Controllable Summarization with Constrained Markov Decision Process
TRANSACTIONS OF THE ASSOCIATION FOR COMPUTATIONAL LINGUISTICS(2021)
摘要
We study controllable text summarization, which allows users to gain control on a particular attribute (e.g., length limit) of the generated summaries. In this work, we propose a novel training framework based on Constrained Markov Decision Process (CMDP), which conveniently includes a reward function along with a set of constraints, to facilitate better summarization control. The reward function encourages the generation to resemble the human-written reference, while the constraints are used to explicitly prevent the generated summaries from violating user-imposed requirements. Our framework can be applied to control important attributes of summarization, including length, covered entities, and abstractiveness, as we devise specific constraints for each of these aspects. Extensive experiments on popular benchmarks show that ourCMDPframework helps generate informative summaries while complying with a given attribute's requirement.(1)
更多查看译文
关键词
summarization,constrained
AI 理解论文
溯源树
样例
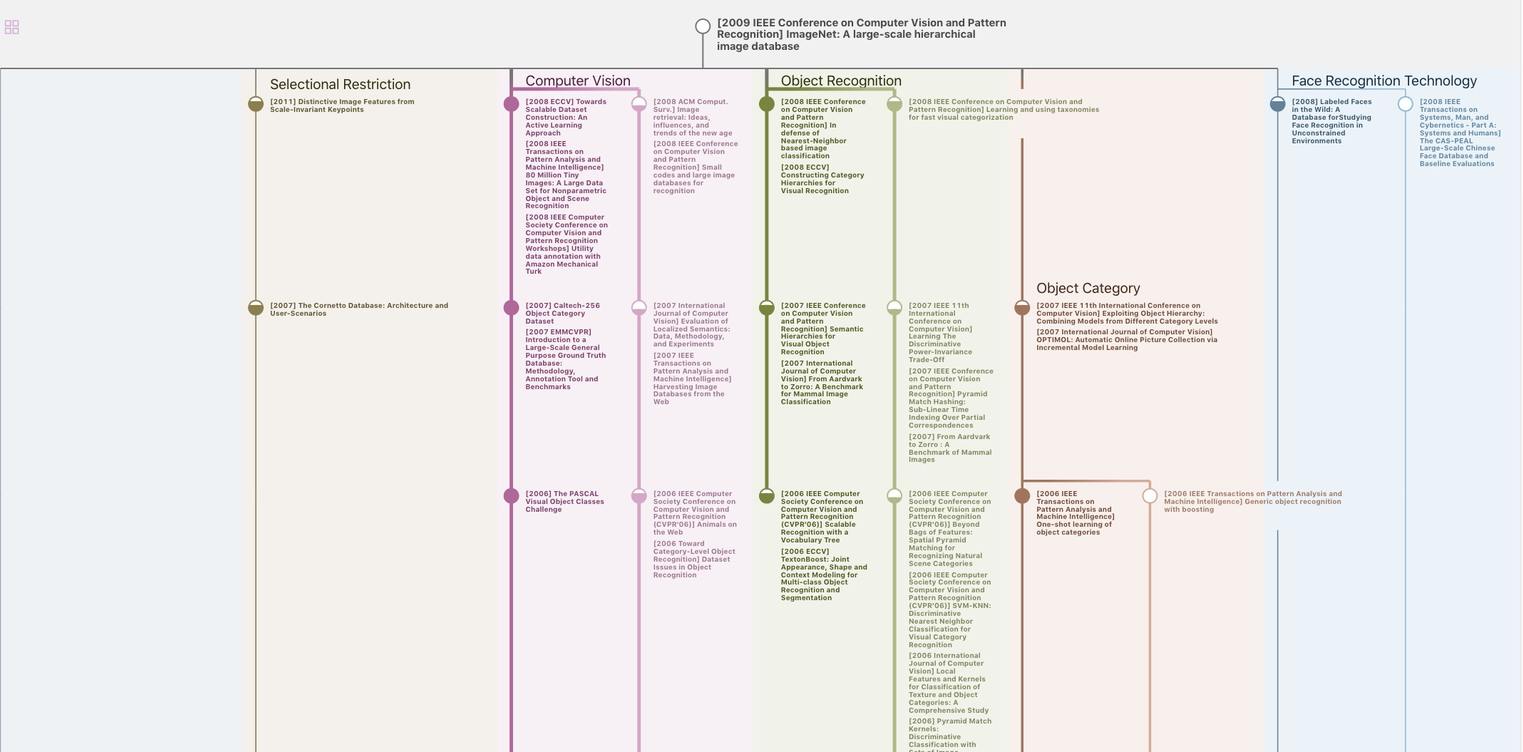
生成溯源树,研究论文发展脉络
Chat Paper
正在生成论文摘要