Diversity collaboratively guided random drift particle swarm optimization
INTERNATIONAL JOURNAL OF MACHINE LEARNING AND CYBERNETICS(2021)
摘要
The random drift particle swarm optimization (RDPSO) algorithm is an effective random search technique inspired by the trajectory analysis of the canonical PSO and the free electron model in metal conductors placed in an external electric field. However, like other PSO variants, the RDPSO algorithm also inevitably encounters premature convergence when solving multimodal problems. To address this issue, this paper proposes a novel diversity collaboratively guided (DCG) strategy for the RDPSO algorithm that enhances the search ability of the algorithm. In this strategy, two kinds of diversity measures are defined and modified in a collaborative manner. Specifically, the whole search process of the RDPSO is divided into three phases based on the changes in the two diversity measures. In each phase, different values are selected for the key parameters of the update equation in the RDPSO to make the particle swarm perform different search modes. Consequently, the improved RDPSO algorithm with the DCG strategy (DCG-RDPSO) can maintain its diversity dynamically at a certain level, and thus can search constantly without stagnation until the search process terminates. The performance evaluation of the proposed algorithm is done on the CEC-2013 benchmark suite, in comparison with several versions of RDPSO, different variants of PSO and several non-PSO evolutionary algorithms. Experimental results show that the proposed DCG strategy can significantly improve the performance and robustness of the RDPSO algorithm for most of the multimodal problems. Further experiments on economic dispatch problems also verify the effectiveness of the DCG strategy.
更多查看译文
关键词
Diversity guided strategy, Random drift particle swarm optimization algorithm, Multimodal optimization problems, Economic dispatch problems
AI 理解论文
溯源树
样例
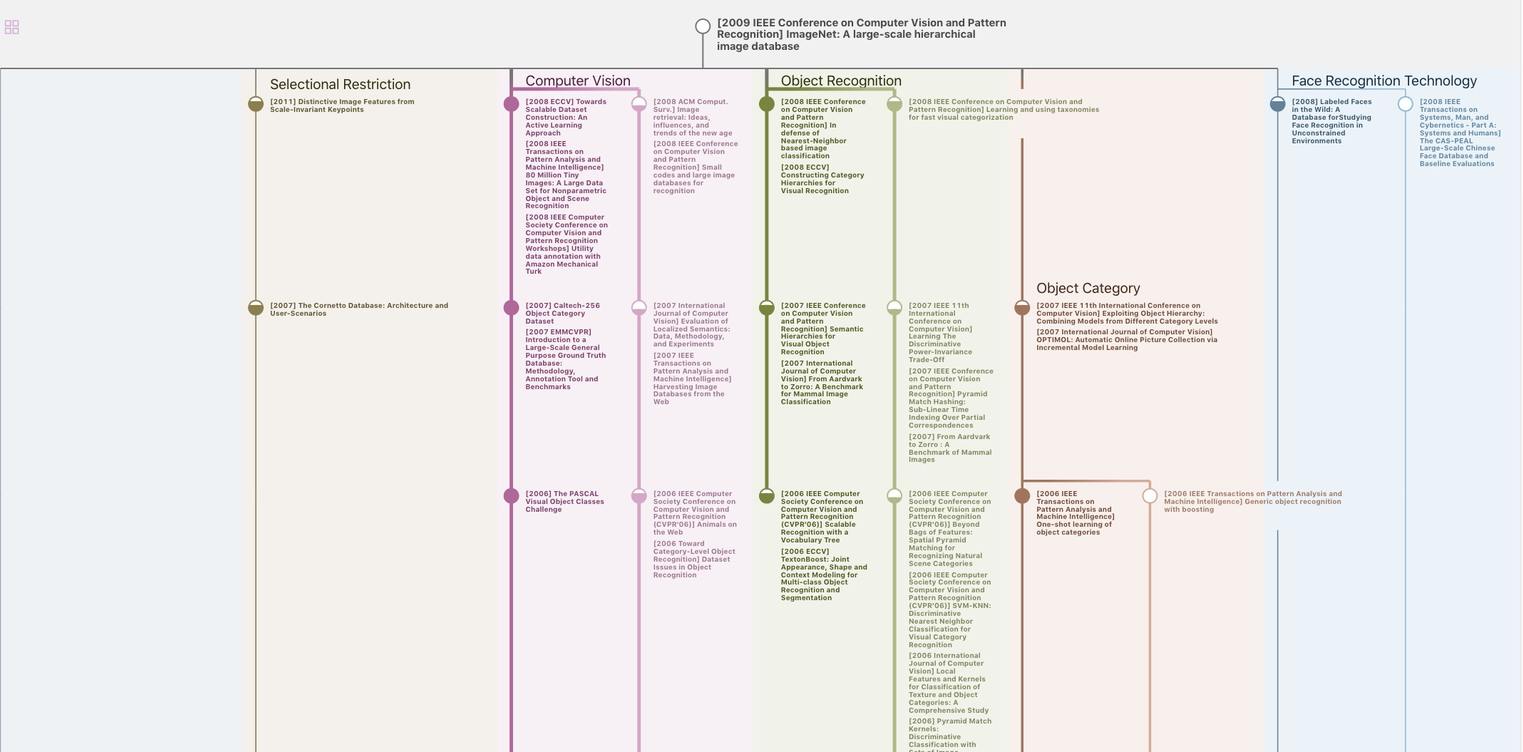
生成溯源树,研究论文发展脉络
Chat Paper
正在生成论文摘要