V2V Cooperative Sensing using Reinforcement Learning with Action Branching
IEEE INTERNATIONAL CONFERENCE ON COMMUNICATIONS (ICC 2021)(2021)
摘要
Cooperative perception plays a vital role in extending a vehicle's sensing range beyond its line-of-sight. However, exchanging raw sensory data under limited communication resources is infeasible. Towards enabling an efficient cooperative perception, vehicles need to address fundamental questions such as: what sensory data needs to be shared? at which resolution? with which vehicles? In this view, this paper proposes a reinforcement learning (RL)-based vehicular association, resource block (RB) allocation, and content selection of cooperative perception messages by utilizing a quad-tree-based point cloud compression mechanism. Simulation results show the ability of the RL agents to efficiently learn the vehicles' association, RB allocation and message content selection that maximizes the fulfillment of the vehicles in terms of the received sensory information.
更多查看译文
关键词
V2V, cooperative perception, reinforcement learning, quadtree
AI 理解论文
溯源树
样例
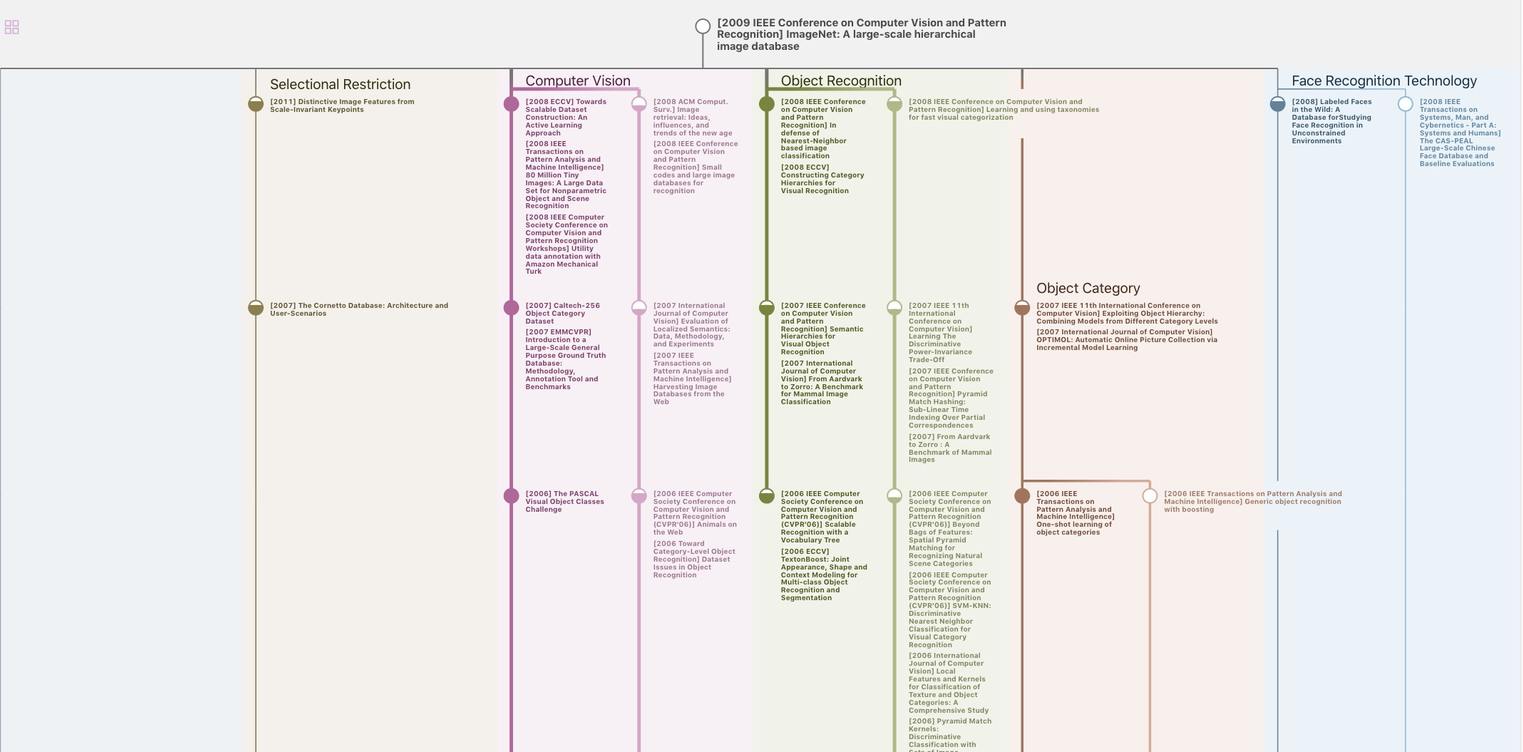
生成溯源树,研究论文发展脉络
Chat Paper
正在生成论文摘要