A note on identification constraints and information criteria in Bayesian latent variable models
Behavior Research Methods(2021)
摘要
It is well known that, in traditional SEM applications, a scale must be set for each latent variable: typically, either the latent variance or a factor loading is fixed to one. While this has no impact on the fit metrics in ML estimation, it can potentially lead to varying Bayesian model comparison metrics due to the use of different prior distributions under each parameterization. This is a problem, because a researcher could artificially improve one’s preferred model simply by changing the identification constraint. Using a single-factor CFA as motivation for study, we first show that Bayesian model comparison metrics can systematically change depending on constraints used. We then study principled methods for setting the scale of the latent variable that stabilize the model comparison metrics. These methods involve (i) the placement of priors on ratios of factor loadings, as opposed to individual loadings; and (ii) use of effect coding. We illustrate the methods via simulation and application.
更多查看译文
关键词
Bayesian SEM,Structural equation models,Model comparison,Identification,blavaan
AI 理解论文
溯源树
样例
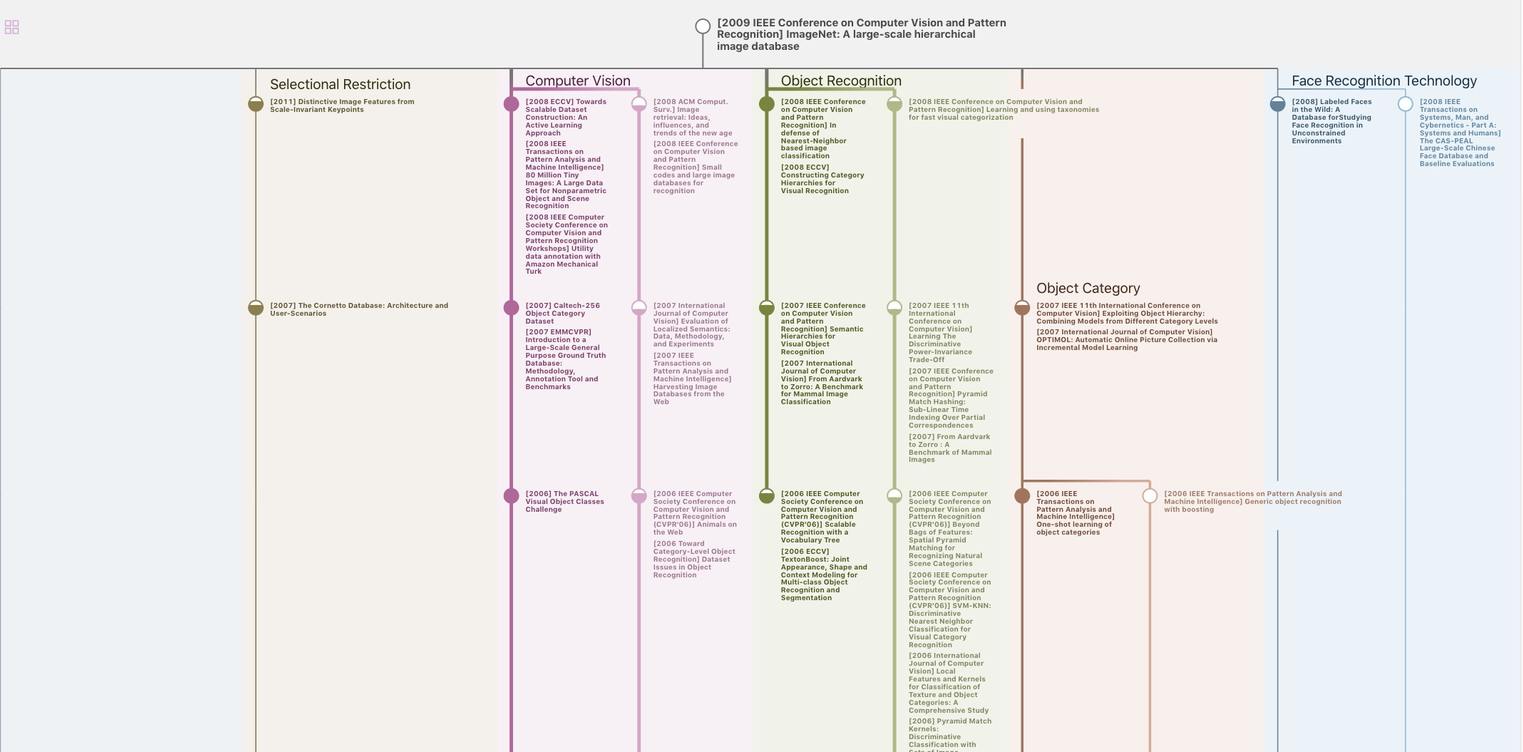
生成溯源树,研究论文发展脉络
Chat Paper
正在生成论文摘要