Development of convolutional neural networks for recognition of tenogenic differentiation based on cellular morphology
Periodicals(2021)
摘要
AbstractHighlights •Convolutional neural network (CNN) models recognize the differences in cellular morphology of bone marrow-derived mesenchymal stem cells (BMSCs).•Application of image enhancement and data augmentation is an effective way to improve network performance.•CNNs can be introduced as a non-invasive methodology assessing stem cell differentiation in the culturing state. Graphical abstractDisplay OmittedAbstractBackground and objective: The use of automated systems for image recognition is highly preferred for regenerative medicine applications to evaluate stem cell differentiation early in the culturing state with non-invasive methodologies instead of invasive counterparts. Bone marrow-derived mesenchymal stem cells (BMSCs) are able to differentiate into desired cell phenotypes, and thereby promise a proper cell source for tendon regeneration. The therapeutic success of stem cell therapy requires cellular characterization prior to the implantation of cells. The foremost problem is that traditional characterization techniques require cellular material which would be more useful for cell therapy, complex laboratory procedures, and human expertise. Convolutional neural networks (CNNs), a class of deep neural networks, have recently made great improvements in image-based classifications, recognition, and detection tasks. We, therefore, aim to develop a potential CNN model in order to recognize differentiated stem cells by learning features directly from image data of unlabelled cells.Methods: The differentiation of bone marrow mesenchymal stem cells (BMSCs) into tenocytes was induced with the treatment of bone morphogenetic protein-12 (BMP-12). Following the treatment and incubation step, the phase-contrast images of cells were obtained and immunofluorescence staining has been applied to characterize the differentiated state of BMSCs. CNN models were developed and trained with the phase-contrast cell images. The comparison of CNN models was performed with respect to prediction performance and training time. Moreover, we have evaluated the effect of image enhancement method, data augmentation, and fine-tuning training strategy to increase classification accuracy of CNN models. The best model was integrated into a mobile application.Results: All the CNN models can fit the biological data extracted from immunofluorescence characterization. CNN models enable the cell classification with satisfactory accuracies. The best result in terms of accuracy and training time is achieved by the model proposed based on Inception-ResNet V2 trained from scratch using image enhancement and data augmentation strategies (96.80%, 434.55 sec).Conclusion: Our study reveals that the CNN models show good performance by identifying stem cell differentiation. Importantly this technique provides a faster and real-time tool in comparison to traditional methods enabling the adjustment of culture conditions during cultivation to improve the yield of therapeutic stem cells.
更多查看译文
关键词
Convolutional neural network, Image recognition, Stem cell differentiation
AI 理解论文
溯源树
样例
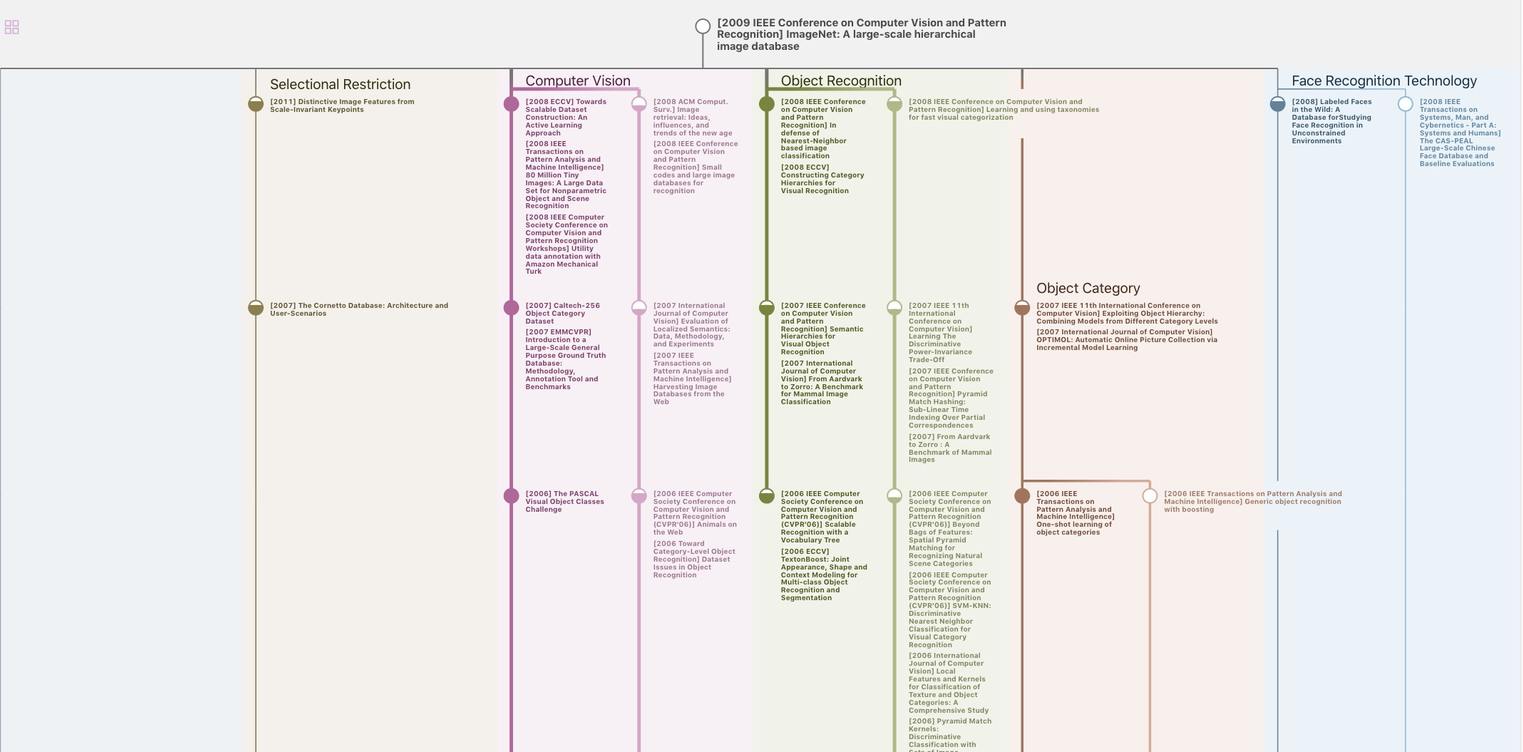
生成溯源树,研究论文发展脉络
Chat Paper
正在生成论文摘要