Improved Prediction Of Drug-Target Interactions Based On Ensemble Learning With Fuzzy Local Ternary Pattern
FRONTIERS IN BIOSCIENCE-LANDMARK(2021)
摘要
Introduction: The prediction of interacting drug-target pairs plays an essential role in the field of drug repurposing, and drug discovery. Although biotechnology and chemical technology have made extraordinary progress, the process of dose-response experiments and clinical trials is still extremely complex, laborious, and costly. As a result, a robust computer-aided model is of an urgent need to predict drug-target interactions (DTIs). Methods: In this paper, we report a novel computational approach combining fuzzy local ternary pattern (FLIP), Position-Specific Scoring Matrix (PSSM), and rotation forest (RF) to identify DTIs. More specially, the target primary sequence is first numerically characterized into PSSM which records the biological evolution information. Afterward, the FLIP method is applied in extracting the highly representative descriptors of PSSM, and the combinations of FLIP descriptors and drug molecular fingerprints are regarded as the complete features of drug-target pairs. Results: Finally, the entire features are fed into rotation forests for inferring potential DTIs. The experiments of 5-fold cross-validation (CV) achieve mean accuracies of 89.08%, 86.14%, 82.41%, and 78.40% on Enzyme, Ion Channel, GPCRs, and Nuclear Receptor datasets. Discussion: For further validating the model performance, we performed experiments with the state-of-art support vector machine (SVM) and light gradient boosting machine (LGBM). The experimental results indicate the superiorities of the proposed model in effectively and reliably detect potential DTIs. There is an anticipation that the proposed model can establish a feasible and convenient tool to identify high-throughput identification of DTIs.
更多查看译文
关键词
Drug-target interactions, Fuzzy local ternary pattern, Drug molecular fingerprints, Rotation forest
AI 理解论文
溯源树
样例
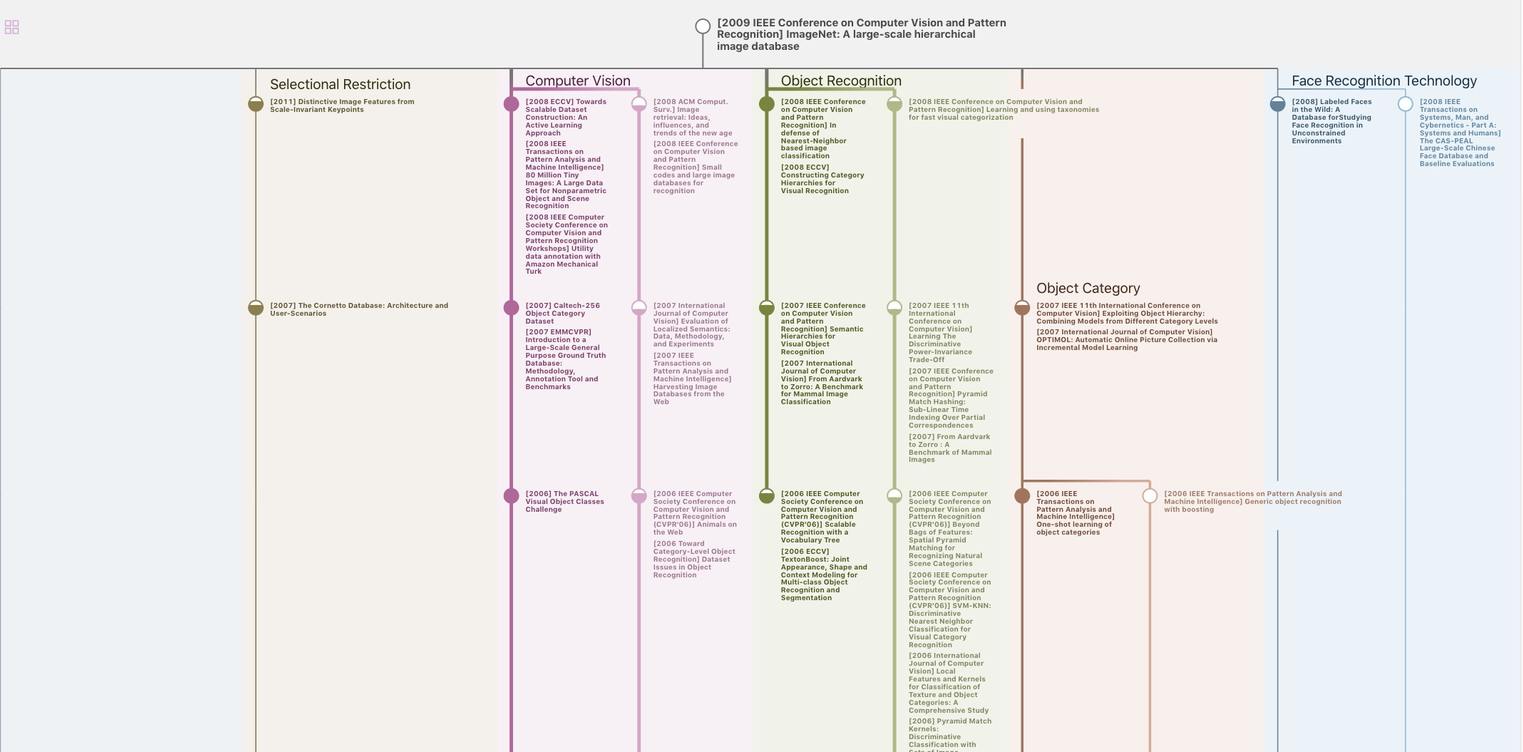
生成溯源树,研究论文发展脉络
Chat Paper
正在生成论文摘要