Classification of Myocardial F-18-FDG PET Uptake Patterns Using Deep Learning
RADIOLOGY-ARTIFICIAL INTELLIGENCE(2021)
摘要
Purpose: To perform automated myocardial segmentation and uptake classification from whole-body fluorine 18 fluorodeoxyglucose (FDG) PET. Materials and Methods: In this retrospective study, consecutive patients who underwent FDG PET imaging for oncologic indications were included (July-August 2018). The left ventricle (LV) on whole-body FDG PET images was manually segmented and classified as showing no myocardial uptake, diffuse uptake, or partial uptake. A total of 609 patients (mean age, 64 years 6 14 [standard deviation]; 309 women) were included and split between training (60%, 365 patients), validation (20%, 122 patients), and testing (20%, 122 patients) datasets. Two sequential neural networks were developed to automatically segment the LV and classify the myocardial uptake pattern using segmentation and classification training data provided by human experts. Linear regression was performed to correlate findings from human experts and deep learning. Classification performance was evaluated using receiver operating characteristic (ROC) analysis. Results: There was moderate agreement of uptake pattern between experts and deep learning (as a fraction of correctly categorized images) with 78% (36 of 46) for no uptake, 71% (34 of 48) for diffuse uptake, and 71% (20 of 28) for partial uptake. There was no bias in LV volume for partial or diffuse uptake categories (P =.56); however, deep learning underestimated LV volumes in the no uptake category. There was good correlation for LV volume (R-2 = 0.35, beta =.71). ROC analysis showed the area under the curve for classifying no uptake and diffuse uptake was high (>= 0.90) but lower for partial uptake (0.77). The feasibility of a myocardial uptake index (MUI) for quantifying the degree of myocardial activity patterns was shown, and there was excellent visual agreement between MUI and uptake patterns. Conclusion: Deep learning was able to segment and classify myocardial uptake patterns on FDG PET images.
更多查看译文
关键词
PET, Heart, Computer Aided Diagnosis, Computer Application-Detection/Diagnosis
AI 理解论文
溯源树
样例
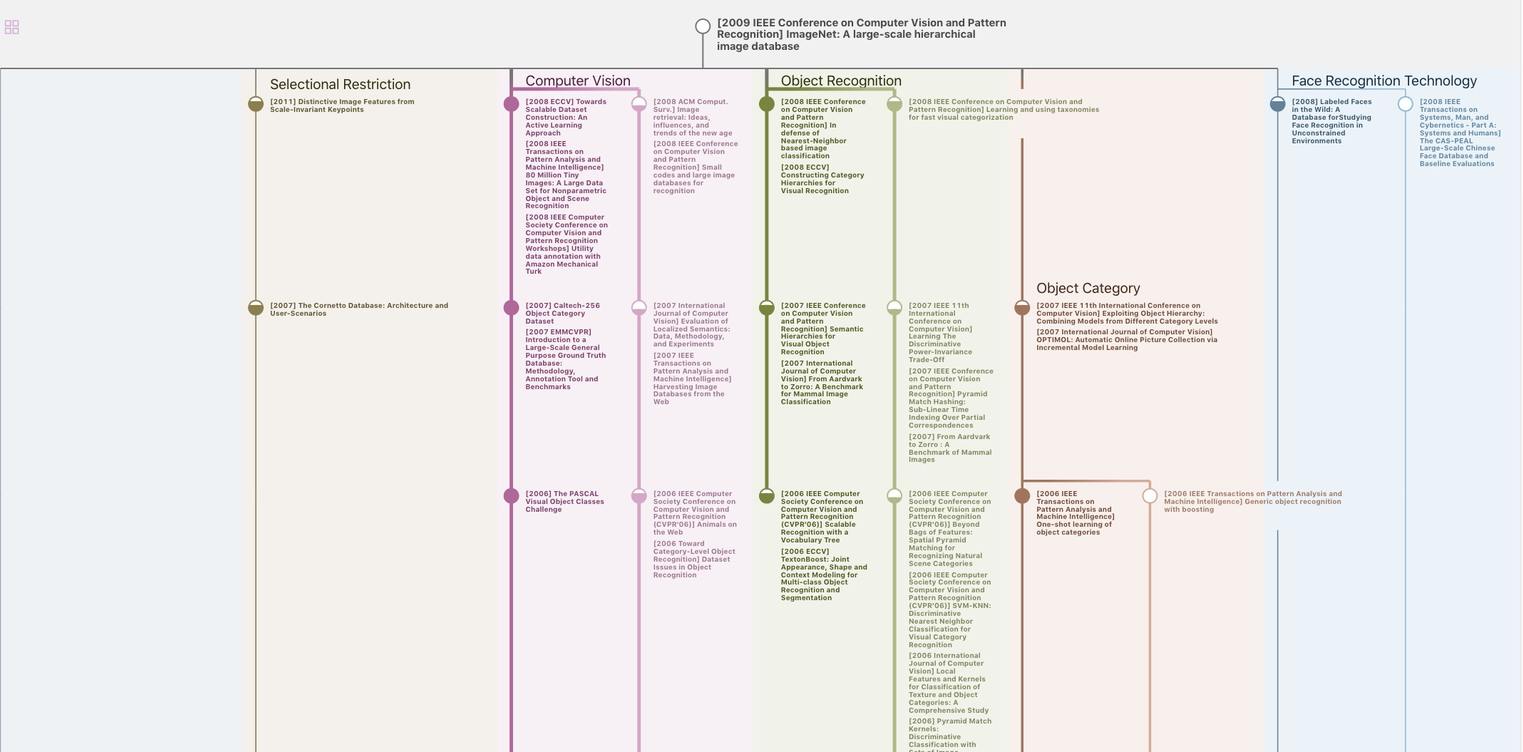
生成溯源树,研究论文发展脉络
Chat Paper
正在生成论文摘要