QA-Driven Zero-shot Slot Filling with Weak Supervision Pretraining.
ACL-IJCNLP 2021: THE 59TH ANNUAL MEETING OF THE ASSOCIATION FOR COMPUTATIONAL LINGUISTICS AND THE 11TH INTERNATIONAL JOINT CONFERENCE ON NATURAL LANGUAGE PROCESSING, VOL 2(2021)
摘要
Slot-filling is an essential component for building task-oriented dialog systems. In this work, we focus on the zero-shot slot-filling problem, where the model needs to predict slots and their values, given utterances from new domains without training on the target domain. Prior methods directly encode slot descriptions to generalize to unseen slot types. However, raw slot descriptions are often ambiguous and do not encode enough semantic information, limiting the models' zero-shot capability. To address this problem, we introduce QA-driven slot filling (QASF), which extracts slot-filler spans from utterances with a span-based QA model. We use a linguistically motivated questioning strategy to turn descriptions into questions, allowing the model to generalize to unseen slot types. Moreover, our QASF model can benefit from weak supervision signals from QA pairs synthetically generated from unlabeled conversations. Our full system substantially outperforms baselines by over 5% on the SNIPS benchmark.
更多查看译文
关键词
Benchmark (computing),Natural language processing,ENCODE,Component (UML),Computer science,Focus (optics),Domain (software engineering),Zero (linguistics),Dialog box,Shot (filmmaking),Artificial intelligence
AI 理解论文
溯源树
样例
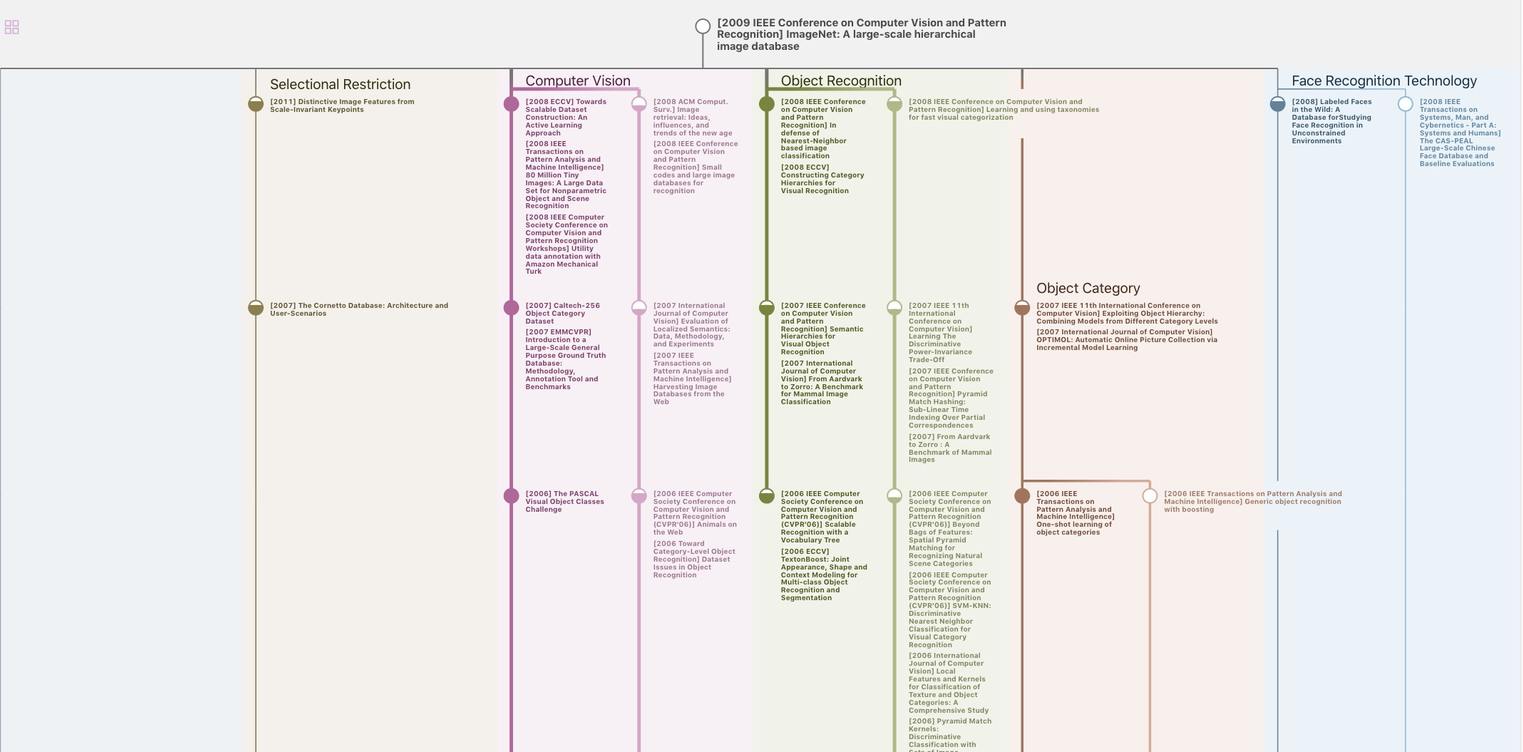
生成溯源树,研究论文发展脉络
Chat Paper
正在生成论文摘要