Debiasing Concept Bottleneck Models with a Causal Analysis Technique
user-5d4bc4a8530c70a9b361c870(2020)
摘要
Concept-based explanation approach is a popular model interpertability tool because it expresses the reasons for a model's predictions in terms of concepts that are meaningful for the domain experts. In this work, we study the problem of the concepts being correlated with confounding information in the features. We propose a new causal prior graph for modeling the impacts of unobserved variables and a method to remove the impact of confounding information and noise using the instrumental variable techniques. We also model the completeness of the concepts set and show that our debiasing method works when the concepts are not complete. Our synthetic and real-world experiments demonstrate the success of our method in removing biases and improving the ranking of the concepts in terms of their contribution to the explanation of the predictions.
更多查看译文
关键词
concept bottleneck models,analysis
AI 理解论文
溯源树
样例
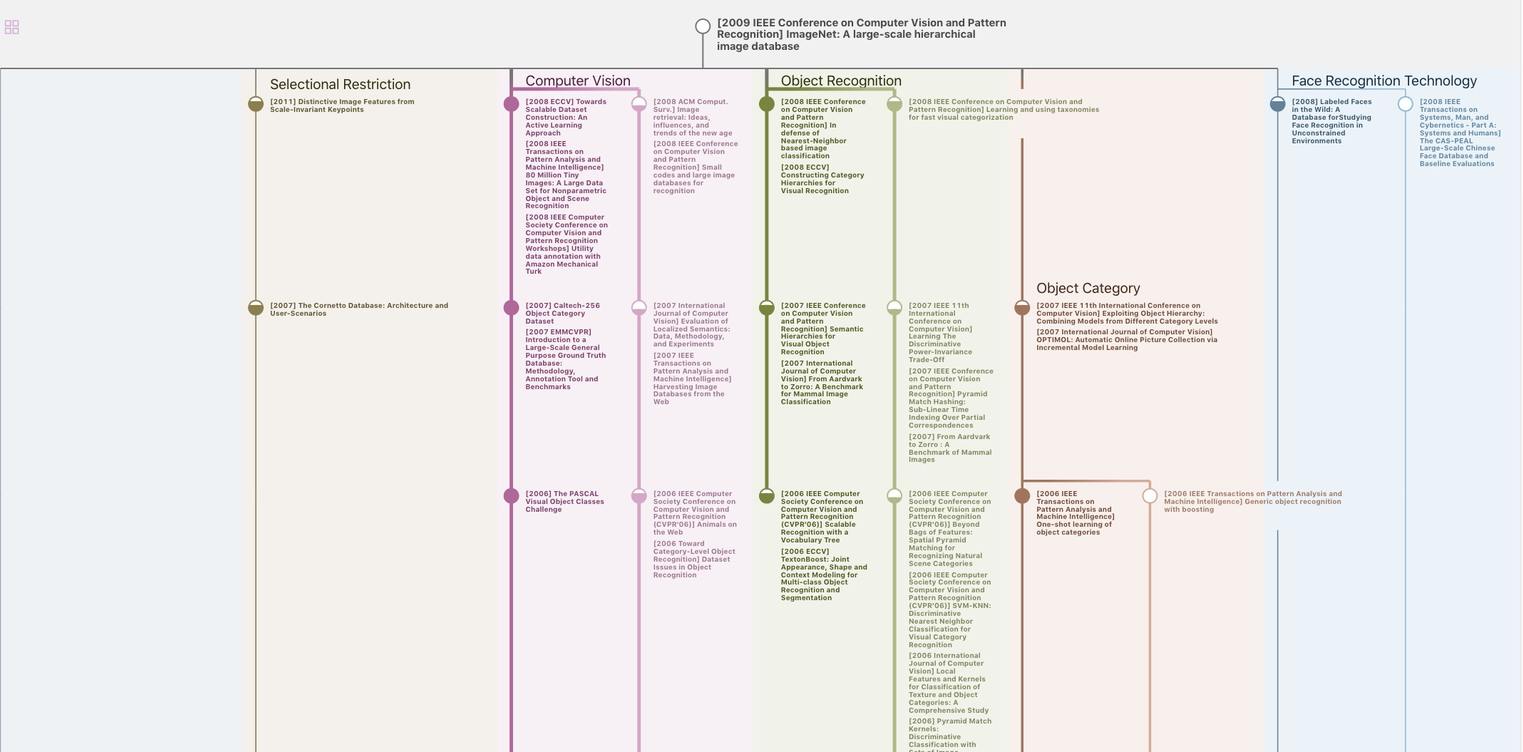
生成溯源树,研究论文发展脉络
Chat Paper
正在生成论文摘要