Self-Learning Sparse PCA for Multimode Process Monitoring
IEEE Transactions on Industrial Informatics(2023)
摘要
This article proposes a novel sparse principal component analysis algorithm with self-learning ability for multimode process monitoring, where the successive modes are learned in a sequential fashion. Different from traditional multimode monitoring methods, a small set of data are collected when a novel mode arrives. The proposed method remembers the learned knowledge by selectively slowing down the changes of parameters important for the previous modes, where the importance measure is estimated by synaptic intelligence. The sufficient condition of fault detectability is proved to provide a comprehensive understanding of the proposed method. Besides, the computation and storage resources are saved in the long run, because it is not necessary to retrain the model from scratch frequently and data are discarded once they have been learned. More importantly, the model furnishes excellent interpretability and the catastrophic forgetting problem is further alleviated owing to the sparsity of parameters. In addition, the hyperparameters are discussed to understand the proposed method comprehensively and the computational complexity is analyzed. Compared with several state-of-the-art approaches, a numerical case, and a practical pulverizing system are adopted to illustrate the effectiveness of the proposed algorithm.
更多查看译文
关键词
Multimode process monitoring,self-learning,sparse principal component analysis,synaptic intelligence
AI 理解论文
溯源树
样例
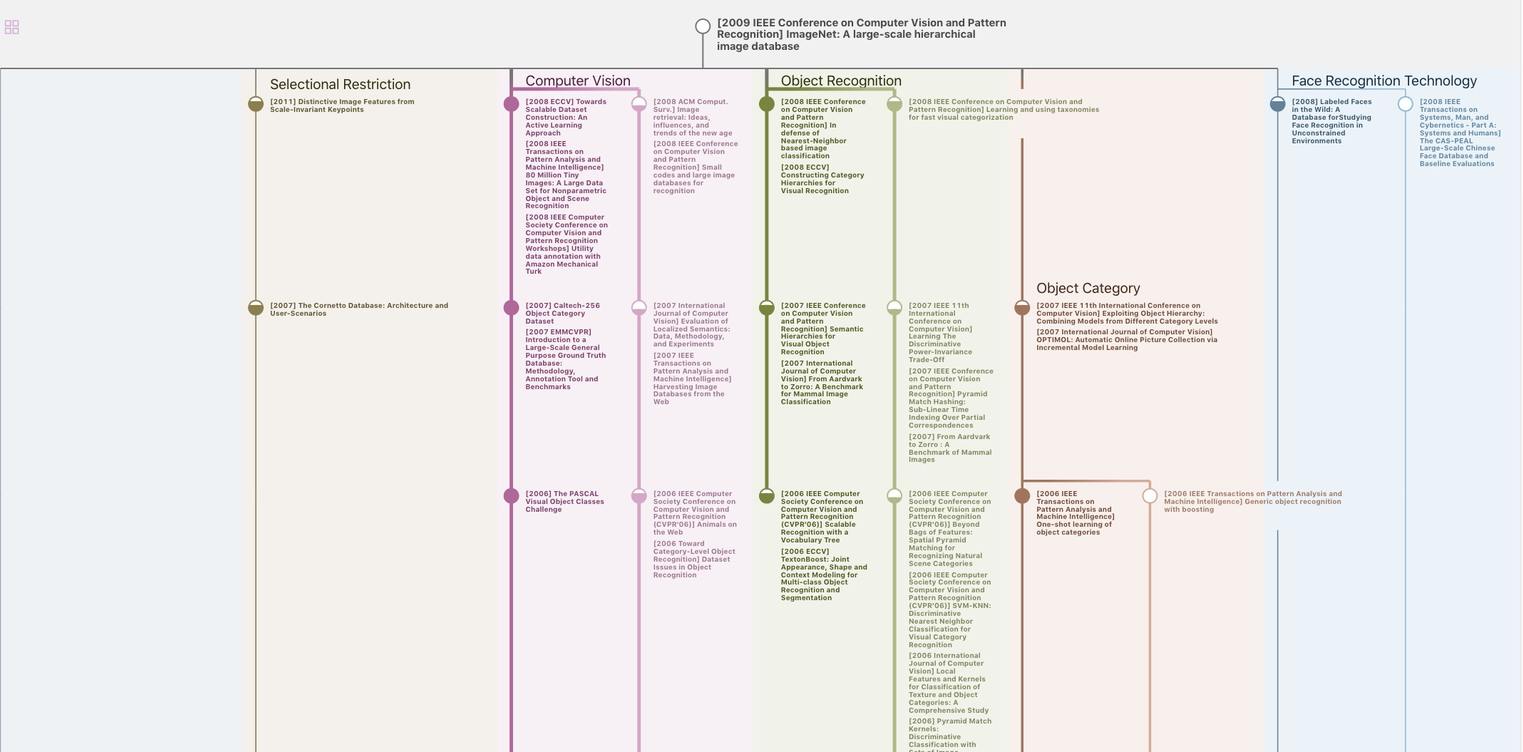
生成溯源树,研究论文发展脉络
Chat Paper
正在生成论文摘要