Online Minimax Multiobjective Optimization: Multicalibeating and Other Applications
arxiv(2022)
摘要
We introduce a simple but general online learning framework in which a learner plays against an adversary in a vector-valued game that changes every round. Even though the learner's objective is not convex-concave (and so the minimax theorem does not apply), we give a simple algorithm that can compete with the setting in which the adversary must announce their action first, with optimally diminishing regret. We demonstrate the power of our framework by using it to (re)derive optimal bounds and efficient algorithms across a variety of domains, ranging from multicalibration to a large set of no regret algorithms, to a variant of Blackwell's approachability theorem for polytopes with fast convergence rates. As a new application, we show how to ``(multi)calibeat'' an arbitrary collection of forecasters -- achieving an exponentially improved dependence on the number of models we are competing against, compared to prior work.
更多查看译文
关键词
multicalibeating,optimization
AI 理解论文
溯源树
样例
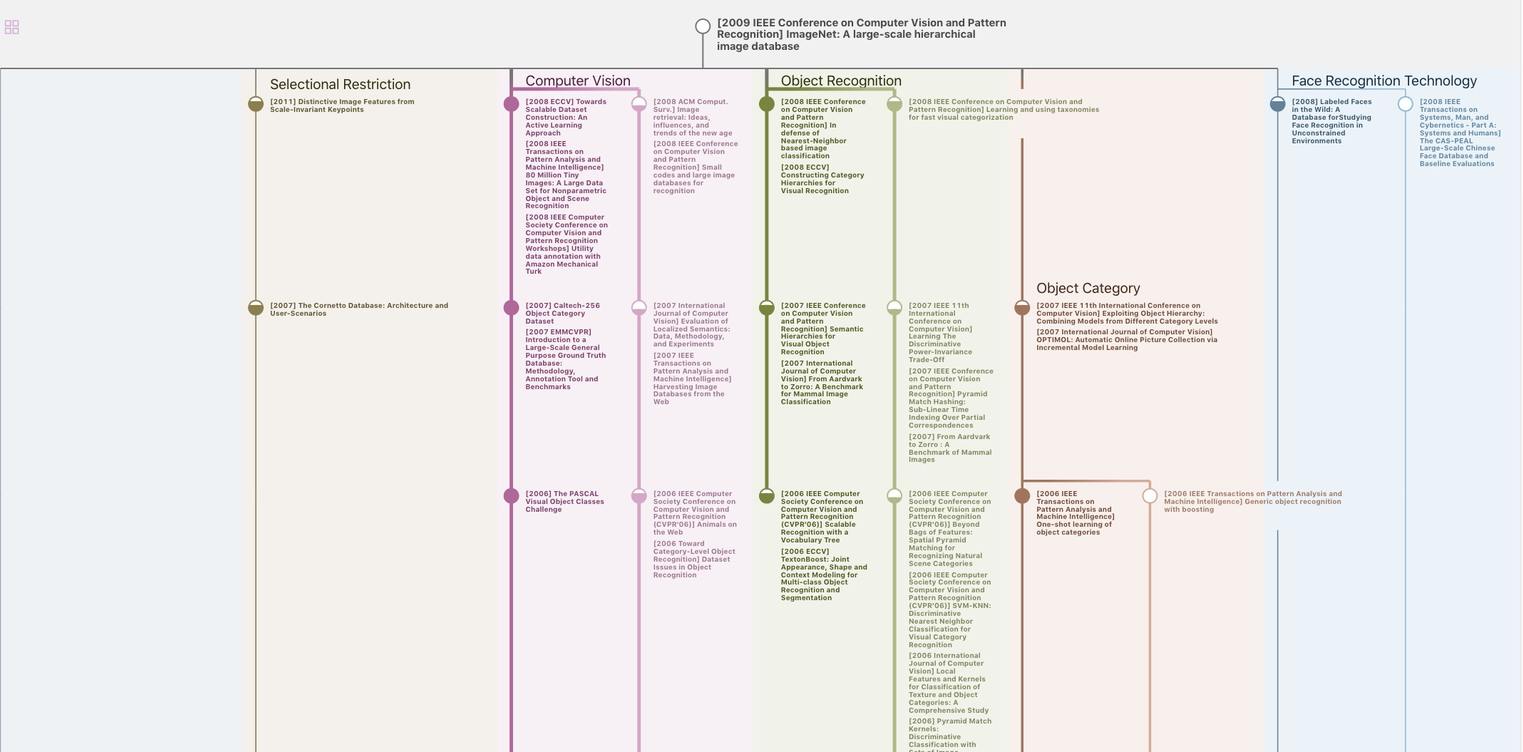
生成溯源树,研究论文发展脉络
Chat Paper
正在生成论文摘要