Reinforcement Learning-Based Optimal Battery Control Under Cycle-Based Degradation Cost
IEEE Transactions on Smart Grid(2022)
摘要
Battery energy storage systems are providing increasing level of benefits to power grid operations by decreasing the resource uncertainty and supporting frequency regulation. Thus, it is crucial to obtain the optimal policy for utility-level battery to efficiently provide these grid-services while accounting for its degradation cost. To solve the optimal battery control (OBC) problem using the powerful reinforcement learning (RL) algorithms, this paper aims to develop a new representation of the cycle-based battery degradation model according to the rainflow algorithm. As the latter depends on the full trajectory, existing work has to rely on linearized approximation for converting it into instantaneous terms for the Markov Decision Process (MDP) based formulation. We propose a new MDP form by introducing additional state variables to keep track of past switching points for determining the cycle depth. The proposed degradation model allows to adopt the powerful deep Q-Network (DQN) based RL algorithm to efficiently search for the OBC policy. Numerical tests using real market data have demonstrated the performance improvements of the proposed cycle-based degradation model in enhancing the battery operations while mitigating its degradation, as compared to earlier work using the linearized approximation.
更多查看译文
关键词
Energy storage,reinforcement learning,battery degradation,rainflow algorithm,deep Q-networks (DQN)
AI 理解论文
溯源树
样例
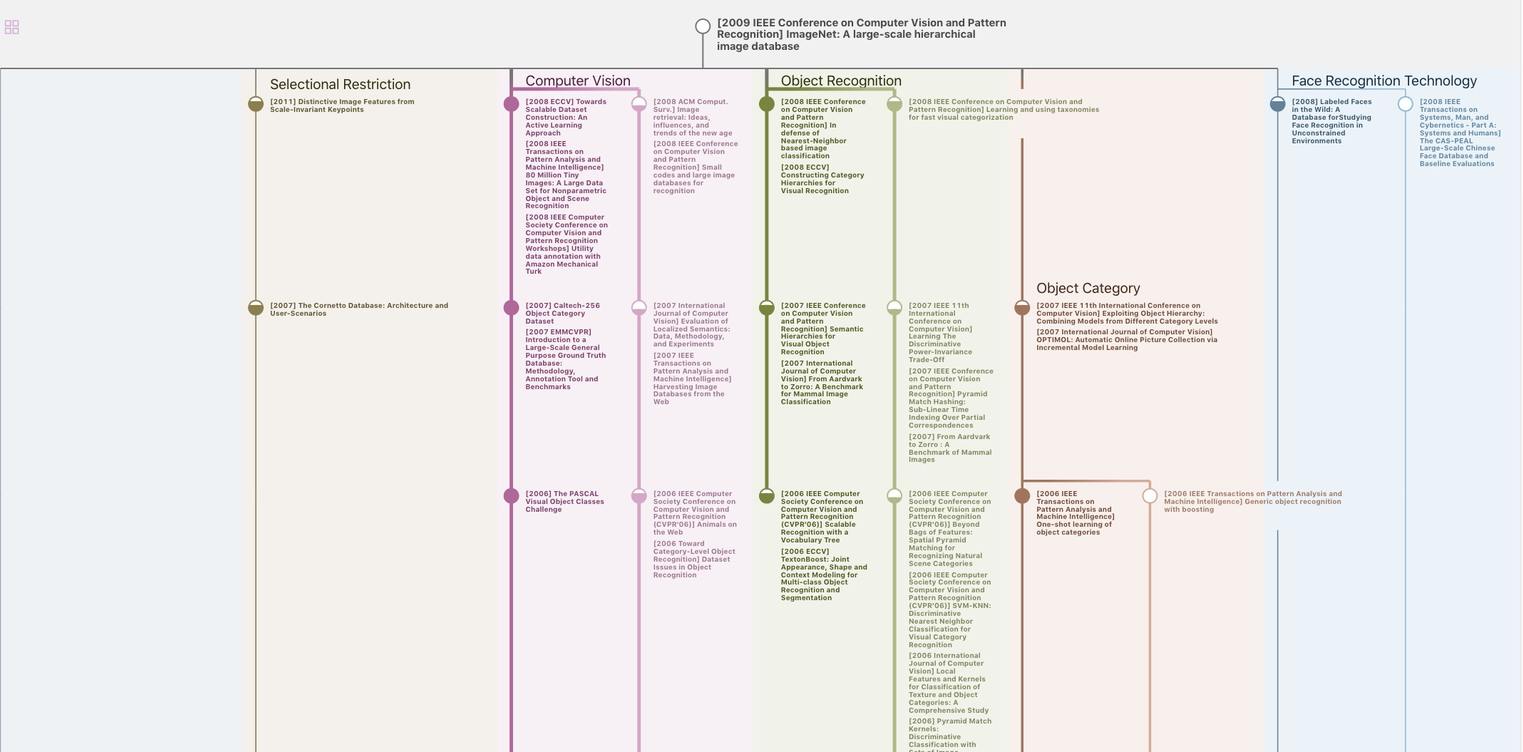
生成溯源树,研究论文发展脉络
Chat Paper
正在生成论文摘要