Zero Knowledge Adversarial Defense Via Iterative Translation Cycle.
ICME(2021)
摘要
Image classification networks based on deep learning are found to be vulnerable to the carefully designed adversarial examples. The existing approaches are still far from solving this problem. In this paper, we propose iterative translation cycle GAN (ITC-GAN) to jointly optimize generators and discriminators, so as to defense against adversarial examples with zero knowledge of adversarial attacks. We train two generators to form an iterative translation cycle which changes deep features of the input images and then reconstructs them. As the cycle of image translation can be conducted iteratively, the noises of adversarial examples are gradually eliminated. We only use clean images to train the whole ITC-GAN, so our method is not coupled to specific attack methods and specific classifiers. We conduct experiments on MNIST, CIFAR10, and ImageNet50. The experimental results demonstrate that ITC-GAN is more robust and flexible than state-of-the-art methods in different adversarial settings.
更多查看译文
关键词
Adversarial system,Zero-knowledge proof,Translation (geometry),Theoretical computer science,Computer science
AI 理解论文
溯源树
样例
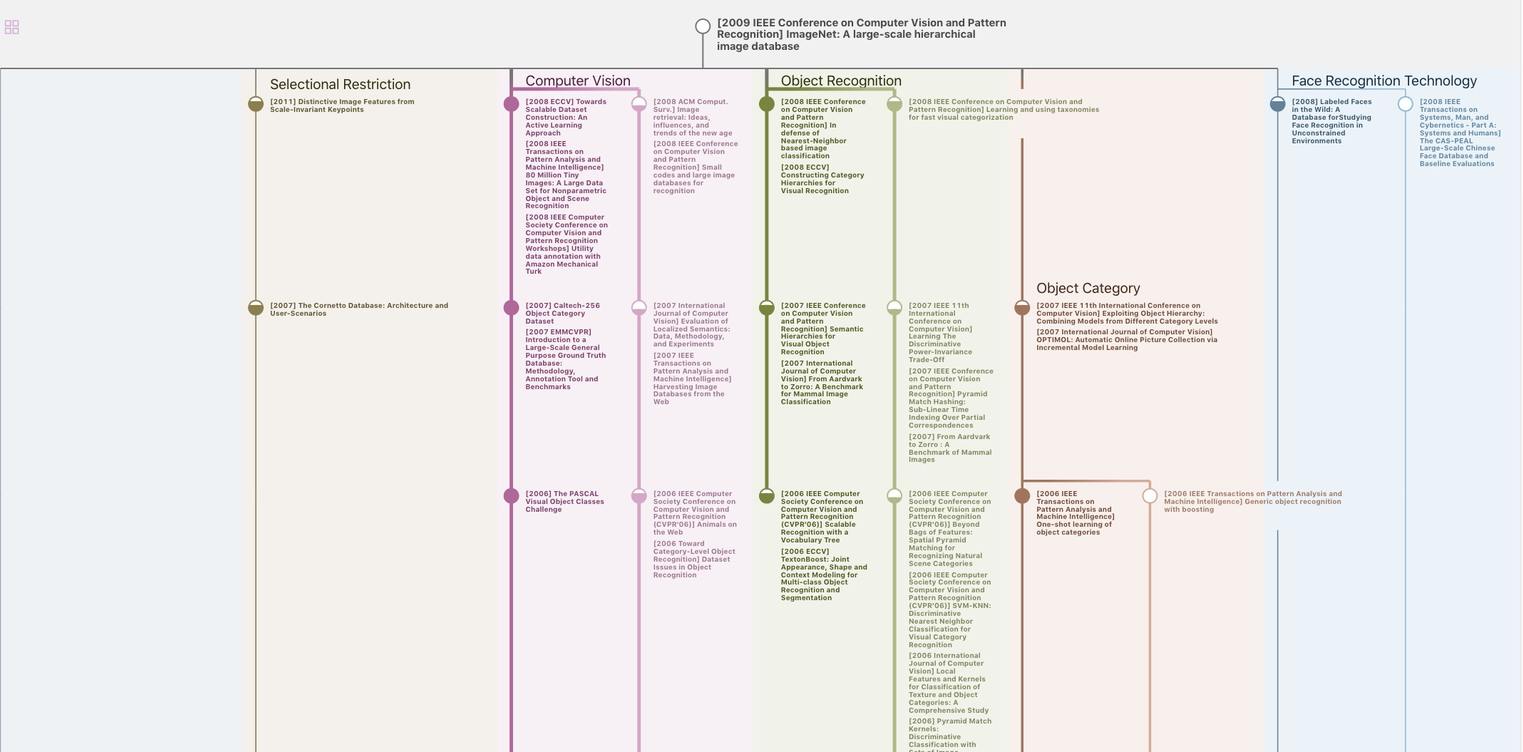
生成溯源树,研究论文发展脉络
Chat Paper
正在生成论文摘要