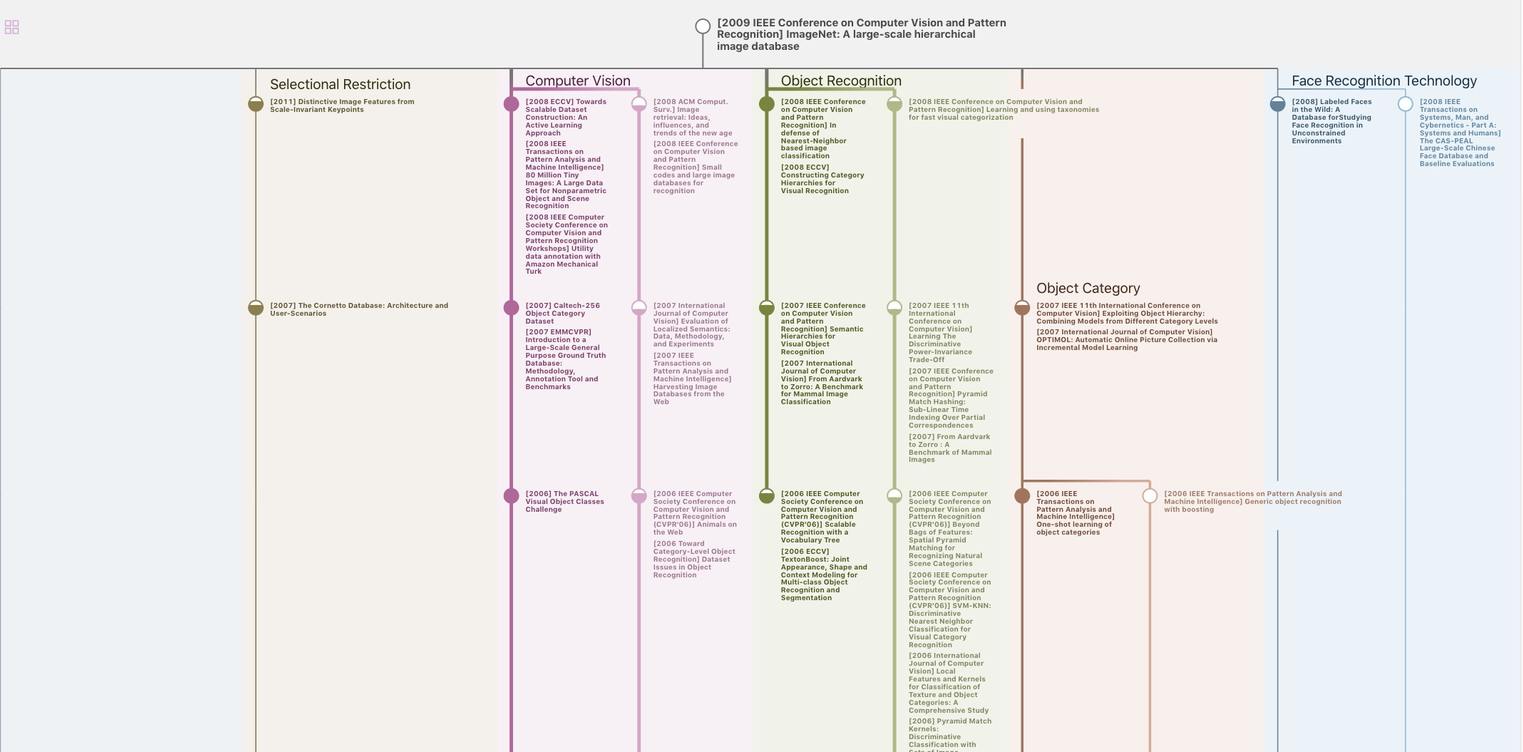
生成溯源树,研究论文发展脉络
Chat Paper
正在生成论文摘要