Semantic Tracklets: An Object-Centric Representation for Visual Multi-Agent Reinforcement Learning
2021 IEEE/RSJ INTERNATIONAL CONFERENCE ON INTELLIGENT ROBOTS AND SYSTEMS (IROS)(2021)
摘要
Solving complex real-world tasks, e.g., autonomous fleet control, often involves a coordinated team of multiple agents which learn strategies from visual inputs via reinforcement learning. Many existing multi-agent reinforcement learning (MARL) algorithms however don't scale to environments where agents operate on visual inputs. To address this issue, algorithmically, recent works have focused on non-stationarity and exploration. In contrast, we study whether scalability can also be achieved via a disentangled representation. For this, we explicitly construct an object-centric intermediate representation to characterize the states of an environment, which we refer to as 'semantic tracklets.' We evaluate 'semantic tracklets' on the visual multi-agent particle environment (VMPE) and on the challenging visual multi-agent GFootball environment. 'Semantic tracklets' consistently outperform baselines on VMPE, and achieve a +2.4 higher score difference than baselines on GFootball. Notably, this method is the first to successfully learn a strategy for five players in the GFootball environment using only visual data. For more, please see our project page: https://ioujenliu.github.io/SemanticTracklets
更多查看译文
关键词
visual data,semantic tracklets,visual multiagent reinforcement learning,disentangled representation,object-centric intermediate representation,visual multiagent particle environment,VMPE,MARL,GFootball environment
AI 理解论文
溯源树
样例
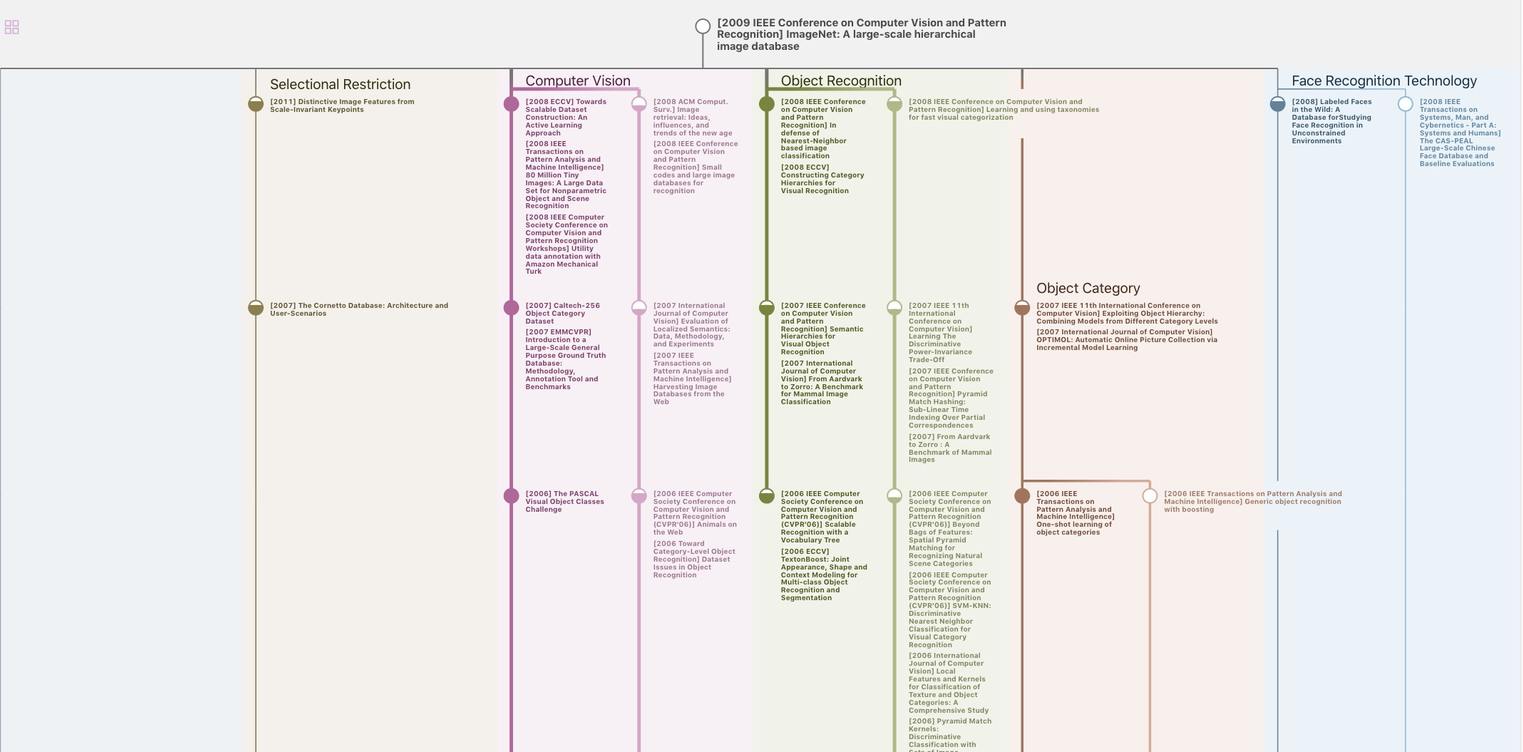
生成溯源树,研究论文发展脉络
Chat Paper
正在生成论文摘要